Towards Environment-independent Human Activity Recognition using Deep Learning and Enhanced CSI.
GLOBECOM(2020)
摘要
Deep learning has shown a strong potential in device-free human activity recognition (HAR). However, a fundamental challenge is ensuring accuracy, without re-training, when exposing a previously trained architecture to a new or unseen environment. To overcome the aforementioned challenge, this paper proposes an environment-robust channel state information (CSI) based HAR by leveraging the properties of a matching network (MatNet) and enhanced features (HAR-MN-EF). To improve the CSI quality, we propose a CSI cleaning and enhancement method (CSI-CE) that includes two key stages: activity-related information extraction (ARIE) and correlation feature extraction based on principal component analysis (CFE-PCA). The ARIE stage is able to effectively enhance the activity-dependent features whilst mitigating behavior-unrelated information. The CFE-PCA stage further improves the extracted features by filtering out the residual activity-unrelated data and the residual noise contained in signals from the former stage. The extracted features are then sequenced into the MatNet to create an environment-robust HAR. Experimental results confirm that an architecture trained by the proposed HAR-MN-EF can be directly adapted to a new environment, achieving reliable sensing accuracies without. requiring additional effort.
更多查看译文
关键词
WiFi, Device free sensing, Channel state information, Human activity recognition, One-shot learning
AI 理解论文
溯源树
样例
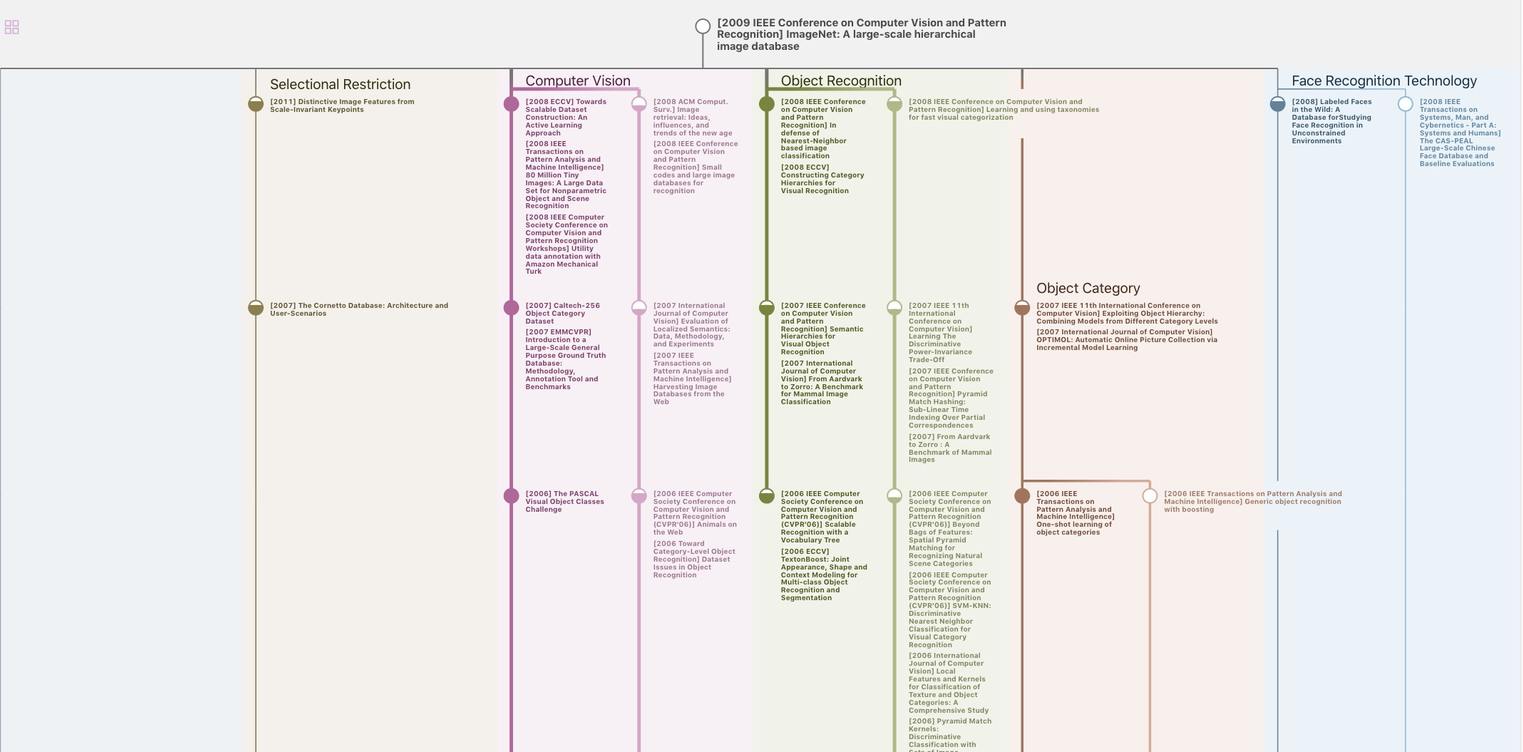
生成溯源树,研究论文发展脉络
Chat Paper
正在生成论文摘要