A Deep Learning Approach To Test The Small-Scale Galaxy Morphology And Its Relationship With Star Formation Activity In Hydrodynamical Simulations
MONTHLY NOTICES OF THE ROYAL ASTRONOMICAL SOCIETY(2021)
摘要
Hydrodynamical simulations of galaxy formation and evolution attempt to fully model the physics that shapes galaxies. The agreement between the morphology of simulated and real galaxies, and the way the morphological types are distributed across galaxy scaling relations are important probes of our knowledge of galaxy formation physics. Here, we propose an unsupervised deep learning approach to perform a stringent test of the fine morphological structure of galaxies coming from the Illustris and IllustrisTNG (TNG100 and TNG50) simulations against observations from a subsample of the Sloan Digital Sky Survey. Our framework is based on PixeICNN, an autoregressive model for image generation with an explicit likelihood. We adopt a strategy that combines the output of two PixeICNN networks in a metric that isolates the small-scale morphological details of galaxies from the sky background. We are able to quantitatively identify the improvements of Illustris'ING, particularly in the high-resolution TNG50 run, over the original Illustris. However, we find that the fine details of galaxy structure are still different between observed and simulated galaxies. This difference is mostly driven by small, more spheroidal, and quenched galaxies that are globally less accurate regardless of resolution and which have experienced little improvement between the three simulations explored. We speculate that this disagreement, that is less severe for quenched discy galaxies, may stem !tom a still too coarse numerical resolution, which struggles to properly capture the inner, dense regions of quenched spheroidal galaxies.
更多查看译文
关键词
methods: miscellaneous, methods: numerical, galaxies: evolution, galaxies: fundamental parameters, galaxies: star formation, galaxies: structure
AI 理解论文
溯源树
样例
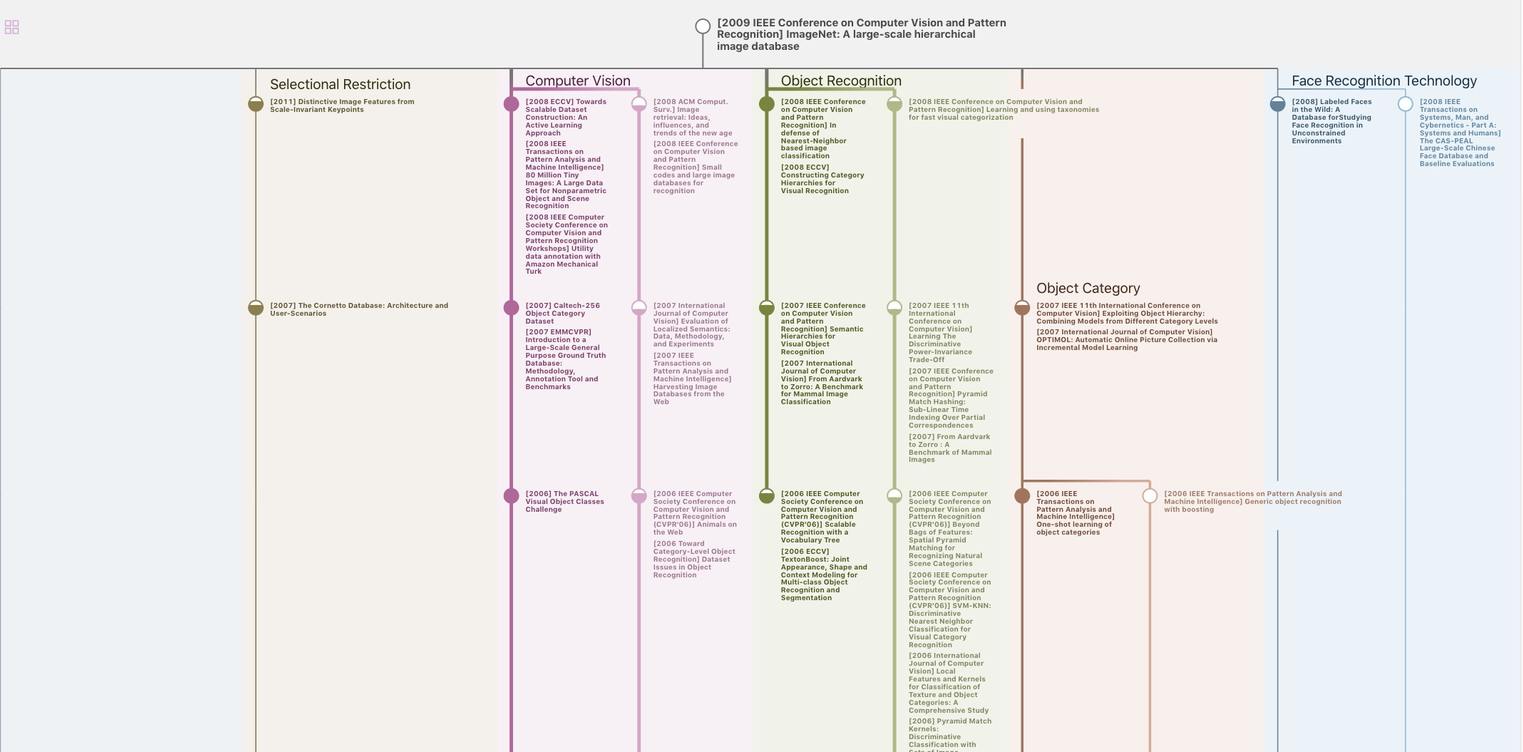
生成溯源树,研究论文发展脉络
Chat Paper
正在生成论文摘要