ACT: an Attentive Convolutional Transformer for Efficient Text Classification.
AAAI(2021)
Abstract
Recently, Transformer has been demonstrating promising performance in many NLP tasks and showing a trend of replacing Recurrent Neural Network (RNN). Meanwhile, less attention is drawn to Convolutional Neural Network (CNN) due to its weak ability in capturing sequential and long-distance dependencies, although it has excellent local feature extraction capability. In this paper, we introduce an Attentive Convolutional Transformer (ACT) that takes the advantages of both Transformer and CNN for efficient text classification. Specifically, we propose a novel attentive convolution mechanism that utilizes the semantic meaning of convolutional filters attentively to transform text from complex word space to a more informative convolutional filter space where important n-grams are captured. ACT is able to capture both local and global dependencies effectively while preserving sequential information. Experiments on various text classification tasks and detailed analyses show that ACT is a lightweight, fast, and effective universal text classifier, outperforming CNNs, RNNs, and attentive models including Transformer.
MoreTranslated text
Key words
attentive convolutional transformer,classification,text
AI Read Science
Must-Reading Tree
Example
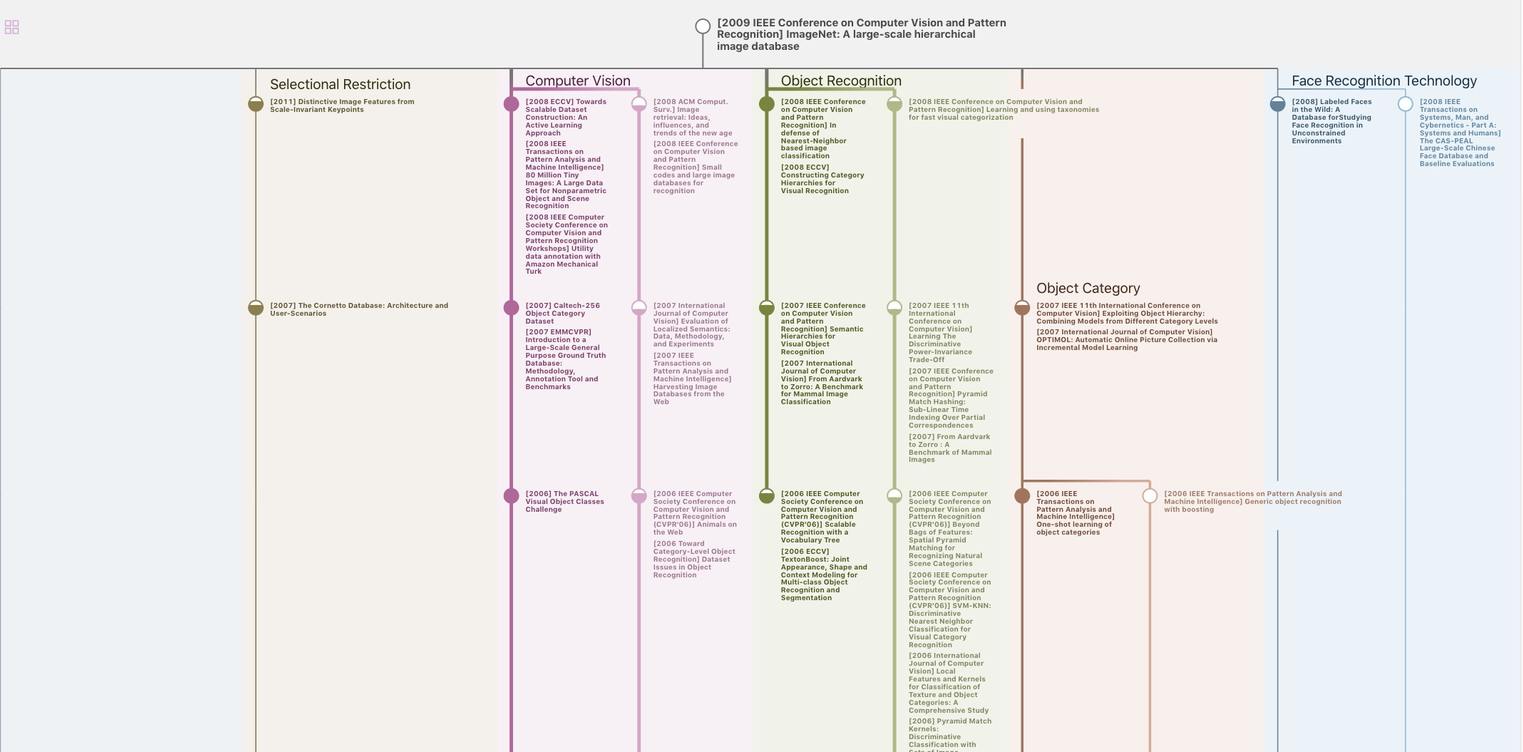
Generate MRT to find the research sequence of this paper
Chat Paper
Summary is being generated by the instructions you defined