PREEMPT: Scalable Epidemic Interventions Using Submodular Optimization on Multi-GPU Systems
SC20: International Conference for High Performance Computing, Networking, Storage and Analysis(2020)
摘要
Preventing and slowing the spread of epidemics is achieved through techniques such as vaccination and social distancing. Given practical limitations on the number of vaccines and cost of administration, optimization becomes a necessity. Previous approaches using mathematical programming methods have shown to be effective but are limited by computational costs. In this work, we present PREEMPT, a new approach for intervention via maximizing the influence of vaccinated nodes on the network. We prove submodular properties associated with the objective function of our method so that it aids in construction of an efficient greedy approximation strategy. Consequently, we present a new parallel algorithm based on greedy hill climbing for PREEMPT, and present an efficient parallel implementation for distributed CPU-GPU heterogeneous platforms. Our results demonstrate that PREEMPT is able to achieve a significant reduction (up to 6.75×) in the percentage of people infected and up to 98% reduction in the peak of the infection on a city-scale network. We also show strong scaling results of PREEMPT on up to 128 nodes of the Summit supercomputer. Our parallel implementation is able to significantly reduce time to solution, from hours to minutes on large networks. This work represents a first-of-its-kind effort in parallelizing greedy hill climbing and applying it toward devising effective interventions for epidemics.
更多查看译文
关键词
Epidemic networks,vaccination,submodular,influence maximization,CPU-GPU,parallel algorithms
AI 理解论文
溯源树
样例
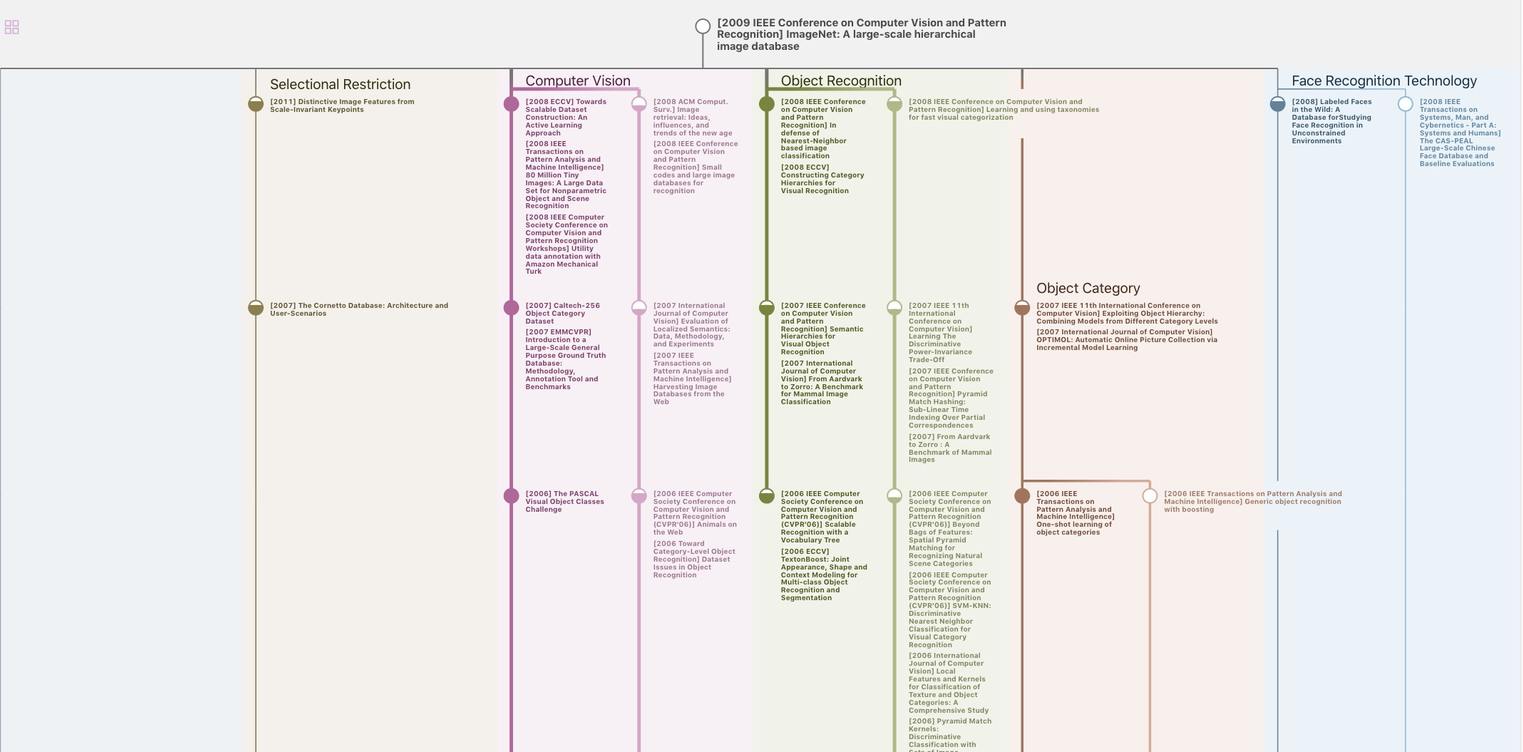
生成溯源树,研究论文发展脉络
Chat Paper
正在生成论文摘要