Knowledge Refinery: Learning from Decoupled Label
Proceedings of the AAAI Conference on Artificial Intelligence(2021)
摘要
Recently, a variety of regularization techniques have been widely applied in deep neural networks, which mainly focus on the regularization of weight parameters to encourage generalization effectively. Label regularization techniques are also proposed with the motivation of softening the labels while neglecting the relation of classes. Among them, the technique of knowledge distillation proposes to distill the soft label, which contains the knowledge of class relations. However, this technique needs to pre-train an extra cumbersome teacher model. In this paper, we propose a method called Knowledge Refinery (KR), which enables the neural network to learn the relation of classes on-the-fly without the teacher-student training strategy. We propose the definition of decoupled labels, which consist of the original hard label and the residual label. To exhibit the generalization of KR, we evaluate our method in both fields of computer vision and natural language processing. Our empirical results show consistent performance gains under all experimental settings.
更多查看译文
AI 理解论文
溯源树
样例
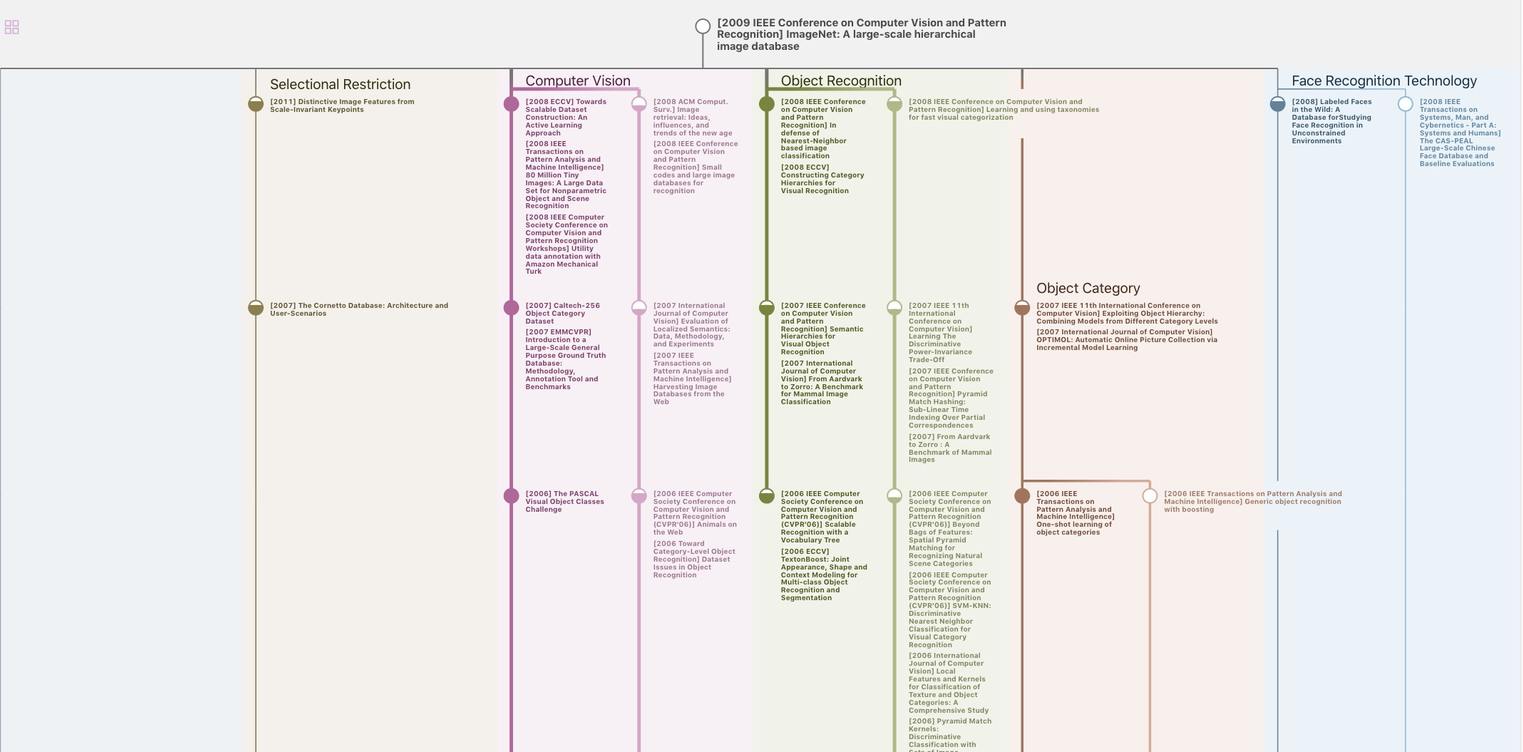
生成溯源树,研究论文发展脉络
Chat Paper
正在生成论文摘要