Efficient Navigation Using a Scalable, Biologically Inspired Spatial Representation.
Cognitive science(2020)
摘要
We present several experiments demonstrating the efficiency and scalability of a biologically inspired spatial representation on navigation tasks using artificial neural networks. Specifically, we demonstrate that encoding coordinates with Spatial Semantic Pointers (SSPs) outperforms six other proposed encoding methods when training a neural network to navigate to arbitrary goals in a 2D environment. The SSP representation naturally generalizes to larger spaces, as there is no definition of a boundary required (unlike most other methods). Additionally, we show how this navigational policy can be integrated into a larger system that combines memory retrieval and selflocalization to produce a behavioural agent capable of finding cued goal objects. We further demonstrate that explicitly incorporating a hexagonal grid cell-like structure in the generation of SSPs can improve performance. This biologically inspired spatial representation has been shown to be able to produce spiking neural models of spatial cognition. The link between SSPs and higher level cognition allows models using this representation to be seamlessly integrated into larger neural models to elicit complex behaviour.
更多查看译文
AI 理解论文
溯源树
样例
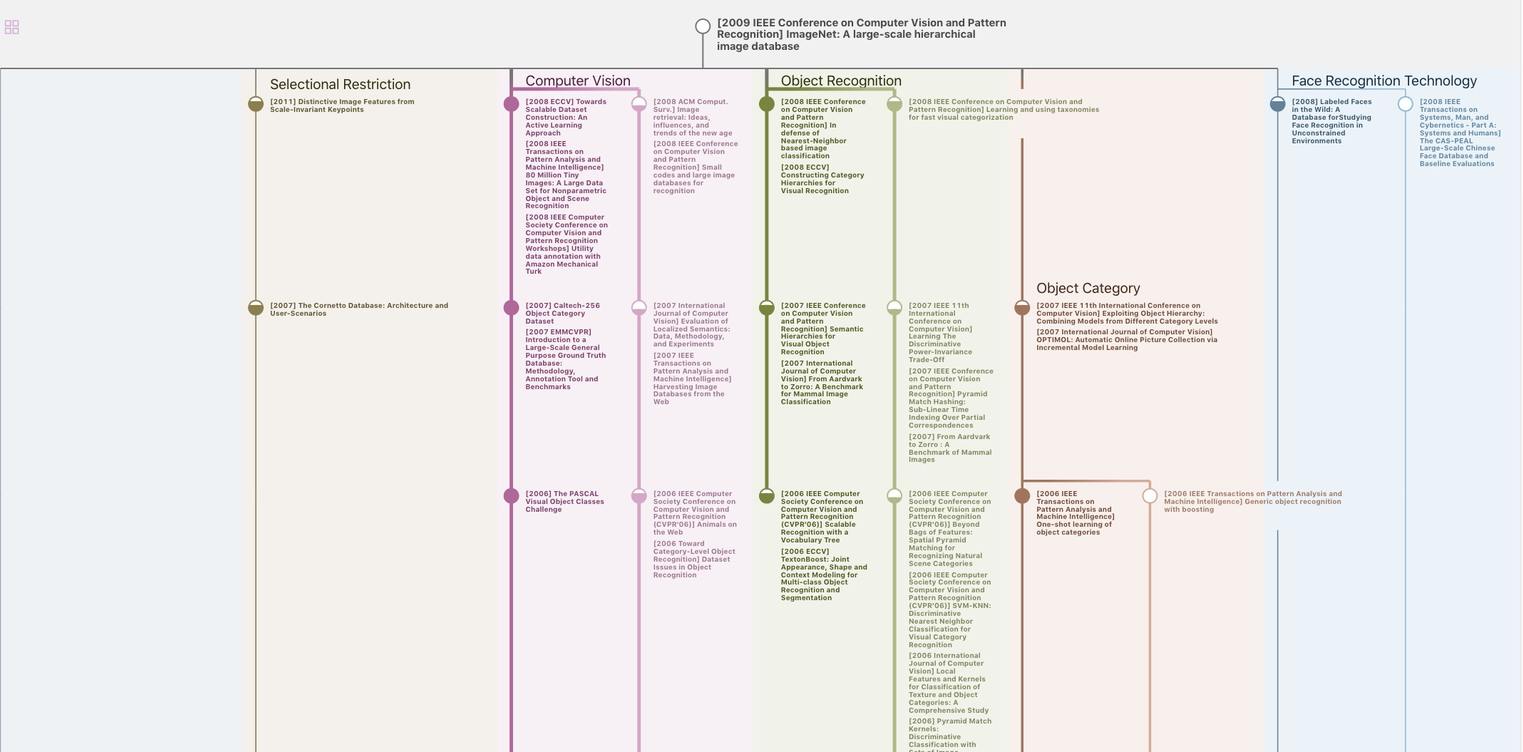
生成溯源树,研究论文发展脉络
Chat Paper
正在生成论文摘要