Network-Agnostic Knowledge Transfer for Medical Image Segmentation
ArXiv(2021)
摘要
Conventional transfer learning leverages weights of pre-trained networks, but mandates the need for similar neural architectures. Alternatively, knowledge distillation can transfer knowledge between heterogeneous networks but often requires access to the original training data or additional generative networks. Knowledge transfer between networks can be improved by being agnostic to the choice of network architecture and reducing the dependence on original training data. We propose a knowledge transfer approach from a teacher to a student network wherein we train the student on an independent transferal dataset, whose annotations are generated by the teacher. Experiments were conducted on five state-of-the-art networks for semantic segmentation and seven datasets across three imaging modalities. We studied knowledge transfer from a single teacher, combination of knowledge transfer and fine-tuning, and knowledge transfer from multiple teachers. The student model with a single teacher achieved similar performance as the teacher; and the student model with multiple teachers achieved better performance than the teachers. The salient features of our algorithm include: 1)no need for original training data or generative networks, 2) knowledge transfer between different architectures, 3) ease of implementation for downstream tasks by using the downstream task dataset as the transferal dataset, 4) knowledge transfer of an ensemble of models, trained independently, into one student model. Extensive experiments demonstrate that the proposed algorithm is effective for knowledge transfer and easily tunable.
更多查看译文
关键词
segmentation,knowledge,transfer,network-agnostic
AI 理解论文
溯源树
样例
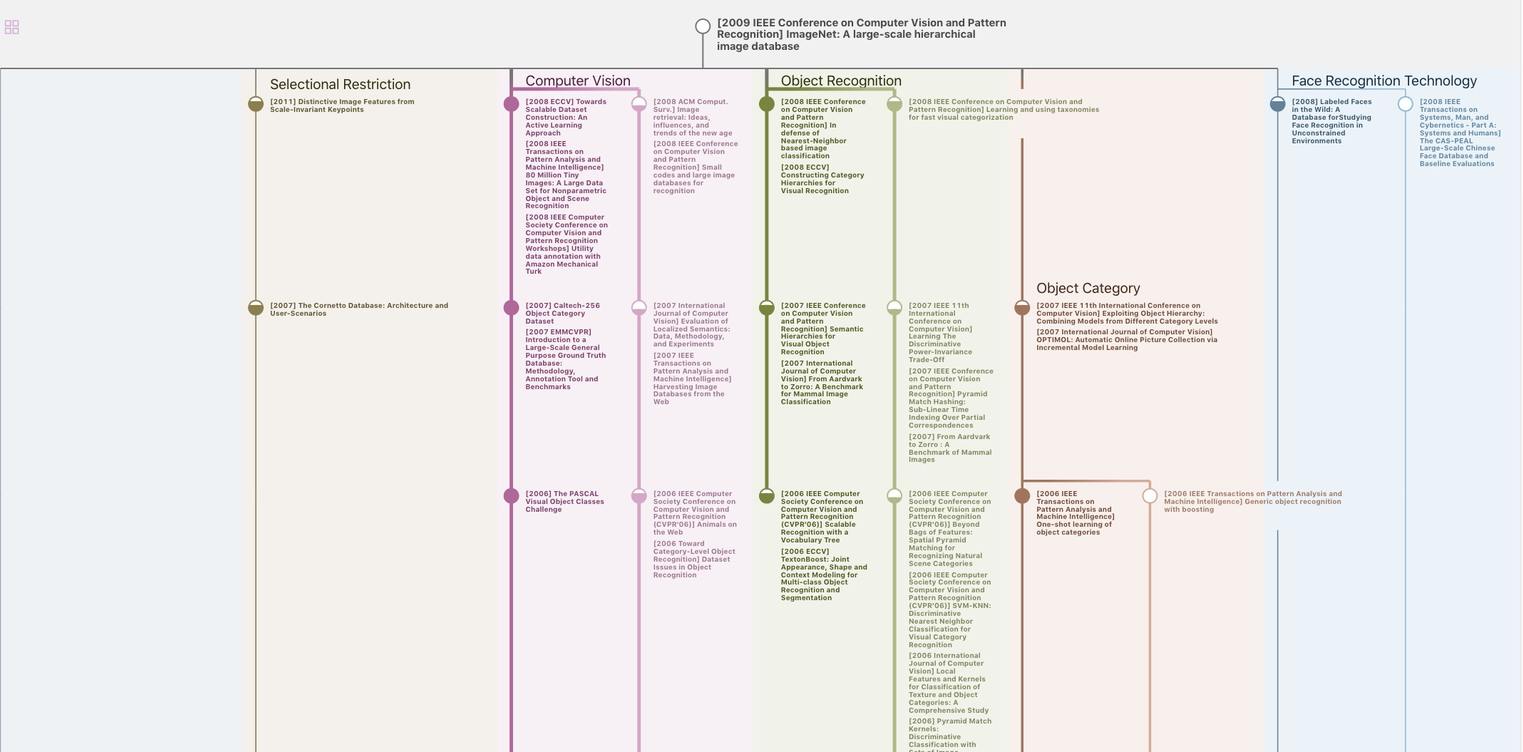
生成溯源树,研究论文发展脉络
Chat Paper
正在生成论文摘要