Typing Errors in Factual Knowledge Graphs: Severity and Possible Ways Out
International World Wide Web Conference(2021)
摘要
ABSTRACT Large-scale factual knowledge graphs (KGs) such as DBpedia and Wikidata are essential to many popular downstream tasks and are also widely used by various research communities as training and/or benchmarking data. Despite their immense success and utility, these KGs are surprisingly noisy. In this study, we investigate the quality of these KGs, where the typing error rate is estimated to be 27% for coarse-grained types on average, and even 73% for certain fine-grained types. In pursuit of solutions, we propose an active typing error detection algorithm that maximizes the utilization of both gold and noisy labels. We also comprehensively discuss and compare the state-of-the-art in unsupervised, semi-supervised, and supervised paradigms to deal with typing errors in factual KGs. The outcomes of this study provide guidelines for researchers to use noisy factual KGs. To help practitioners deploy the techniques and conduct further research, we published our code and data 1.
更多查看译文
关键词
label noise, data cleaning, noise model, learning with noise, factual knowledge graph
AI 理解论文
溯源树
样例
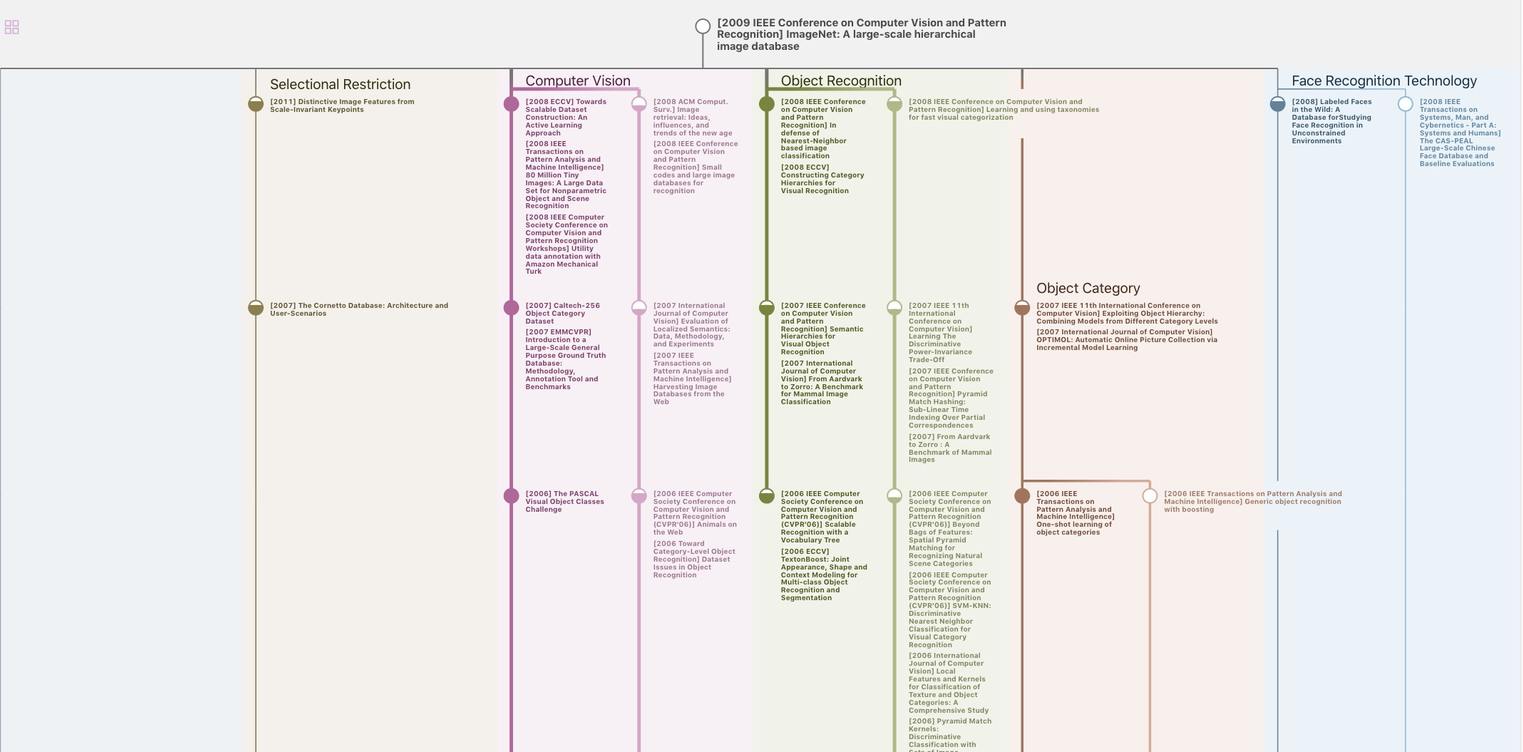
生成溯源树,研究论文发展脉络
Chat Paper
正在生成论文摘要