Learning Goal Conditioned Socially Compliant Navigation from Demonstration Using Risk-Based Features.
IEEE robotics & automation letters(2021)
摘要
One of the main challenges of operating mobile robots in social environments is the safe and fluid navigation therein, specifically the ability to share a space with other human inhabitants by complying with the explicit and implicit rules that we humans follow during navigation. While these rules come naturally to us, they resist simple and explicit definitions. In this letter, we present a learning-based solution to address the question of socially compliant navigation, which is to navigate while maintaining adherence to the navigational policies a person might use. We infer these policies by learning from human examples using inverse reinforcement learning techniques. In particular, this letter contributes an efficient sampling-based approximation to enable model-free deep inverse reinforcement learning, and a goal conditioned risk-based feature representation that adequately captures local information surrounding the agent. We validate our approach by comparing against a classical algorithm and a reinforcement learning agent and evaluate our feature representation against similar feature representations from the literature. We find that the combination of our proposed method and our feature representation produce higher quality trajectories and that our proposed feature representation plays a critical role in successful navigation.
更多查看译文
关键词
Navigation,Trajectory,Reinforcement learning,Entropy,Computational modeling,Two dimensional displays,Robot sensing systems,Inverse reinforcement learning,learning from demonstration,motion and path planning,robot navigation,social navigation
AI 理解论文
溯源树
样例
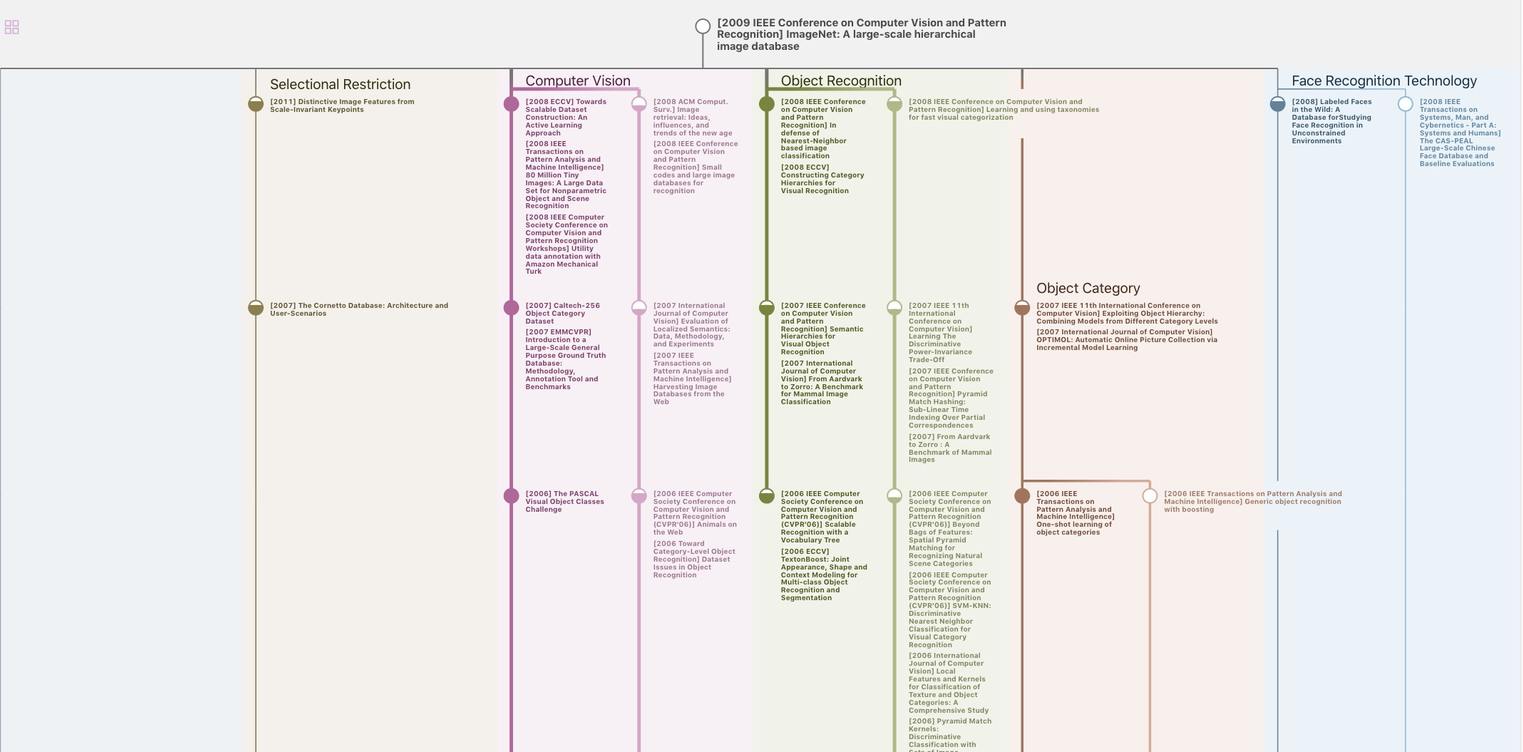
生成溯源树,研究论文发展脉络
Chat Paper
正在生成论文摘要