Gaussian Models for CSI Fingerprinting in Practical Indoor Environment Identification
2020 IEEE GLOBAL COMMUNICATIONS CONFERENCE (GLOBECOM)(2020)
摘要
It is not uncommon to experience highly dynamic channels in indoor environments due to time-varying signals as well as moving reflectors and scatterers. This greatly affects the performance of wireless sensing systems that use received signal strength indicator (RSSI) and channel state information (CSI) fingerprints for indoor positioning and event detection. Solutions to this dynamic channel problem often involve laborintensive database maintenance and customized hardware. With this, we present Gaussian models that can withstand temporal and environmental dynamics in practical indoor environments using off-the-shelf devices in this paper. Although systems employing Gaussian models have been previously proposed in the literature, most systems use RSSI instead of CSI to represent the wireless channel. By using a Gaussian distribution to model CSI fingerprints, which offer more abundant information regarding the channel dynamics than RSSI, we can exploit the variance inherent in the wireless channels. Our experiments demonstrate that the Gaussian classifier incurs minimal delay of less than 4 seconds and achieves high classification accuracy compared to other techniques. In particular, it achieves up to 50% and 150% performance improvement over the time-reversal resonating strength (TRRS) and the support vector machines (SVM) methods, respectively.
更多查看译文
关键词
Channel State Information (CSI), Gaussian Classifiers, Clustering, Wireless Sensing
AI 理解论文
溯源树
样例
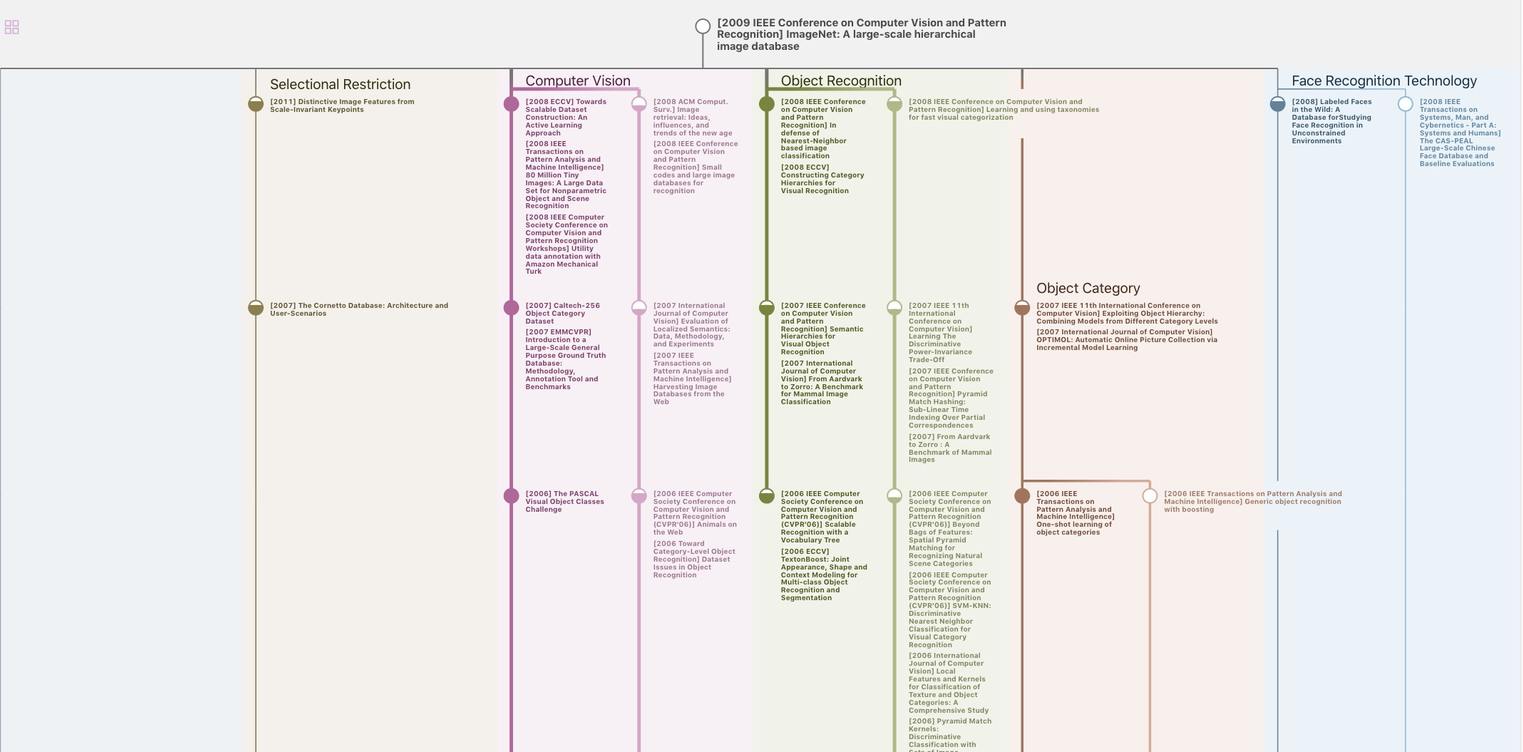
生成溯源树,研究论文发展脉络
Chat Paper
正在生成论文摘要