Deep Learning-based Adaptive Beamforming for mmWave Wireless Body Area Network.
GLOBECOM(2020)
摘要
Artificial intelligence (AI) is becoming a mainstream for telecommunication industry. With the utilization of millimeter-wave in 5G network, it becomes feasible to use beamforming techniques for on-body sensors in Wireless Body Area Network (WBAN) applications. Thus, there is a need for developing bearnforming algorithms that can optimize WBAN network performance and a realistic dataset that can be used for training, testing, and benchmarking of the algorithms. Thus, we propose a dataset generation method for mmWave WBAN that utilizes computer vision and an adaptive deep learning-based algorithm for performance optimization of mmWave WBAN beamforming. Two major ideas are proposed: First, collecting human poses from estimation of 3D human poses in videos and generating more realistic poses using generative adversarial nets (GAN) are adopted; second, a GAN aims to predict the next beamforming directions using the previous set of directions as inputs. With available labeled human pose videos, the WBAN dataset we generate provides a sufficient amount of samples for training, testing, and benchmarking of beamforming algorithms. Additionally, the proposed adaptive beamforming algorithm does not require any intrusive data gathering methods. Our numerical studies show the advantages of our proposed approaches.
更多查看译文
关键词
Deep learning,beamforming,mmWave
AI 理解论文
溯源树
样例
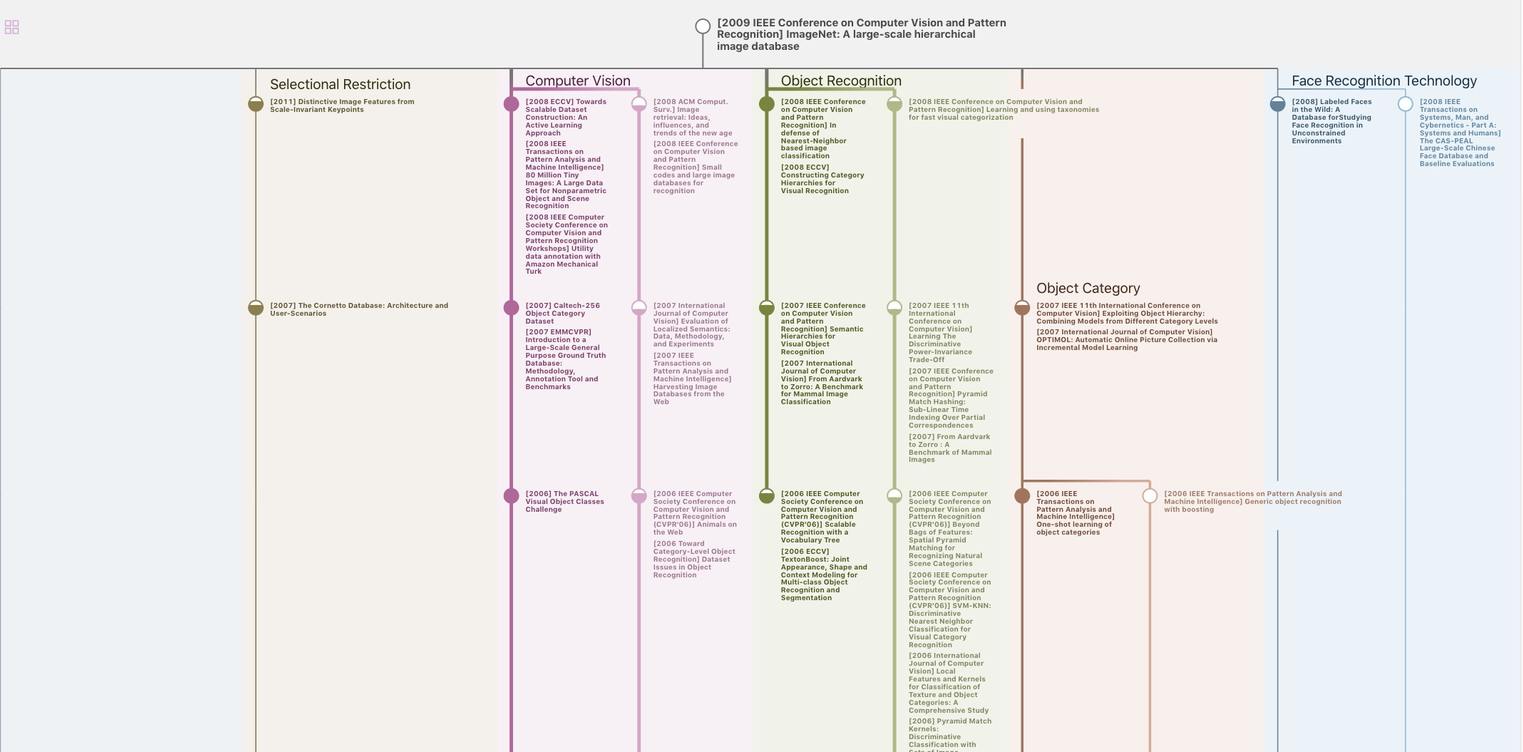
生成溯源树,研究论文发展脉络
Chat Paper
正在生成论文摘要