Study of Spatial-Spectral Feature Extraction Frameworks With 3-D Convolutional Neural Network for Robust Hyperspectral Imagery Classification
IEEE JOURNAL OF SELECTED TOPICS IN APPLIED EARTH OBSERVATIONS AND REMOTE SENSING(2021)
摘要
Advances in hyperspectral remote sensing have instigated multitude of applications for better understanding of our planet through remote data acquisition and observation of natural phenomena such as weather monitoring and prediction to include tornado, wild fires, global warming, etc. For this, data analysis methods that exploit the rich spectral and spatial information in hyperspectral data are often employed to gain insights about the natural phenomenon. This work presents a new deep learning based hyperspectral data analysis framework, which efficiently utilizes both spatial and spectral information present in the data to achieve superior classification performance. Gabor filtering is used for spatial feature extraction in conjunction with sparse random projections for spectral feature extraction and dimensionality reduction. Finally, supervised classification using a 3-D convolutional neural network was employed to perform a volumetric hyperspectral data analysis. Experimental results reveal that the proposed spatial-spectral hyperspectral data analysis frameworks outperform the conventional 2-D convolution neural network-based spectral-spatial feature extraction techniques.
更多查看译文
关键词
Hyperspectral imaging,Feature extraction,Data analysis,Principal component analysis,Two dimensional displays,Data mining,Three-dimensional displays,3-D convolutional neural network (3-D CNN),deep learning,dimensionality reduction (DR),feature extraction,Gabor filtering,Gaussian filtering,hyperspectral classification,principal component analysis (PCA),sparse random projection (RP),support vector machine (SVM)
AI 理解论文
溯源树
样例
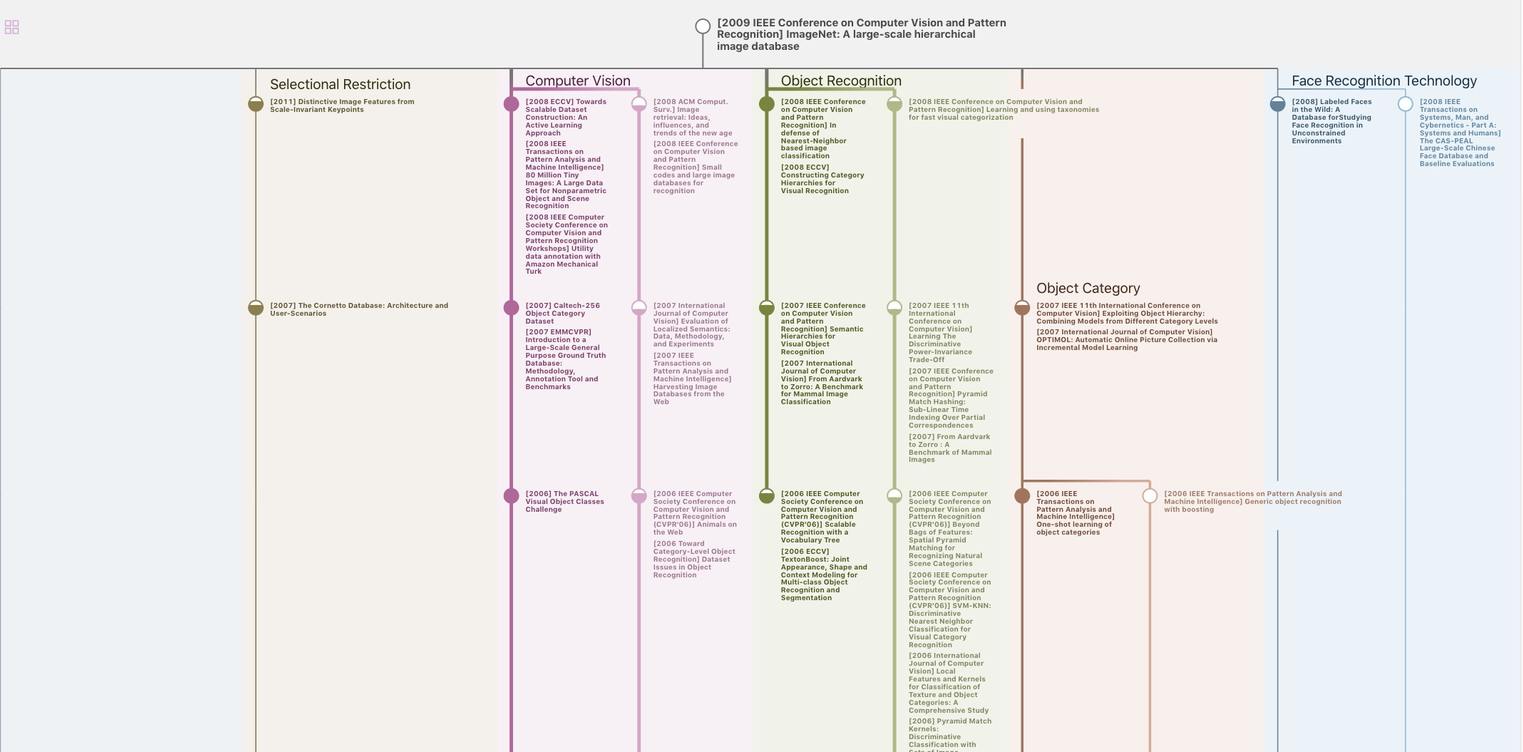
生成溯源树,研究论文发展脉络
Chat Paper
正在生成论文摘要