On the capacity of deep generative networks for approximating distributions
Neural Networks(2022)
摘要
We study the efficacy and efficiency of deep generative networks for approximating probability distributions. We prove that neural networks can transform a low-dimensional source distribution to a distribution that is arbitrarily close to a high-dimensional target distribution, when the closeness is measured by Wasserstein distances and maximum mean discrepancy. Upper bounds of the approximation error are obtained in terms of the width and depth of neural network. Furthermore, it is shown that the approximation error in Wasserstein distance grows at most linearly on the ambient dimension and that the approximation order only depends on the intrinsic dimension of the target distribution. On the contrary, when f-divergences are used as metrics of distributions, the approximation property is different. We show that in order to approximate the target distribution in f-divergences, the dimension of the source distribution cannot be smaller than the intrinsic dimension of the target distribution.
更多查看译文
关键词
Deep ReLU networks,Generative adversarial networks,Approximation complexity,Wasserstein distance,Maximum mean discrepancy
AI 理解论文
溯源树
样例
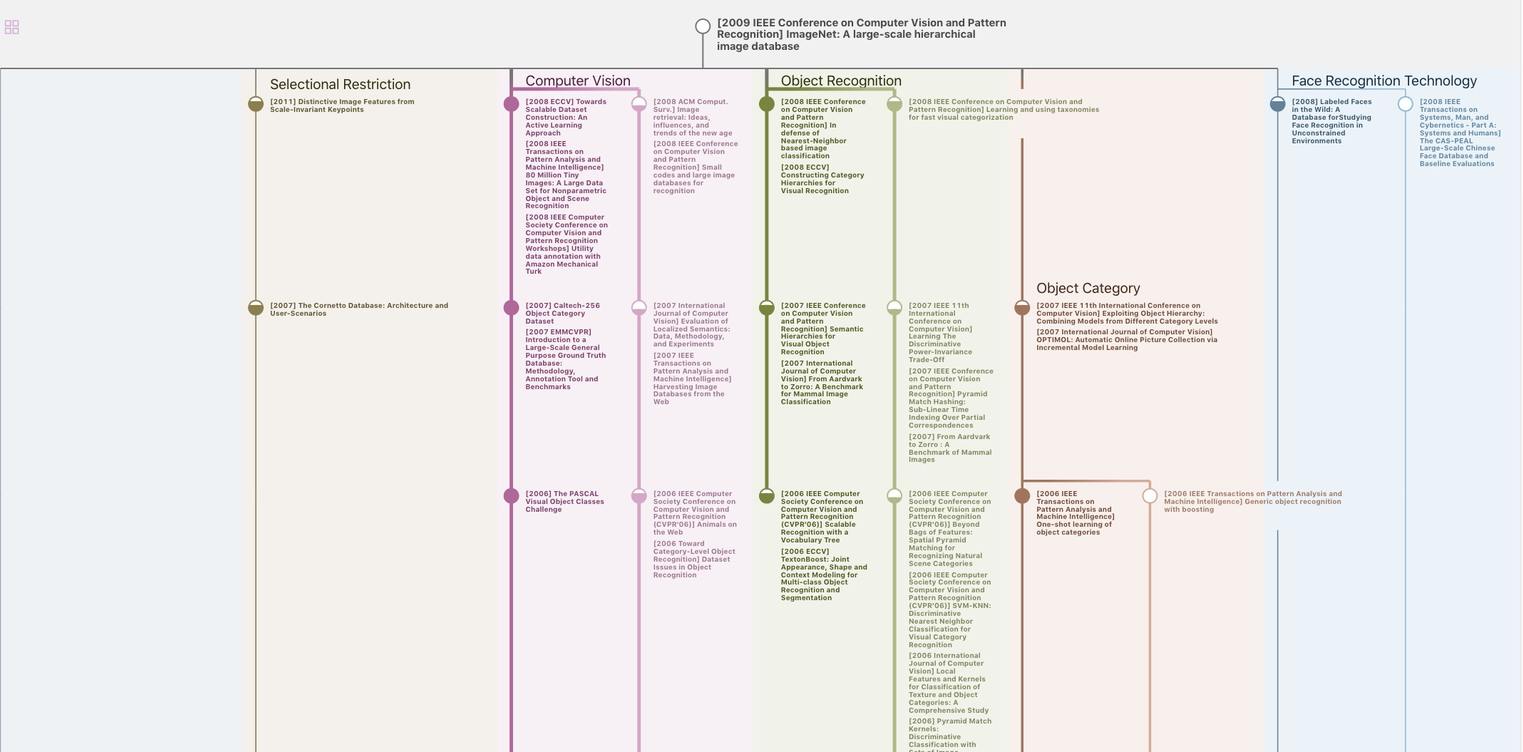
生成溯源树,研究论文发展脉络
Chat Paper
正在生成论文摘要