Iterative Weak Learnability and Multi-Class AdaBoost
arxiv(2021)
摘要
We construct an efficient recursive ensemble algorithm for the multi-class classification problem, inspired by SAMME (Zhu, Zou, Rosset, and Hastie (2009)). We strengthen the weak learnability condition in Zhu, Zou, Rosset, and Hastie (2009) by requiring that the weak learnability condition holds for any subset of labels with at least two elements. This condition is simpler to check than many proposed alternatives (e.g., Mukherjee and Schapire (2013)). As SAMME, our algorithm is reduced to the Adaptive Boosting algorithm (Schapire and Freund (2012)) if the number of labels is two, and can be motivated as a functional version of the steepest descending method to find an optimal solution. In contrast to SAMME, our algorithm's final hypothesis converges to the correct label with probability 1. For any number of labels, the probability of misclassification vanishes exponentially as the training period increases. The sum of the training error and an additional term, that depends only on the sample size, bounds the generalization error of our algorithm as the Adaptive Boosting algorithm.
更多查看译文
关键词
iterative weak learnability,multi-class
AI 理解论文
溯源树
样例
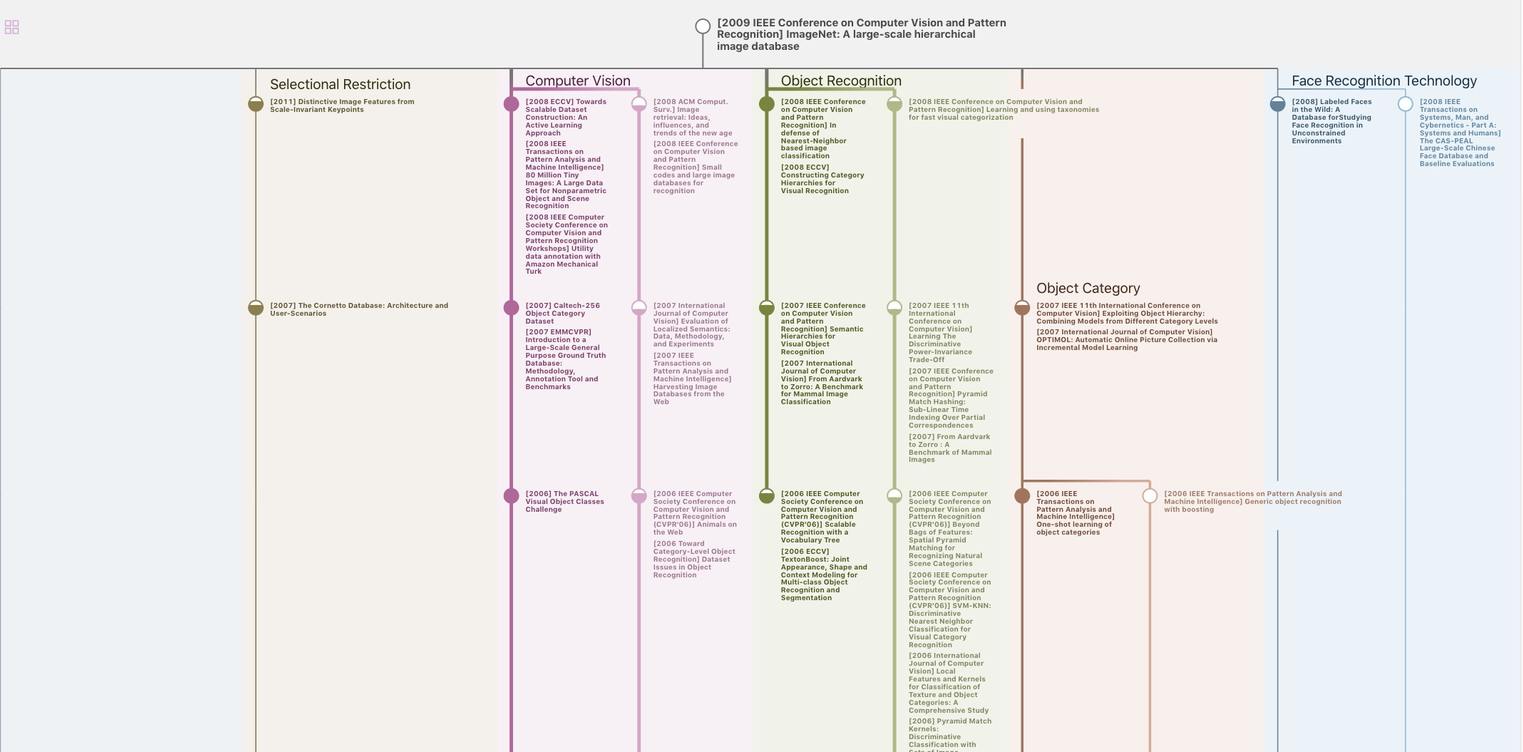
生成溯源树,研究论文发展脉络
Chat Paper
正在生成论文摘要