Debiased inference on heterogeneous quantile treatment effects with regression rank scores
JOURNAL OF THE ROYAL STATISTICAL SOCIETY SERIES B-STATISTICAL METHODOLOGY(2024)
摘要
Understanding treatment effect heterogeneity is vital to many scientific fields because the same treatment may affect different individuals differently. Quantile regression provides a natural framework for modelling such heterogeneity. We propose a new method for inference on heterogeneous quantile treatment effects (HQTE) in the presence of high-dimensional covariates. Our estimator combines an l(1)-penalised regression adjustment with a quantile-specific bias correction scheme based on rank scores. We study the theoretical properties of this estimator, including weak convergence and semi-parametric efficiency of the estimated HQTE process. We illustrate the finite-sample performance of our approach through simulations and an empirical example, dealing with the differential effect of statin usage for lowering low-density lipoprotein cholesterol levels for the Alzheimer's disease patients who participated in the UK Biobank study.
更多查看译文
关键词
causal inference,debiased Inference,high-dimensional data,quantile regression,semi-parametric efficiency
AI 理解论文
溯源树
样例
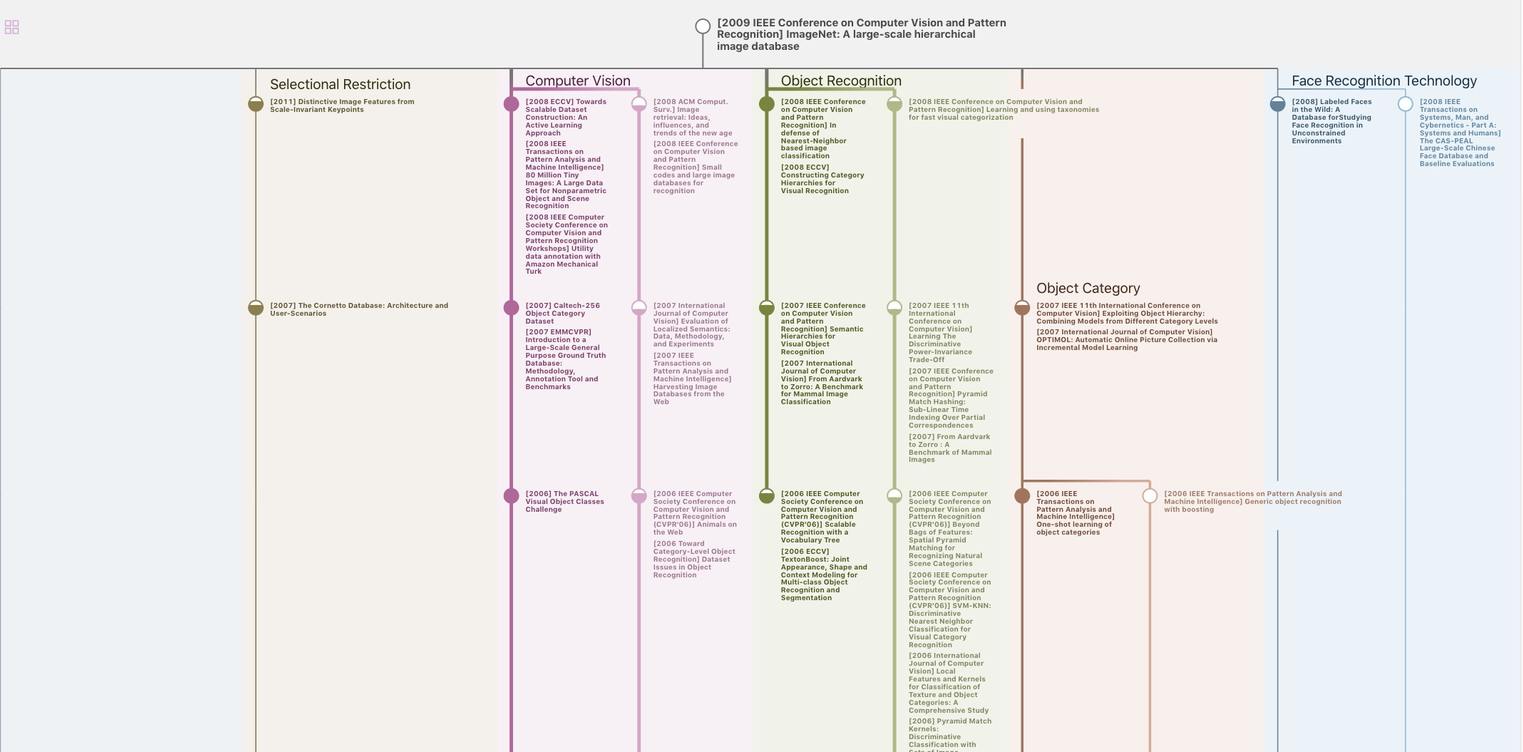
生成溯源树,研究论文发展脉络
Chat Paper
正在生成论文摘要