Adaptive Stochastic Model Predictive Control of Linear Systems Using Gaussian Process Regression
IET control theory & applications(2020)
摘要
This paper presents a stochastic model predictive control method for linear time-invariant systems subject to state-dependent additive uncertainties modelled by Gaussian process (GP). The new method is developed by re-building the tube-based model predictive control framework with chance constraints via adaptive constraint tightening. In particular, the tightened constraint set is constructed by forecasting the confidence region of uncertainty. Utilising this adaptive strategy, the Gaussian process based stochastic model predictive control (GP-SMPC) algorithm is designed by applying the adaptive tightened constraints in all prediction horizons. To reduce the computation load, the one-step GP-SMPC algorithm is further developed by imposing the tightened constraints only to the first-step nominal state and the worst-case constraints to the remaining steps. Under the assumption that the state-dependent uncertainties are bounded, the recursive feasibility of the designed optimisation problem is ensured, and the closed-loop system stability is guaranteed. The effectiveness and advantage over existing methods are verified via simulation and comparison studies.
更多查看译文
关键词
Optimisation techniques,Other topics in statistics,Linear control systems,Stability in control theory,Optimal control,Self-adjusting control systems
AI 理解论文
溯源树
样例
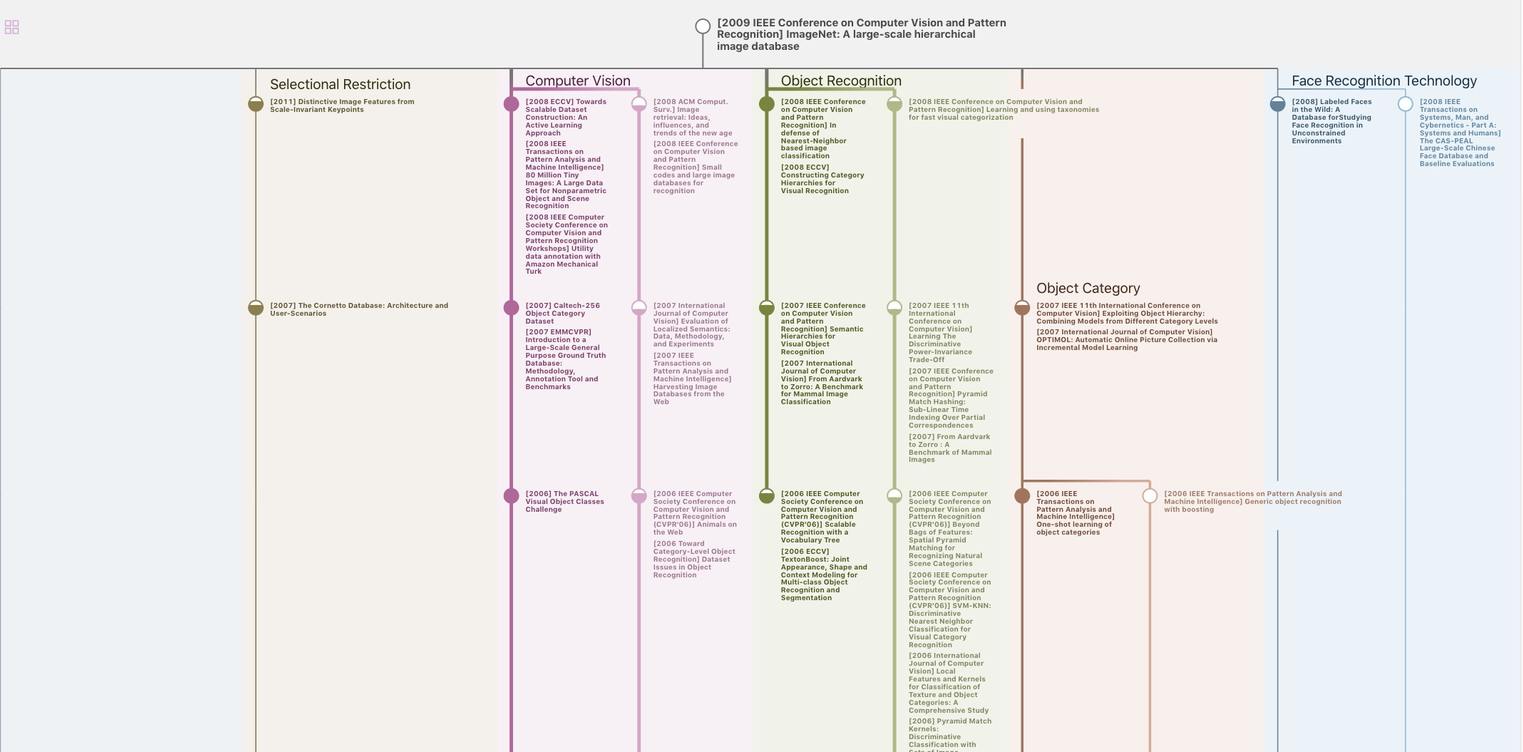
生成溯源树,研究论文发展脉络
Chat Paper
正在生成论文摘要