A classifier for spurious astrometric solutions in Gaia eDR3
MONTHLY NOTICES OF THE ROYAL ASTRONOMICAL SOCIETY(2022)
摘要
The Gaia early Data Release 3 has delivered exquisite astrometric data for 1.47 billion sources, which is revolutionizing many fields in astronomy. For a small fraction of these sources, the astrometric solutions are poor, and the reported values and uncertainties may not apply. Before any analysis, it is important to recognize and excise these spurious results - this is commonly done by means of quality flags in the Gaia catalogue. Here, we devise a means of separating 'good' from 'bad' astrometric solutions that is an order of magnitude cleaner than any single flag: 99.3 per cent pure and 97.3 per cent complete, as validated on our test data. We devise an extensive sample of manifestly bad astrometric solutions, with parallax that is negative at >= 4.5 sigma; and a corresponding sample of presumably good solutions, including sources in HEALPIX pixels on the sky that do not contain such negative parallaxes, and sources that fall on the main sequence in a colour-absolute magnitude diagram. We then train a neural network that uses 17 pertinent Gaia catalogue entries and information about nearby sources to discriminate between these two samples, captured in a single 'astrometric fidelity' parameter. A diverse set of verification tests shows that our approach works very cleanly, including for sources with positive parallaxes. The main limitations of our approach are in the very low signal-to-noise ratio and the crowded regime. Our astrometric fidelities for all of eDR3 can be queried via the Virtual Observatory, our code and data are public.
更多查看译文
关键词
space vehicles: instruments, virtual observatory tools, Galaxy: kinematics and dynamics, Galaxy: stellar content
AI 理解论文
溯源树
样例
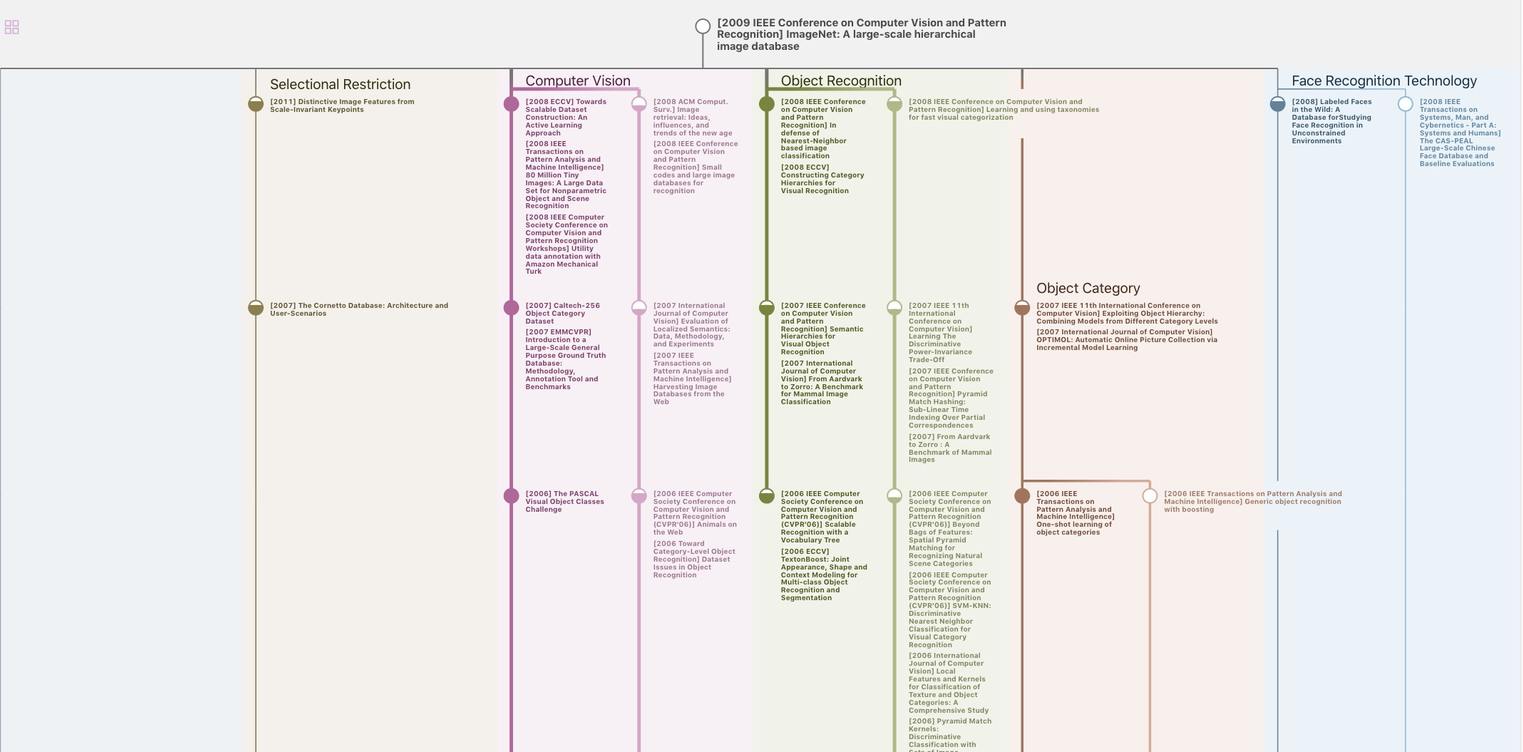
生成溯源树,研究论文发展脉络
Chat Paper
正在生成论文摘要