A CNN-Based Length-Aware Cascade Road Damage Detection Approach.
Sensors(2021)
摘要
Accurate and robust detection of road damage is essential for public transportation safety. Currently, deep convolutional neural networks (CNNs)-based road damage detection algorithms to localize and classify damage with a bounding box have achieved remarkable progress. However, research in this field fails to take into account two key characteristics of road damage: weak semantic information and abnormal geometric properties, resulting in inappropriate feature representation and suboptimal detection results. To boost the performance, we propose a CNN-based cascaded damage detection network, called CrdNet. The proposed model has three parts: (1) We introduce a novel backbone network, named LrNet, that reuses low-level features and mixes suitable range dependency features to learn high-to-low level feature fusions for road damage weak semantic information representation. (2) We apply multi-scale and multiple aspect ratios anchor mechanism to generate high-quality positive samples regarding the damage with abnormal geometric properties for network training. (3) We designed an adaptive proposal assignment strategy and performed cascade predictions on corresponding branches that can establish different range dependencies. The experiments show that the proposed method achieves mean average precision (mAP) of 90.92% on a collected road damage dataset, demonstrating the good performance and robustness of the model.
更多查看译文
关键词
deep convolutional neural network (CNN),road damage detection,abnormal geometric properties,length-aware,multi-scale attention
AI 理解论文
溯源树
样例
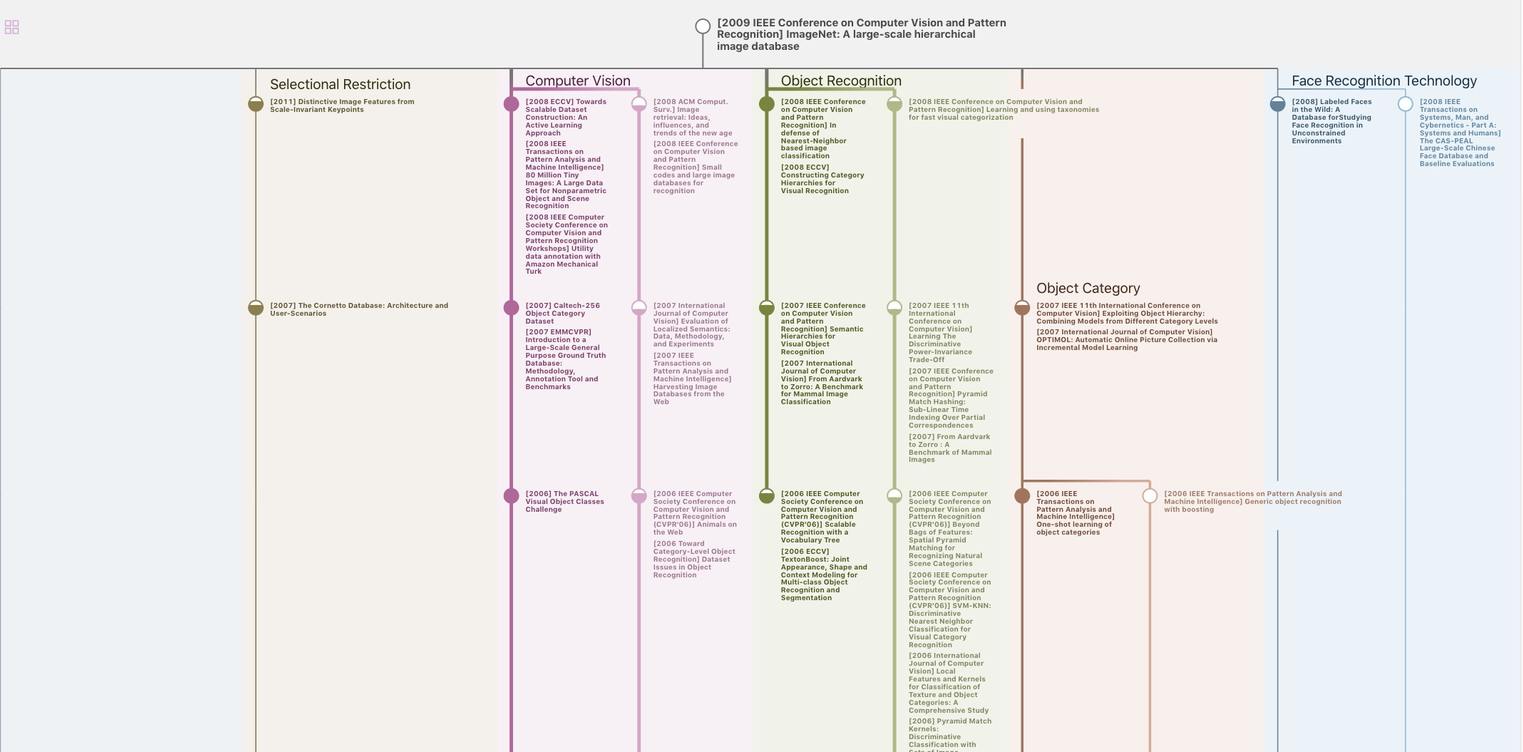
生成溯源树,研究论文发展脉络
Chat Paper
正在生成论文摘要