Unsupervised Anomaly Detection and Localisation with Multi-scale Interpolated Gaussian Descriptors
arxiv(2021)
摘要
Current unsupervised anomaly detection and localisation systems are commonly formulated as one-class classifiers that depend on an effective estimation of the distribution of normal images and robust criteria to identify anomalies. However, the distribution of normal images estimated by current systems tends to be unstable for classes of normal images that are under-represented in the training set, and the anomaly identification criteria commonly explored in the field does not work well for multi-scale structural and non-structural anomalies. In this paper, we introduce an unsupervised anomaly detection and localisation method designed to address these two issues. More specifically, we introduce a normal image distribution estimation method that is robust to under-represented classes of normal images -- this method is based on adversarially interpolated descriptors from training images and a Gaussian classifier. We also propose a new anomaly identification criterion that can accurately detect and localise multi-scale structural and non-structural anomalies. In extensive experiments on MNIST, Fashion MNIST, CIFAR10 and MVTec AD data sets, our approach shows better results than the current state of the arts in the standard experimental setup for unsupervised anomaly detection and localisation. Code is available at https://github.com/tianyu0207/IGD.
更多查看译文
关键词
anomaly,detection,multi-scale
AI 理解论文
溯源树
样例
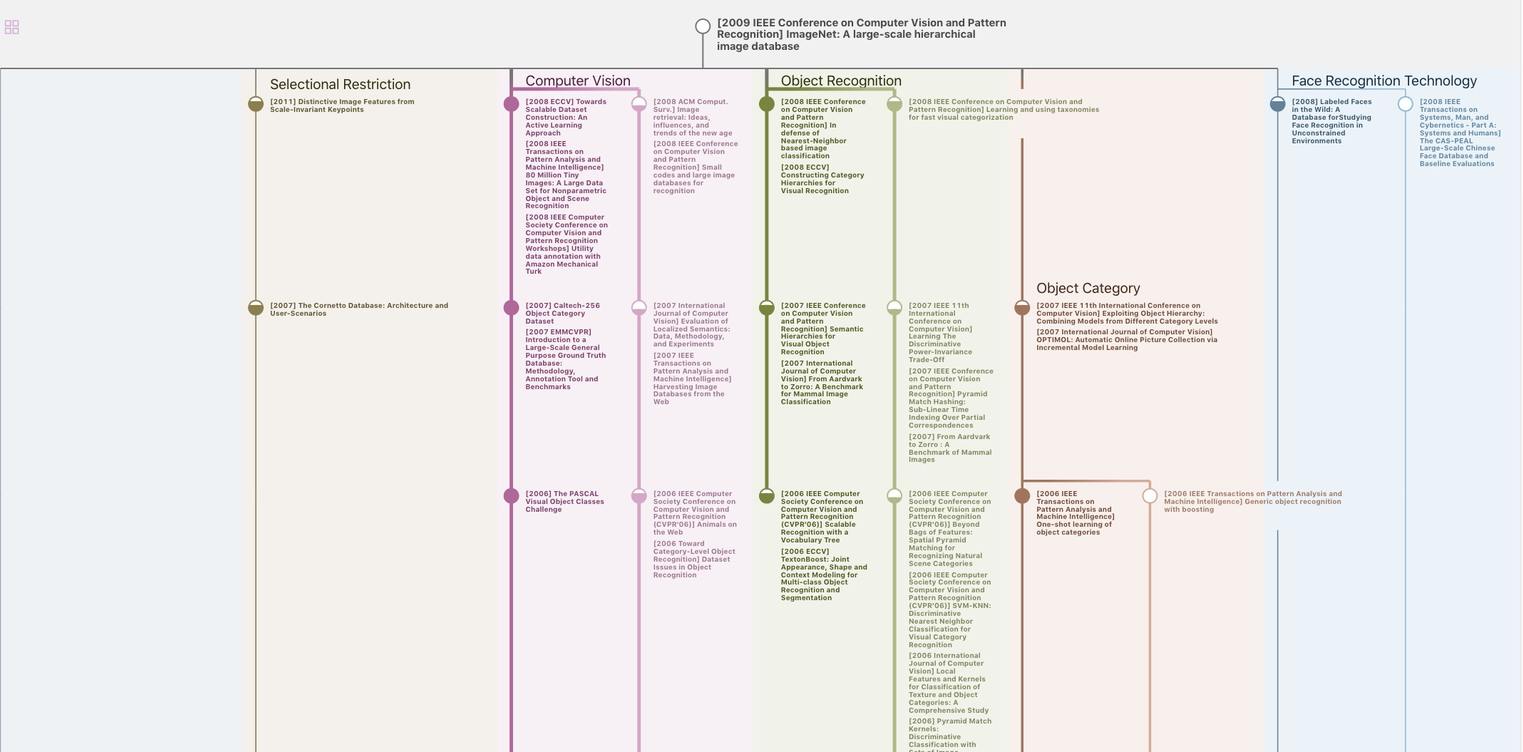
生成溯源树,研究论文发展脉络
Chat Paper
正在生成论文摘要