The problem of perfect predictors in statistical spike train models
arxiv(2021)
摘要
Generalized Linear Models (GLMs) have been used extensively in statistical models of spike train data. However, the IRLS algorithm, which is often used to fit such models, can fail to converge in situations where response and non-response can be separated by a single predictor or a linear combination of multiple predictors. Such situations are likely to arise in many neural systems due to properties such as refractoriness and incomplete sampling of the signals that influence spiking. In this paper, we describe multiple classes of approaches to address this problem: Standard IRLS with a fixed iteration limit, computing the maximum likelihood solution in the limit, Bayesian estimation, regularization, change of basis, and modifying the search parameters. We demonstrate a specific application of each of these methods to spiking data from rat somatosensory cortex and discuss the advantages and disadvantages of each. We also provide an example of a roadmap for selecting a method based on the problem's particular analysis issues and scientific goals.
更多查看译文
关键词
statistical spike train models,perfect predictors
AI 理解论文
溯源树
样例
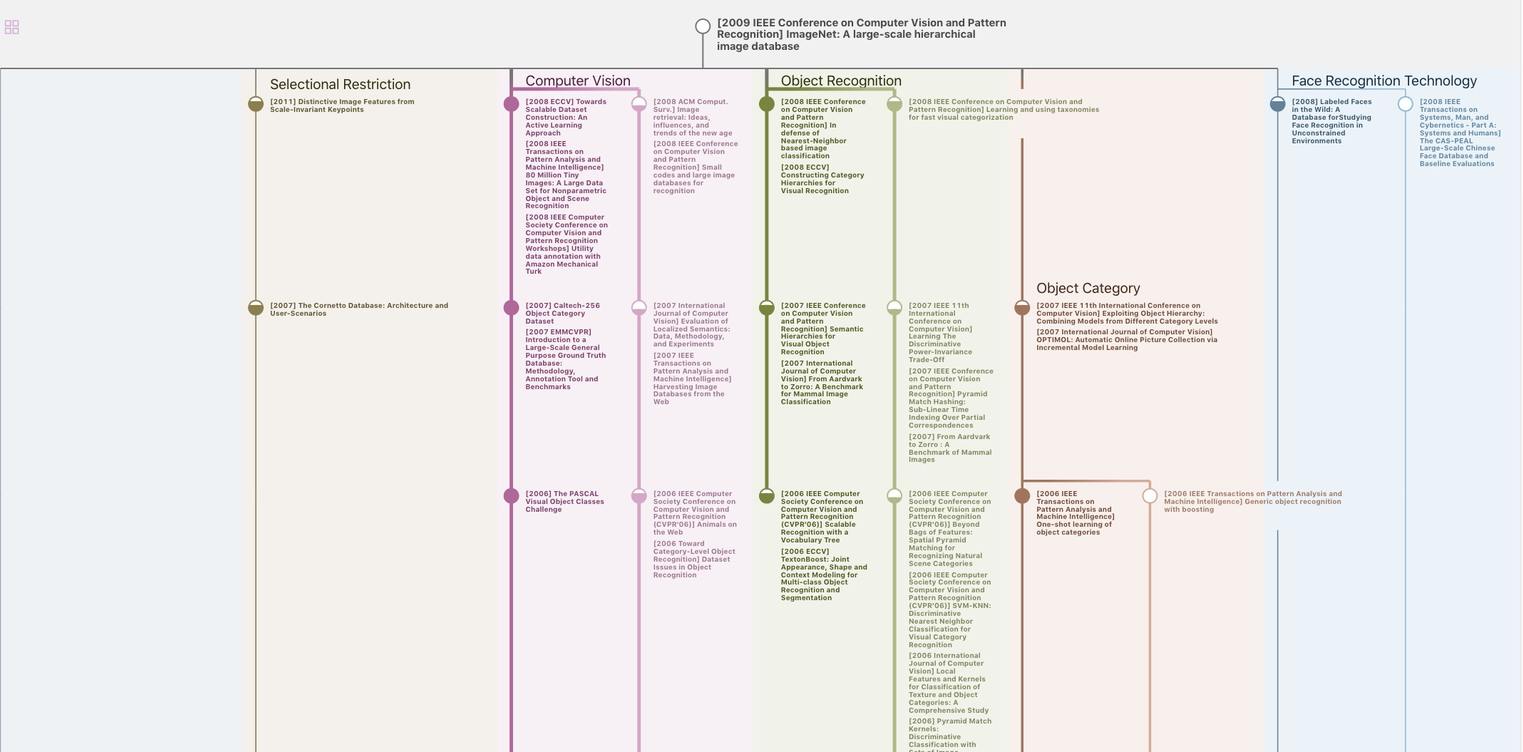
生成溯源树,研究论文发展脉络
Chat Paper
正在生成论文摘要