Uncertainty In Bayesian Deep Label Distribution Learning
APPLIED SOFT COMPUTING(2021)
摘要
Though widely used, most deep convolutional neural networks fail to capture prediction uncertainty, which can be crucial in scenarios such as automotive applications and disease diagnosis. Aleatoric and epistemic uncertainties have been proposed in the Bayesian deep learning framework for regression and classification, which require training images with unambiguous labels for success. Some situations do not have precise labels by nature, such as age estimation or lesion contour annotation by different physicians in the real world. Label distribution learning (LDL) has been proposed to account for the label ambiguity. However, uncertainty estimation has not been studied for LDL. This study presents a Bayesian deep label distribution learning (BLDL) to obtain the uncertainties of LDL tasks. We define semantic uncertainty to account for the incompleteness of data acquisition and inaccurate data labeling and unify the three types of uncertainties' mathematical expressions for classification and LDL. We further demonstrate that applying the three types of uncertainties in the loss functions significantly improves model performance, and using semantic uncertainty as a criterion to select the training samples substantially promotes active learning. (C) 2020 The Authors. Published by Elsevier B.V.
更多查看译文
关键词
Bayesian deep label distribution learning, Semantic uncertainty, Classification, Active learning
AI 理解论文
溯源树
样例
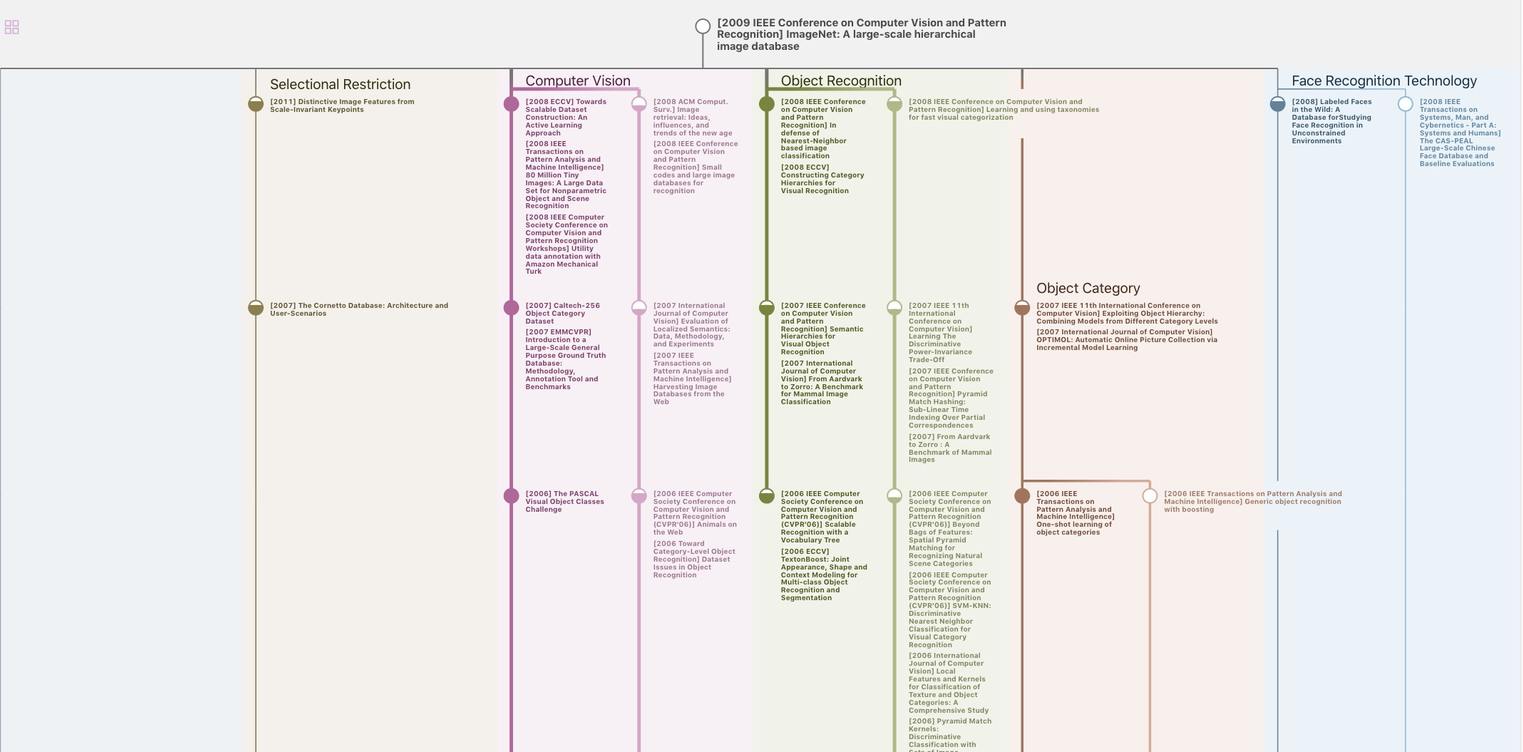
生成溯源树,研究论文发展脉络
Chat Paper
正在生成论文摘要