Manifold Repairing, Reconstruction and Denoising from Scattered Data in High-Dimension
arxiv(2021)
摘要
We consider a problem of great practical interest: the repairing and recovery of a low-dimensional manifold embedded in high-dimensional space from noisy scattered data. Suppose that we observe a point cloud sampled from the low-dimensional manifold, with noise, and let us assume that there are holes in the data. Can we recover missing information inside the holes? While in low-dimension the problem was extensively studied, manifold repairing in high dimension is still an open problem. We introduce a new approach, called Repairing Manifold Locally Optimal Projection (R-MLOP), that expands the MLOP method introduced by Faigenbaum-Golovin et al. in 2020, to cope with manifold repairing in low and high-dimensional cases. The proposed method can deal with multiple holes in a manifold. We prove the validity of the proposed method, and demonstrate the effectiveness of our approach by considering different manifold topologies, for single and multiple holes repairing, in low and high dimensions.
更多查看译文
关键词
scattered data,denoising,reconstruction,high-dimension
AI 理解论文
溯源树
样例
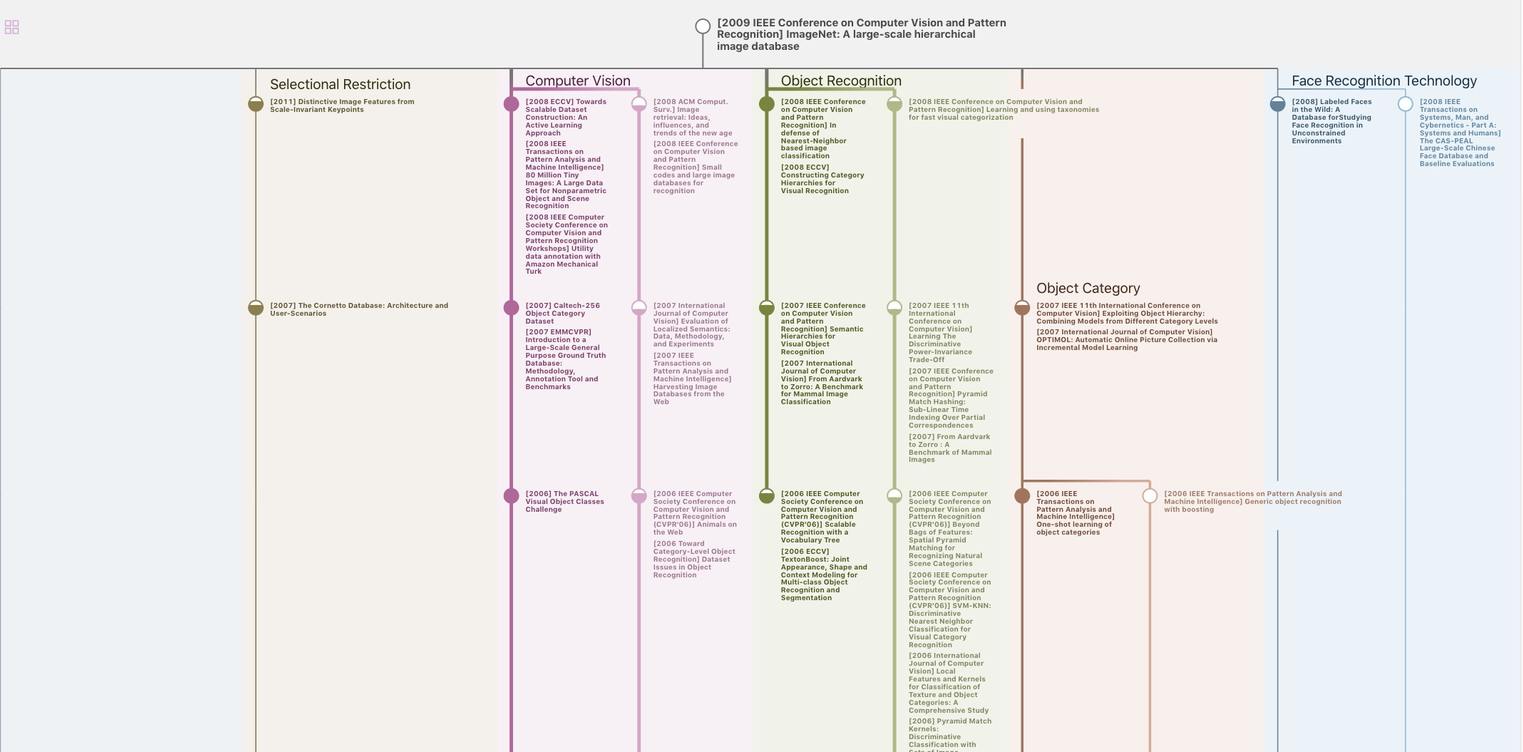
生成溯源树,研究论文发展脉络
Chat Paper
正在生成论文摘要