Land Cover Change Detection With Vhr Satellite Imagery Based On Multi-Scale Slic-Cnn And Scae Features
IEEE ACCESS(2020)
摘要
Change detection with very high resolution (VHR) satellite images is of great application values when evaluating and monitoring land use changes. However, intrinsic complexity of satellite images will introduce more difficulties to change detection tasks. In this study, a new change detection method is proposed by combining multi-scale simple linear iterative clustering-convolutional neural network (SLIC-CNN) with stacked convolutional auto-encoder (SCAE) features to improve change detection capabilities with VHR satellite images. First, the multi-scale SLIC-based image segmentation is performed on multi-temporal images to generate segment objects while keeping their edge information as much as possible. Second, the convolutional layers in a CNN architecture are used to generate change map, then, an SCAE feature-based classification procedure is performed to generate "from-to" change information. Finally, a Bayesian information criterion is used to optimize the results of change detection. In this study, the experiments carried out reveal that the multi-scale SLIC image segmentation algorithm affects the integrity of change regions; CNN features have an effect on the consistency of change regions; and SCAE features influence the performance of support vector machine (SVM) classifiers. And, features extracted from the architectures enhance the ability of information extraction from ground objects. Comparison results also show the superiority to other change detection methods.
更多查看译文
关键词
Image segmentation,Feature extraction,Satellites,Change detection algorithms,Spatial resolution,Computer architecture,Detection algorithms,Change detection,image segmentation,multi-scale simple linear iterative clustering-convolutional neural network (SLIC-CNN),stacked convolutional auto-encoder (SCAE),VHR satellite imagery
AI 理解论文
溯源树
样例
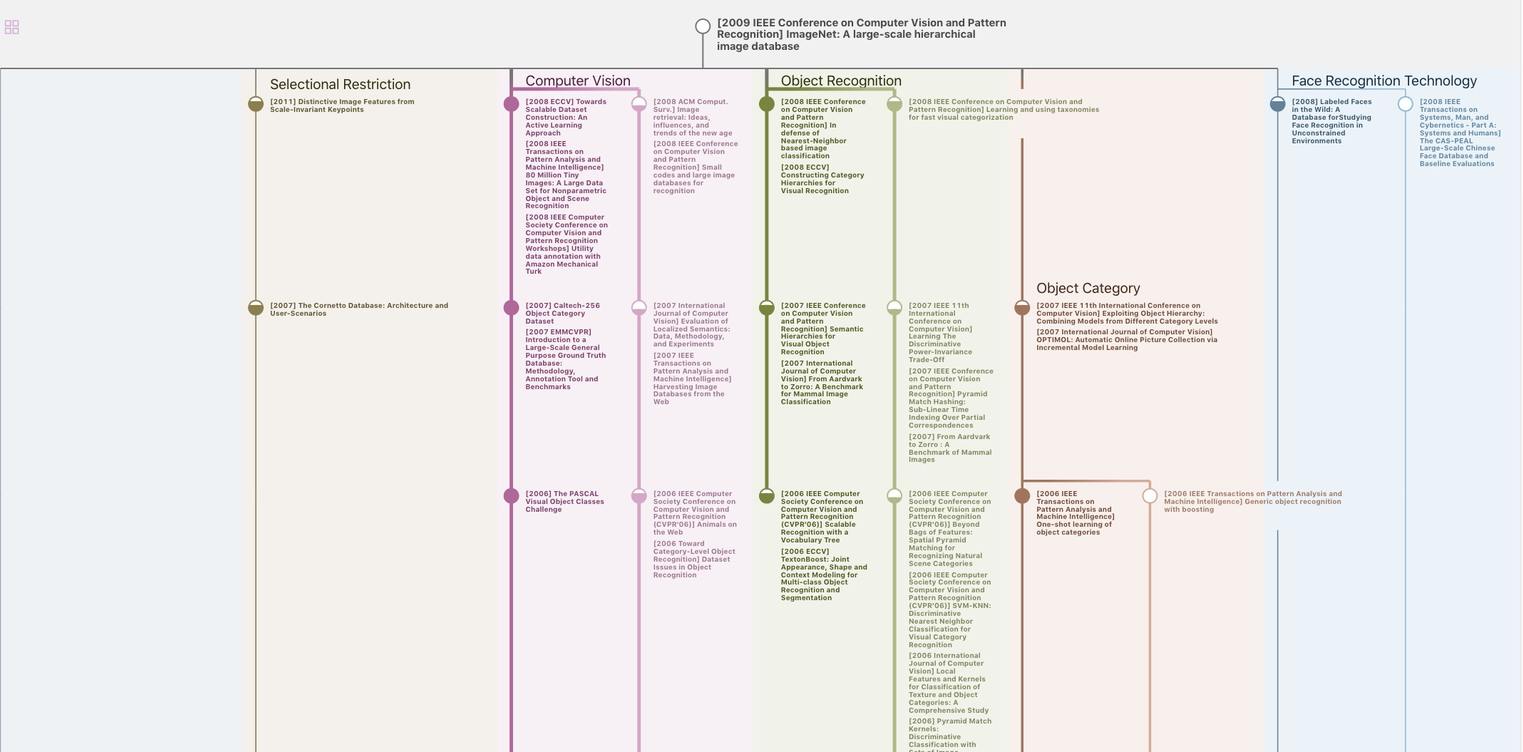
生成溯源树,研究论文发展脉络
Chat Paper
正在生成论文摘要