An Online Framework for Temporal Social Unrest Event Prediction Using News Stream
2020 International Conference on Cyber-Enabled Distributed Computing and Knowledge Discovery (CyberC)(2020)
摘要
Continuously assessing the risk of upcoming social unrest events and predict the likelihood of these events are of great importance. Thanks to the era of big data, people's understanding, experience, values, and ideology are mirrored in the organization of cyber space. In this paper, we propose an online prediction framework, using frequent subgraph patterns and hidden semi-Markov models (HSMMs). The feature called BoEAG (Bag-of-Event-Association-subGraph) is constructed based on frequents subgraph mining and the bag of word model. The new framework leverages the large scale digital history events captured from GDELT (Global Data on Events, Location, and Tone) to characterize the transitional process of the social unrest events' evolutionary stages, uncovering the underlying event development mechanics and formulates the social unrest event prediction as a sequence classification problem based on Bayes decision. Experimental results with data from Thailand demonstrate the effectiveness of the new framework, which outperforms the traditional HMM by 12.7% and the logistic regression 37.4%.
更多查看译文
关键词
frequent subgraph,graph mining,big data,algorithm
AI 理解论文
溯源树
样例
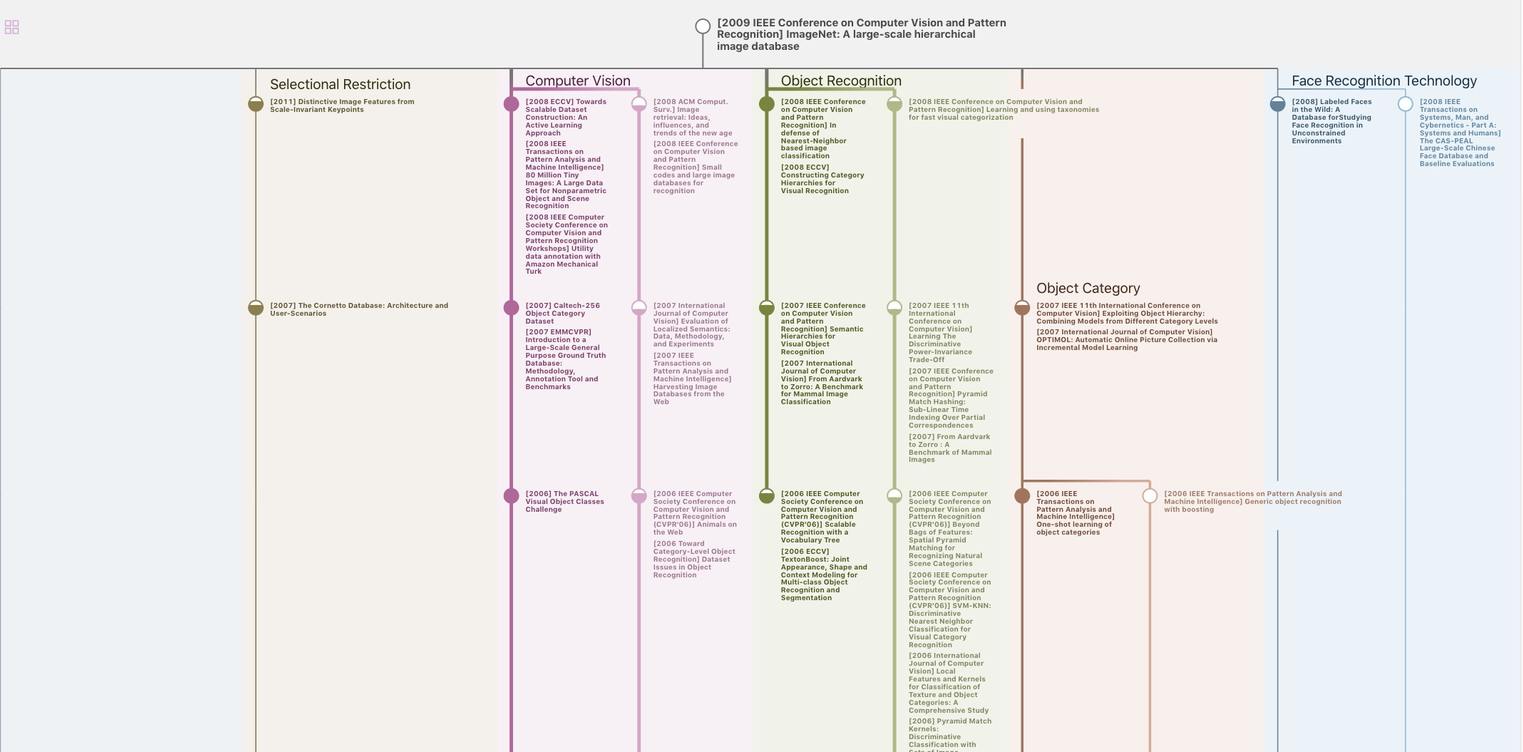
生成溯源树,研究论文发展脉络
Chat Paper
正在生成论文摘要