Classification Using Fractional Anisotropy Predicts Conversion in Genetic Frontotemporal Dementia, a Proof of Concept
Brain communications(2020)
摘要
Frontotemporal dementia is a highly heritable and devastating neurodegenerative disease. About 10-20% of all frontotemporal dementia is caused by known pathogenic mutations, but a reliable tool to predict clinical conversion in mutation carriers is lacking. In this retrospective proof-of-concept case-control study, we investigate whether MRI-based and cognition-based classifiers can predict which mutation carriers from genetic frontotemporal dementia families will develop symptoms ('convert') within 4 years. From genetic frontotemporal dementia families, we included 42 presymptomatic frontotemporal dementia mutation carriers. We acquired anatomical, diffusion-weighted imaging, and resting-state functional MRI, as well as neuropsychological data. After 4 years, seven mutation carriers had converted to frontotemporal dementia ('converters'), while 35 had not ('non-converters'). We trained regularized logistic regression models on baseline MRI and cognitive data to predict conversion to frontotemporal dementia within 4 years, and quantified prediction performance using area under the receiver operating characteristic curves. The prediction model based on fractional anisotropy, with highest contribution of the forceps minor, predicted conversion to frontotemporal dementia beyond chance level (0.81 area under the curve, family-wise error corrected P = 0.025 versus chance level). Other MRI-based and cognitive features did not outperform chance level. Even in a small sample, fractional anisotropy predicted conversion in presymptomatic frontotemporal dementia mutation carriers beyond chance level. After validation in larger data sets, conversion prediction in genetic frontotemporal dementia may facilitate early recruitment into clinical trials.
更多查看译文
关键词
frontotemporal dementia,MAPT protein,human,GRN protein,human,multimodal MRI,classification
AI 理解论文
溯源树
样例
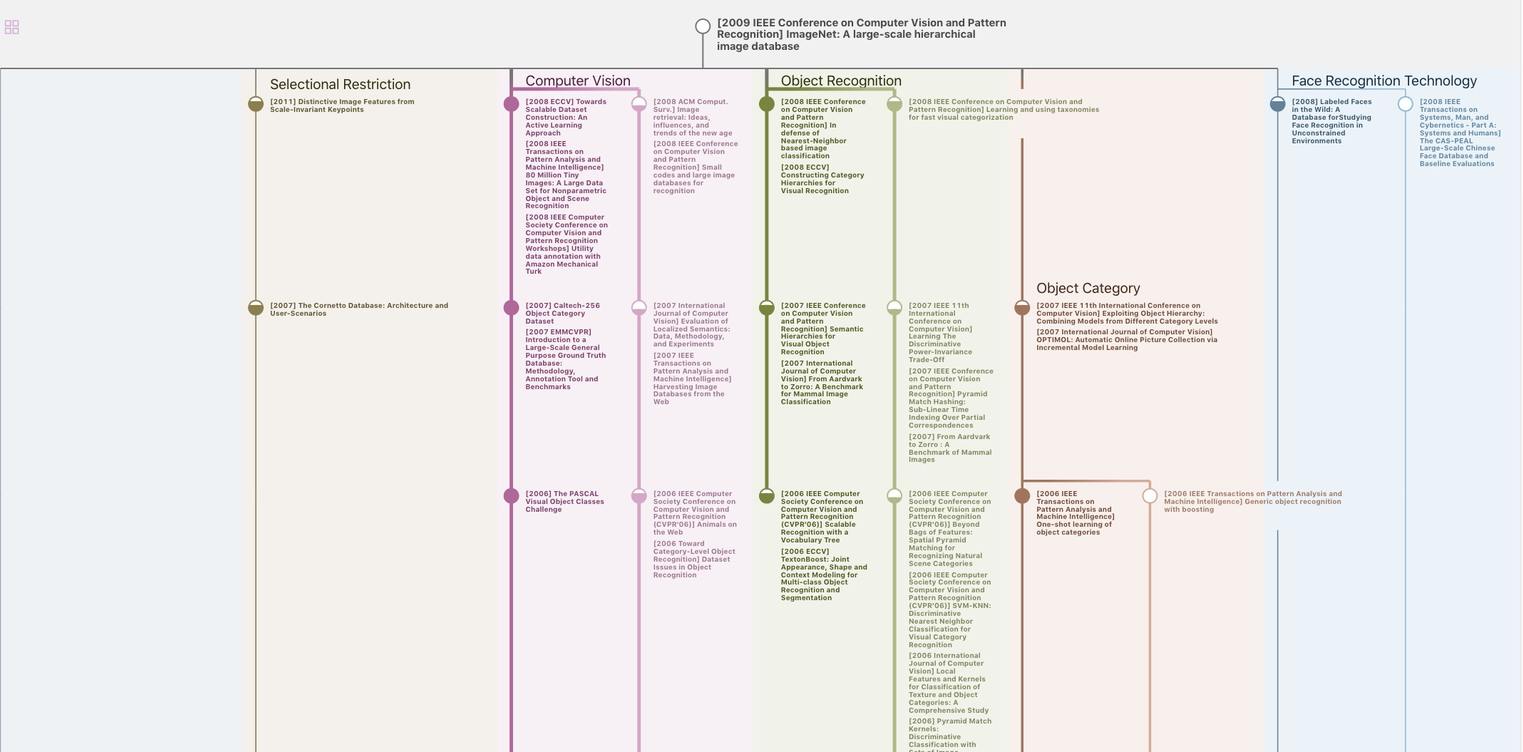
生成溯源树,研究论文发展脉络
Chat Paper
正在生成论文摘要