Sdf-Bayes: Cautious Optimism In Safe Dose-Finding Clinical Trials With Drug Combinations And Heterogeneous Patient Groups
24TH INTERNATIONAL CONFERENCE ON ARTIFICIAL INTELLIGENCE AND STATISTICS (AISTATS)(2021)
摘要
Phase I clinical trials are designed to test the safety (non-toxicity) of drugs and find the maximum tolerated dose (MTD). This task becomes significantly more challenging when multiple-drug dose-combinations (DC) are involved, due to the inherent conict between the exponentially increasing DC candidates and the limited patient budget. This paper proposes a novel Bayesian design, SDF-Bayes, for finding the MTD for drug combinations in the presence of safety constraints. Rather than the conventional principle of escalating or de-escalating the current dose of one drug (perhaps alternating between drugs), SDF-Bayes proceeds by cautious optimism: it chooses the next DC that, on the basis of current information, is most likely to be the MTD (optimism), subject to the constraint that it only chooses DCs that have a high probability of being safe (caution). We also propose an extension, SDF-Bayes-AR, that accounts for patient heterogeneity and enables heterogeneous patient recruitment. Extensive experiments based on both synthetic and realworld datasets demonstrate the advantages of SDF-Bayes over state of the art DC trial designs in terms of accuracy and safety.
更多查看译文
关键词
drug combinations,clinical trials,sdf-bayes,dose-finding
AI 理解论文
溯源树
样例
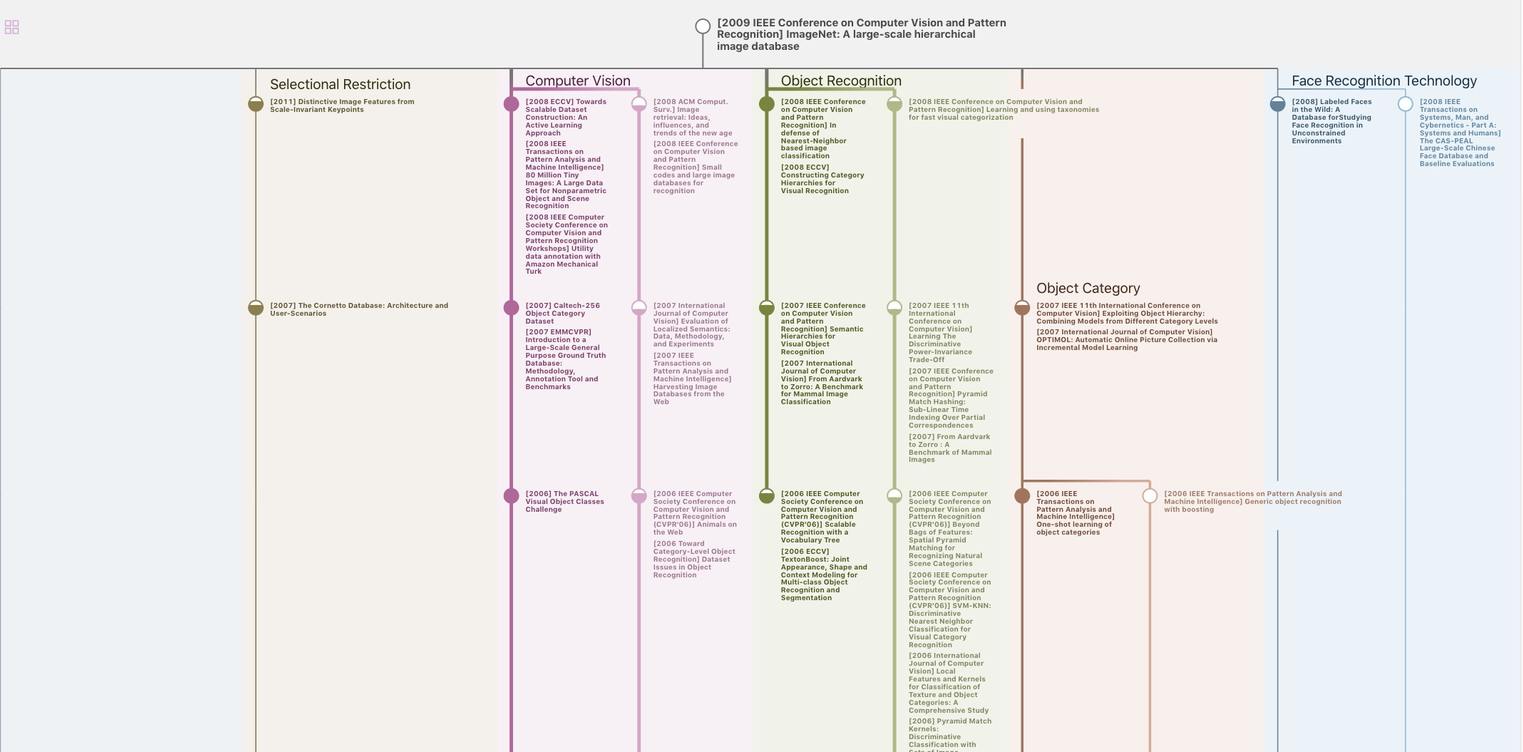
生成溯源树,研究论文发展脉络
Chat Paper
正在生成论文摘要