NRTSI: Non-Recurrent Time Series Imputation for Irregularly-sampled Data
arxiv(2021)
摘要
Time series imputation is a fundamental task for understanding time series with missing data. Existing imputation methods often rely on recurrent models such as RNNs and ordinary differential equations, both of which suffer from the error compounding problems of recurrent models. In this work, we view the imputation task from the perspective of permutation equivariant modeling of sets and propose a novel imputation model called NRTSI without any recurrent modules. Taking advantage of the permutation equivariant nature of NRTSI, we design a principled and efficient hierarchical imputation procedure. NRTSI can easily handle irregularly-sampled data, perform multiple-mode stochastic imputation, and handle the scenario where dimensions are partially observed. We show that NRTSI achieves state-of-the-art performance across a wide range of commonly used time series imputation benchmarks.
更多查看译文
关键词
data,nrtsi,non-recurrent,irregularly-sampled
AI 理解论文
溯源树
样例
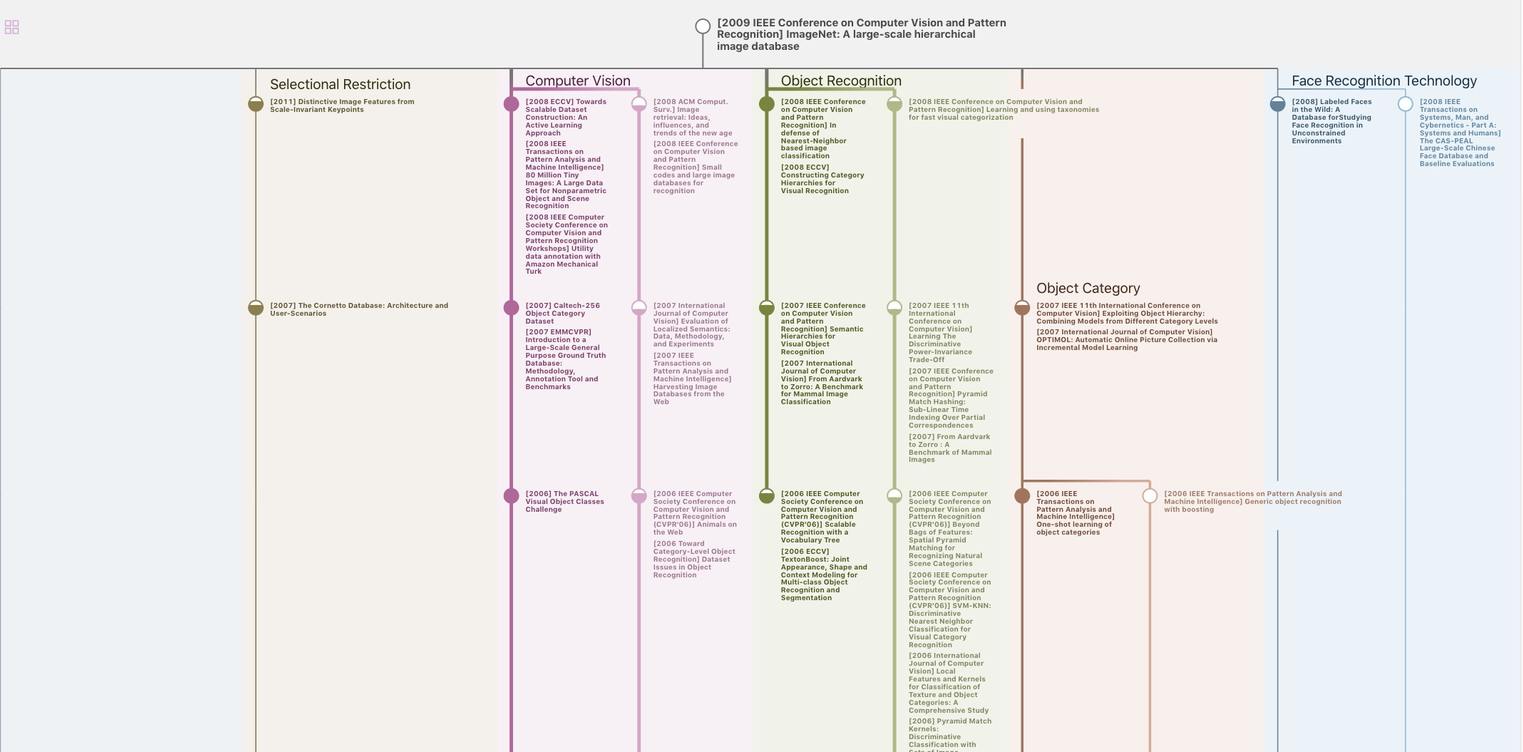
生成溯源树,研究论文发展脉络
Chat Paper
正在生成论文摘要