Bias-Variance Reduced Local SGD for Less Heterogeneous Federated Learning
INTERNATIONAL CONFERENCE ON MACHINE LEARNING, VOL 139(2021)
摘要
Recently, local SGD has got much attention and been extensively studied in the distributed learning community to overcome the communication bottleneck problem. However, the superiority of local SGD to minibatch SGD only holds in quite limited situations. In this paper, we study a new local algorithm called Bias-Variance Reduced Local SGD (BVR-L-SGD) for nonconvex distributed optimization. Algorithmically, our proposed bias and variance reduced local gradient estimator fully utilizes small second-order heterogeneity of local objectives and suggests randomly picking up one of the local models instead of taking the average of them when workers are synchronized. Theoretically, under small heterogeneity of local objectives, we show that BVR-L-SGD achieves better communication complexity than both the previous non-local and local methods under mild conditions, and particularly BVR-L-SGD is the first method that breaks the barrier of communication complexity Theta(1/epsilon) for general nonconvex smooth objectives when the heterogeneity is small and the local computation budget is large. Numerical results are given to verify the theoretical findings and give empirical evidence of the superiority of our method.
更多查看译文
关键词
heterogeneous federated learning,bias-variance
AI 理解论文
溯源树
样例
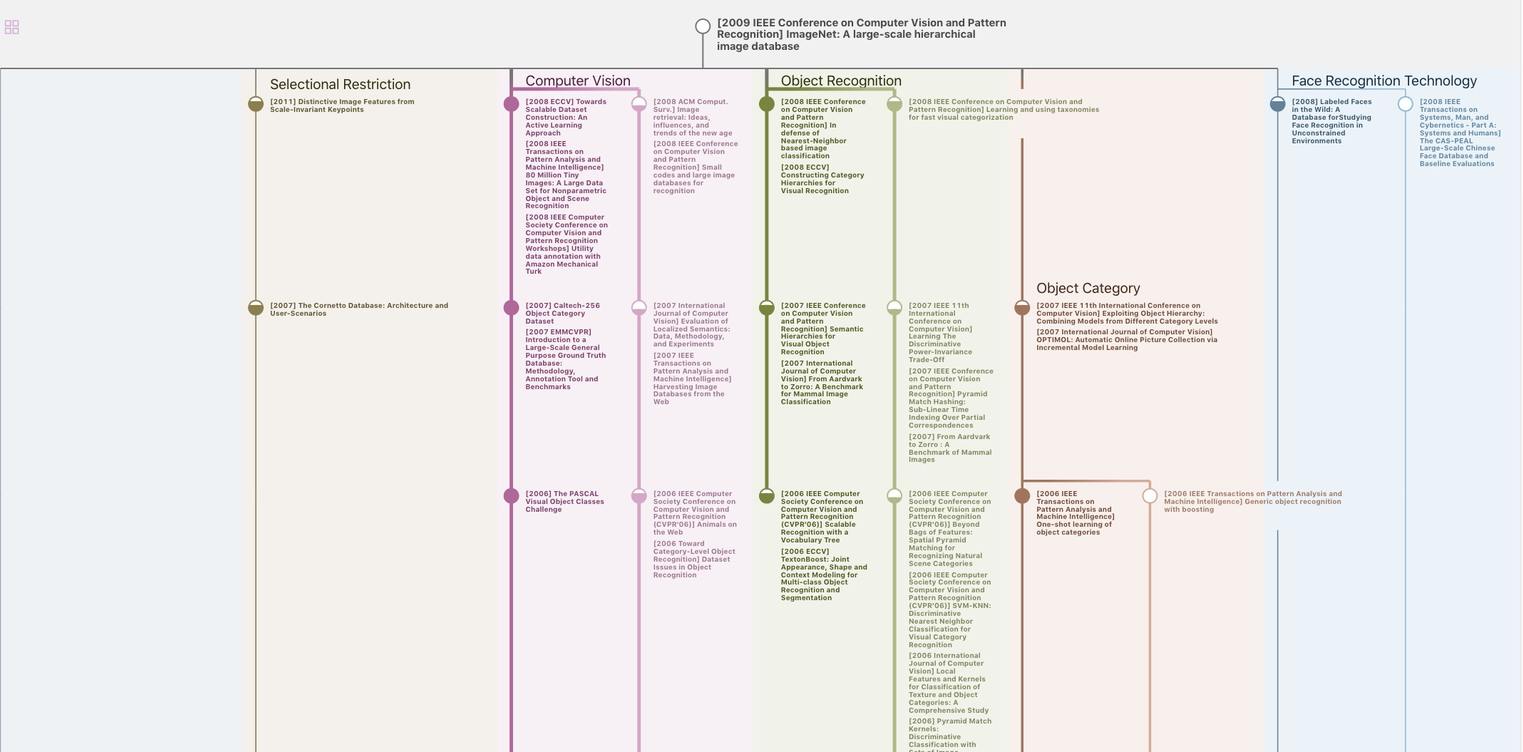
生成溯源树,研究论文发展脉络
Chat Paper
正在生成论文摘要