Automatic sleep staging with a single-channel EEG based on ensemble empirical mode decomposition
Physica A(2021)
摘要
Objective evaluation of sleep is crucial to recognize sleep disorders and take effective interventions accordingly. Due to the shortcomings of the conventional polysomnographic acquisition, an automatic sleep scoring method based on a single-channel electroencephalogram (EEG) is vital in sleep medicine practice. In this study, we proposed a data-driven and robust automatic sleep staging scheme based on a single-channel EEG. With the decomposition of the EEG epochs using Ensemble Empirical Mode Decomposition (EEMD), we extracted various features using statistical, time-domain and nonlinear dynamics characteristics from not only the original EEG signal but also the decomposed intrinsic mode functions (IMFs). A classification model with the eXtreme Gradient Boosting (XGBoost) algorithm was trained and tested using 5-fold cross-validation on three different databases, i.e., the Sleep-EDF database available at Physionet, the DREAMS Subjects database and the database of Sleep Heart Health Study (SHHS). The results demonstrated that the analysis of IMFs derived from EEMD could provide substantial supplement to the classification of EEG sleep stages. In the tasks of 4-class and 5-class sleep staging, the proposed method achieved an accuracy of 93.1% and 91.9% for the Sleep-EDF database, 86.4% and 83.4% for the DREAMS Subjects database, and 87.5% and 85.8% for the SHHS database, respectively. Furthermore, our observation that prefrontal EEG derivations should be optimal choices might evoke promising application of wearable EEG devices on sleep monitoring because EEG signals can be easily obtained using dry electrodes on the forehead. Additionally, the proposed method is computationally efficient and should be valuable in real-time sleep-scoring. (C) 2020 Elsevier B.V. All rights reserved.
更多查看译文
关键词
Electroencephalogram,Ensemble empirical mode decomposition,Machine learning,Boosting,Sleep stage classification
AI 理解论文
溯源树
样例
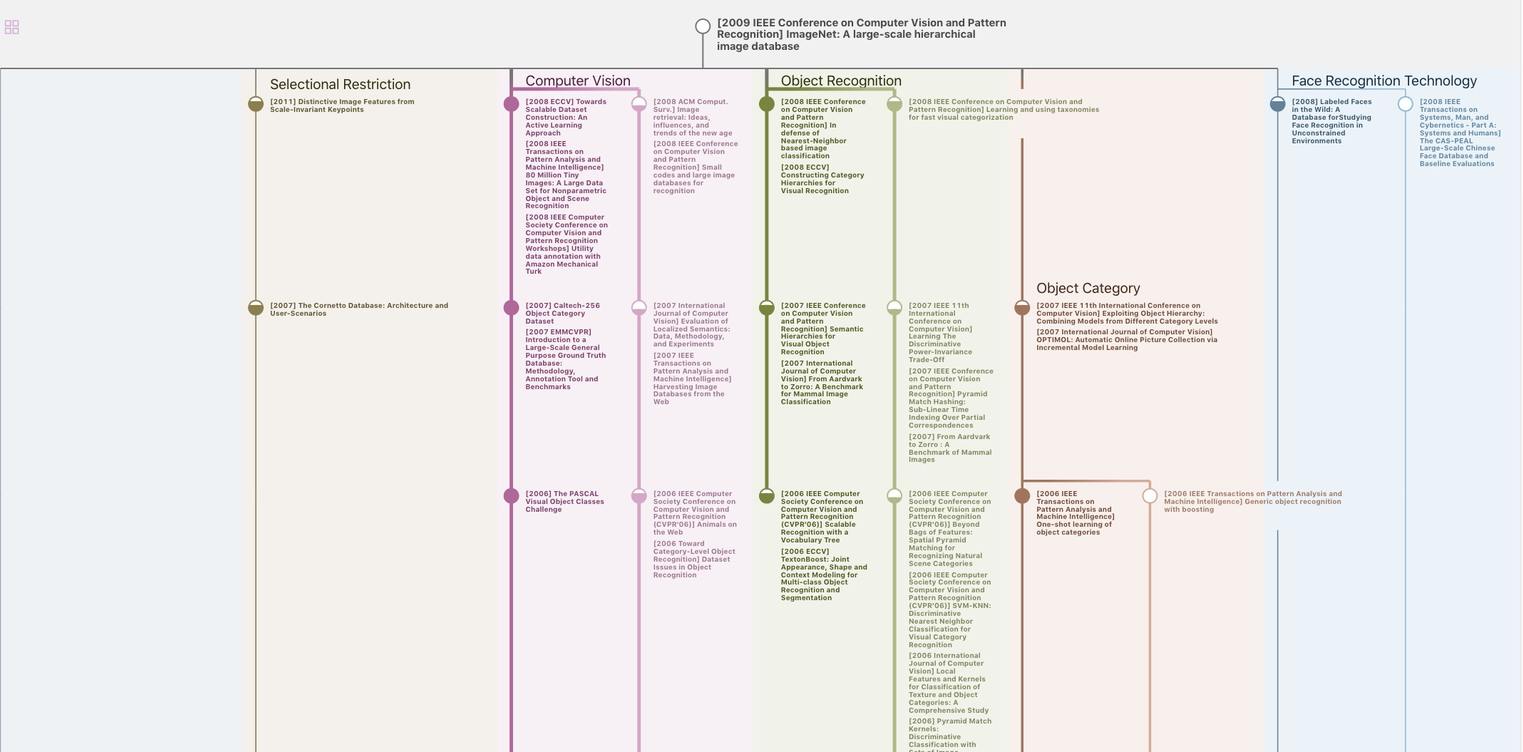
生成溯源树,研究论文发展脉络
Chat Paper
正在生成论文摘要