A Coding Theory Perspective on MultiplexedMolecular Profiling of Biological Tissues
arxiv(2021)
摘要
High-throughput and quantitative experimental technologies are experiencing rapid advances in the biological sciences. One important recent technique is multiplexed fluorescence in situ hybridization (mFISH), which enables the identification and localization of large numbers of individual strands of RNA within single cells. Core to that technology is a coding problem: with each RNA sequence of interest being a codeword, how to design a codebook of probes, and how to decode the resulting noisy measurements? Published work has relied on assumptions of uniformly distributed codewords and binary symmetric channels for decoding and to a lesser degree for code construction. Here we establish that both of these assumptions are inappropriate in the context of mFISH experiments and substantial decoding performance gains can be obtained by using more appropriate, less classical, assumptions. We propose a more appropriate asymmetric channel model that can be readily parameterized from data and use it to develop a maximum a posteriori (MAP) decoders. We show that false discovery rate for rare RNAs, which is the key experimental metric, is vastly improved with MAP decoders even when employed with the existing sub-optimal codebook. Using an evolutionary optimization methodology, we further show that by permuting the codebook to better align with the prior, which is an experimentally straightforward procedure, significant further improvements are possible.
更多查看译文
关键词
coding theory perspective,multiplexed molecular profiling,biological tissues,quantitative experimental technologies,biological sciences,multiplexed fluorescence,situ hybridization,localization,single cells,coding problem,codeword,resulting noisy measurements,uniformly distributed codewords,binary symmetric channels,code construction,mFISH experiments,substantial decoding performance gains,appropriate asymmetric channel model,posteriori decoders,false discovery rate,rare RNAs,MAP decoders,evolutionary optimization methodology
AI 理解论文
溯源树
样例
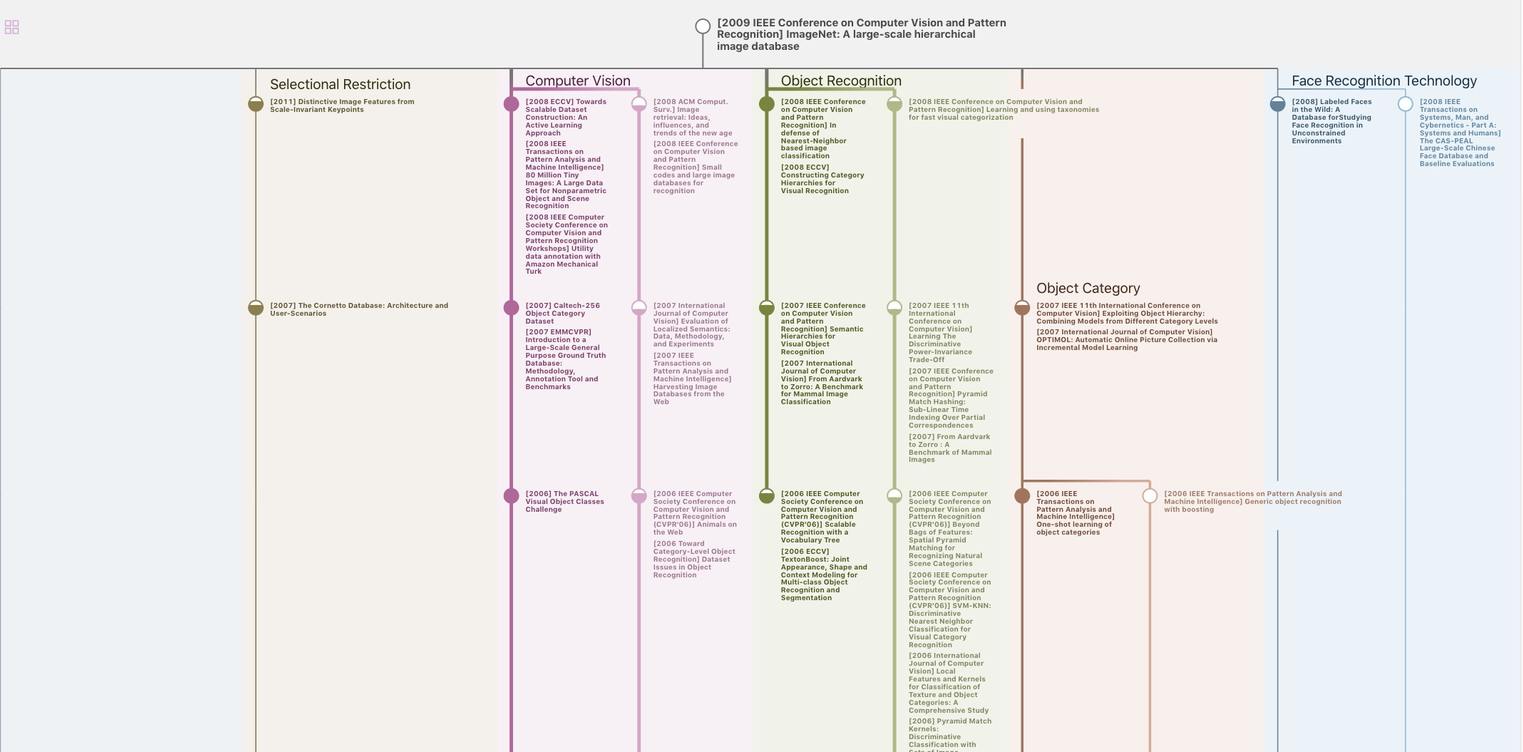
生成溯源树,研究论文发展脉络
Chat Paper
正在生成论文摘要