Reliable Multiview Learning for Spectral Clustering
2020 7th International Conference on Dependable Systems and Their Applications (DSA)(2020)
摘要
The purpose of multiview clustering is to improve clustering performance by using multiview information. At present, the research of multiview mainly focuses on the multiview similarity learning. However, the existing multiview similarity learning methods have two drawbacks. First of all, similarity learning is learned based on the fixed graph learned from each view separately, thus ignoring the potential relationship between the views. Secondly, the real data is often destroyed. When the data are contaminated with noise or outlier, the learned similarity graph is unreliable. Therefore, this paper proposes a Reliable Multiview Graph Learning method (RMGL). This method can deal with noisy data. By constructing a data recovery model to restore the true distribution structure of data from each view, the reliability of similarity learning could be guaranteed. Then, a reliable neighbor similarity is obtained with multiview adaptive graph learning. Experimental results on several datasets show that the proposed method is superior to the existing methods on clustering performance and has strong robustness to noisy data.
更多查看译文
关键词
clustering,similarity learning,graph construction,noise removal
AI 理解论文
溯源树
样例
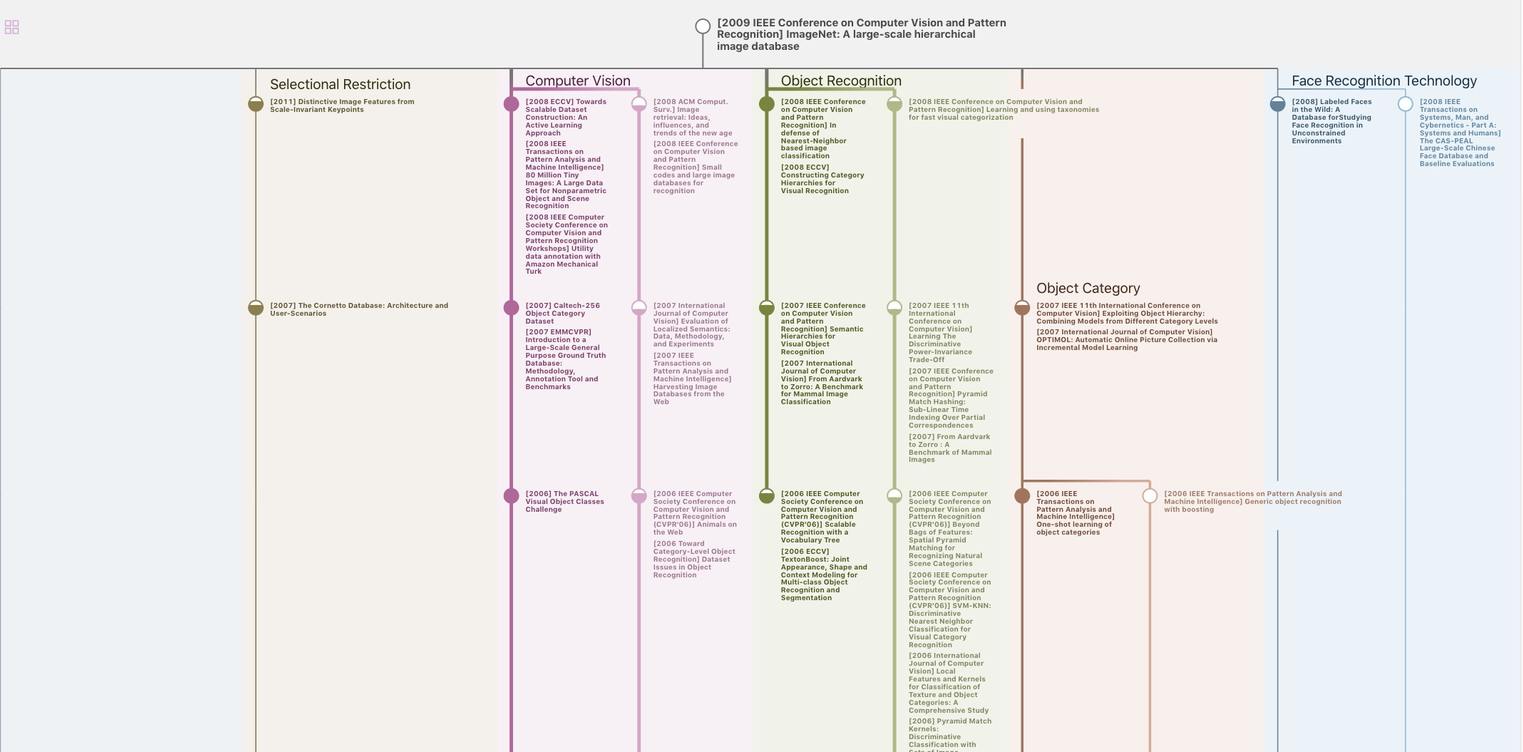
生成溯源树,研究论文发展脉络
Chat Paper
正在生成论文摘要