Absolute Value Constraint: The Reason for Invalid Performance Evaluation Results of Neural Network Models for Stock Price Prediction
arxiv(2021)
摘要
Neural networks for stock price prediction(NNSPP) have been popular for decades. However, most of its study results remain in the research paper and cannot truly play a role in the securities market. One of the main reasons leading to this situation is that the prediction error(PE) based evaluation results have statistical flaws. Its prediction results cannot represent the most critical financial direction attributes. So it cannot provide investors with convincing, interpretable, and consistent model performance evaluation results for practical applications in the securities market. To illustrate, we have used data selected from 20 stock datasets over six years from the Shanghai and Shenzhen stock market in China, and 20 stock datasets from NASDAQ and NYSE in the USA. We implement six shallow and deep neural networks to predict stock prices and use four prediction error measures for evaluation. The results show that the prediction error value only partially reflects the model accuracy of the stock price prediction, and cannot reflect the change in the direction of the model predicted stock price. This characteristic determines that PE is not suitable as an evaluation indicator of NNSPP. Otherwise, it will bring huge potential risks to investors. Therefore, this paper establishes an experiment platform to confirm that the PE method is not suitable for the NNSPP evaluation, and provides a theoretical basis for the necessity of creating a new NNSPP evaluation method in the future.
更多查看译文
关键词
stock price prediction,neural network models,neural network,invalid performance evaluation results
AI 理解论文
溯源树
样例
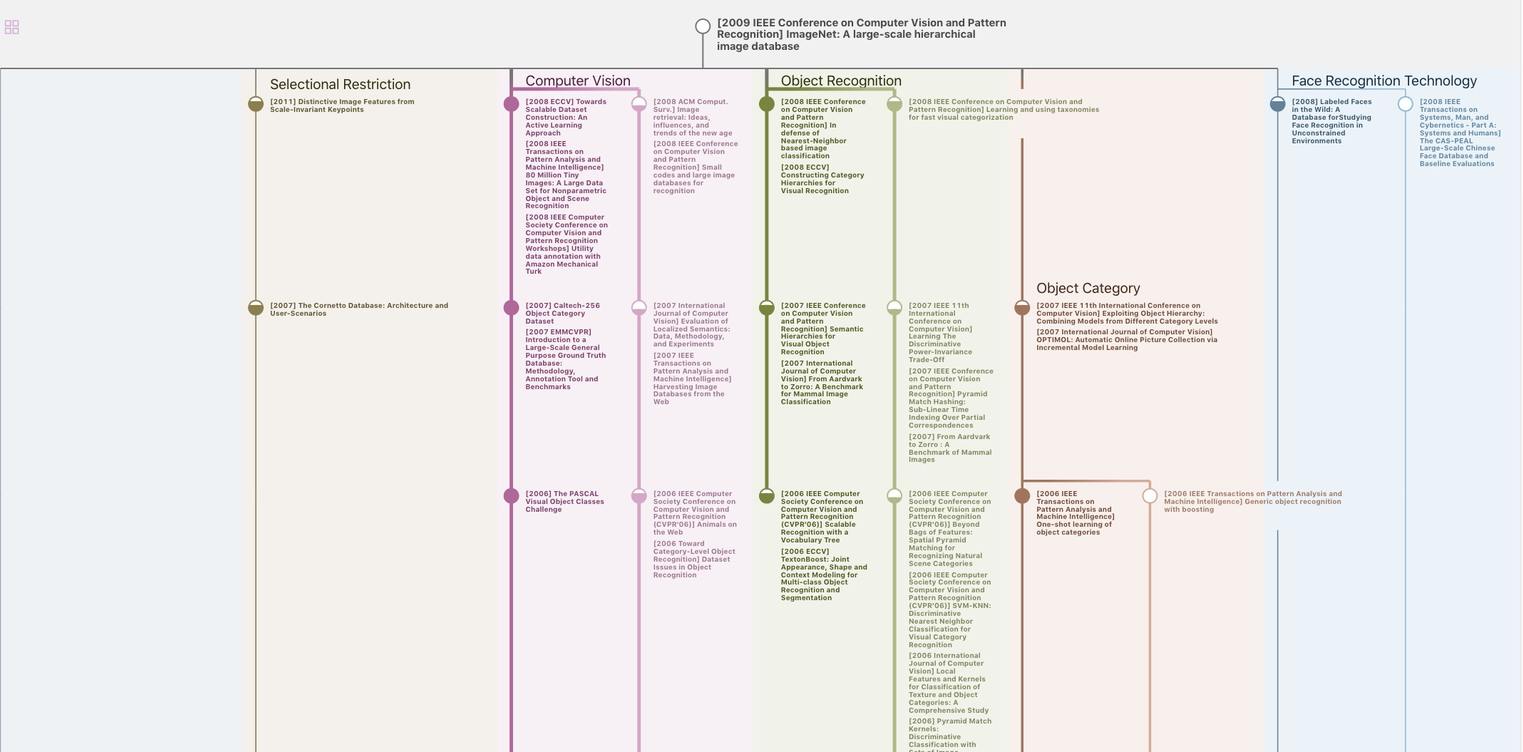
生成溯源树,研究论文发展脉络
Chat Paper
正在生成论文摘要