Differentiation of Pseudoprogression from True Progressionin Glioblastoma Patients after Standard Treatment: A Machine Learning Strategy Combinedwith Radiomics Features from T 1 -weighted Contrast-enhanced Imaging
BMC MEDICAL IMAGING(2021)
摘要
Background Based on conventional MRI images, it is difficult to differentiatepseudoprogression from true progressionin GBM patients after standard treatment, which isa critical issue associated with survival. The aim of this study was to evaluate the diagnostic performance of machine learning using radiomics modelfrom T 1 -weighted contrast enhanced imaging(T 1 CE) in differentiating pseudoprogression from true progression after standard treatment for GBM. Methods Seventy-sevenGBM patients, including 51 with true progression and 26 with pseudoprogression,who underwent standard treatment and T 1 CE, were retrospectively enrolled.Clinical information, including sex, age, KPS score, resection extent, neurological deficit and mean radiation dose, were also recorded collected for each patient. The whole tumor enhancementwas manually drawn on the T 1 CE image, and a total of texture 9675 features were extracted and fed to a two-step feature selection scheme. A random forest (RF) classifier was trained to separate the patients by their outcomes.The diagnostic efficacies of the radiomics modeland radiologist assessment were further compared by using theaccuracy (ACC), sensitivity and specificity. Results No clinical features showed statistically significant differences between true progression and pseudoprogression.The radiomic classifier demonstrated ACC, sensitivity, and specificity of 72.78%(95% confidence interval [CI]: 0.45,0.91), 78.36%(95%CI: 0.56,1.00) and 61.33%(95%CI: 0.20,0.82).The accuracy, sensitivity and specificity of three radiologists’ assessment were66.23%(95% CI: 0.55,0.76), 61.50%(95% CI: 0.43,0.78) and 68.62%(95% CI: 0.55,0.80); 55.84%(95% CI: 0.45,0.66),69.25%(95% CI: 0.50,0.84) and 49.13%(95% CI: 0.36,0.62); 55.84%(95% CI: 0.45,0.66), 69.23%(95% CI: 0.50,0.84) and 47.06%(95% CI: 0.34,0.61), respectively. Conclusion T 1 CE–based radiomics showed better classification performance compared with radiologists’ assessment.The radiomics modelwas promising in differentiating pseudoprogression from true progression.
更多查看译文
关键词
Glioblastoma, MRI, Pseudoprogression, Radiomics, Texture feature, Machine learning
AI 理解论文
溯源树
样例
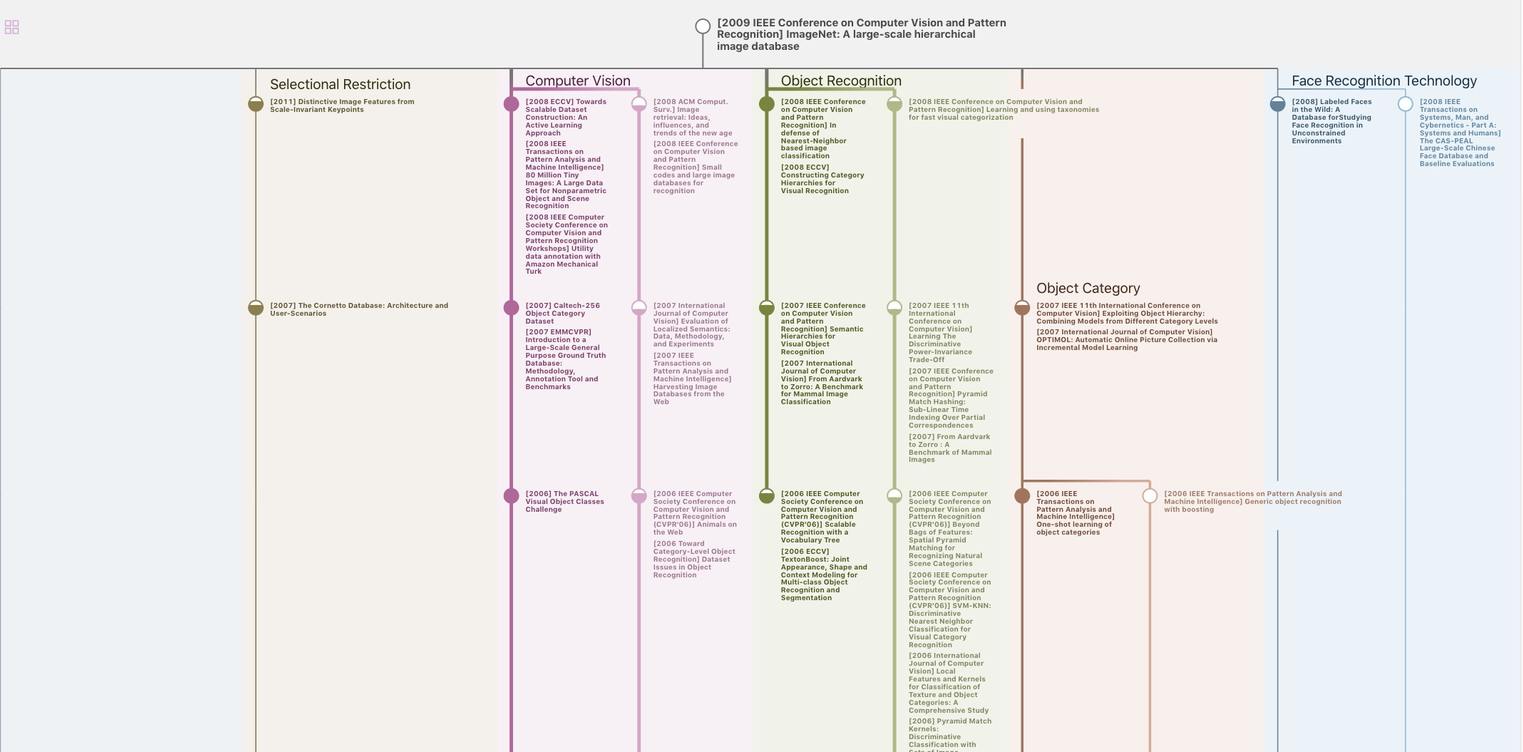
生成溯源树,研究论文发展脉络
Chat Paper
正在生成论文摘要