Exploring the Limits of Few-Shot Link Prediction in Knowledge Graphs
EACL(2021)
摘要
Real-world knowledge graphs are often characterized by low-frequency relations - a challenge that has prompted an increasing interest in few-shot link prediction methods. These methods perform link prediction for a set of new relations, unseen during training, given only a few example facts of each relation at test time. In this work, we perform a systematic study on a spectrum of models derived by generalizing the current state of the art for few-shot link prediction, with the goal of probing the limits of learning in this few-shot setting. We find that a simple zero-shot baseline - which ignores any relation-specific information - achieves surprisingly strong performance. Moreover, experiments on carefully crafted synthetic datasets show that having only a few examples of a relation fundamentally limits models from using fine-grained structural information and only allows for exploiting the coarse-grained positional information of entities. Together, our findings challenge the implicit assumptions and inductive biases of prior work and highlight new directions for research in this area.
更多查看译文
关键词
knowledge,prediction,graphs,few-shot
AI 理解论文
溯源树
样例
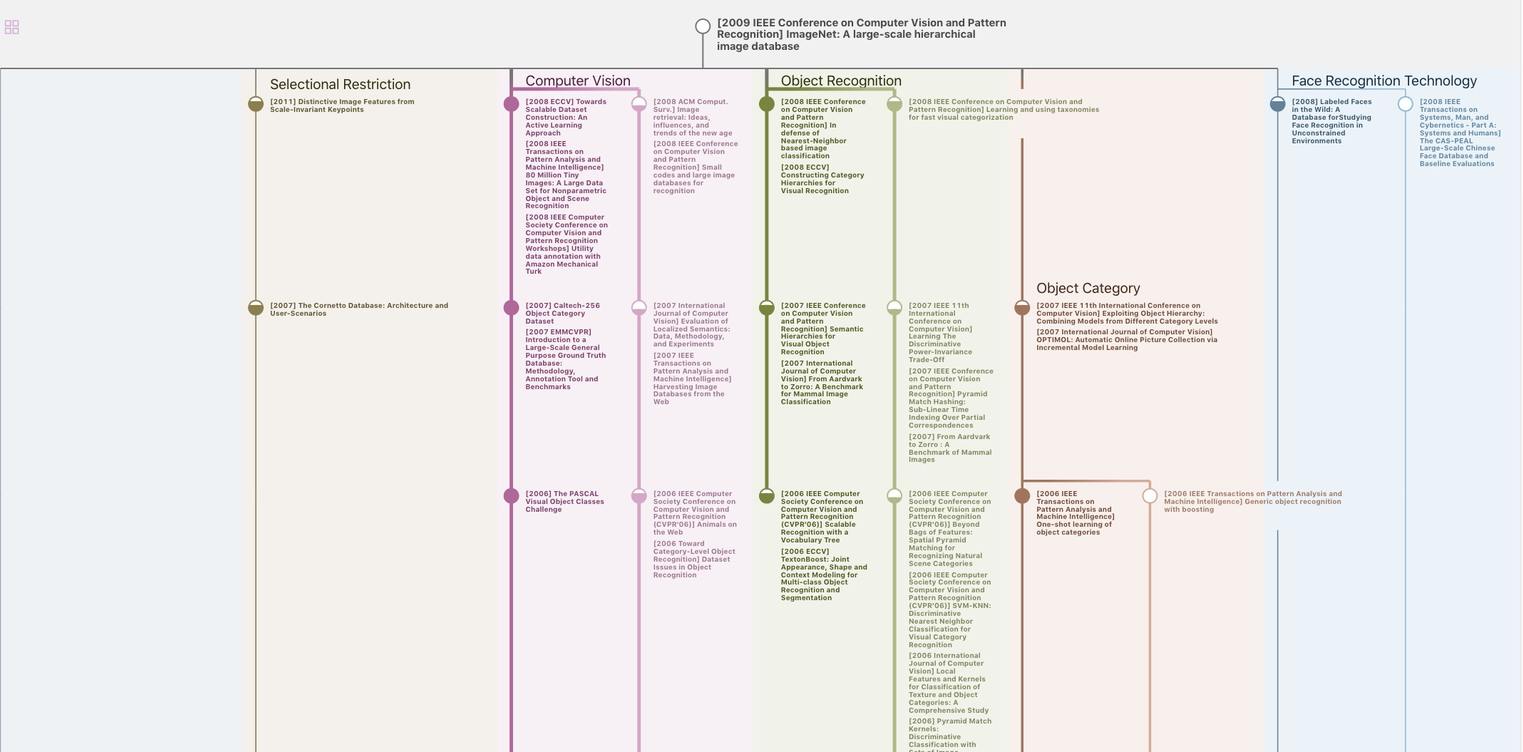
生成溯源树,研究论文发展脉络
Chat Paper
正在生成论文摘要