Oops I Took A Gradient: Scalable Sampling For Discrete Distributions
INTERNATIONAL CONFERENCE ON MACHINE LEARNING, VOL 139(2021)
摘要
We propose a general and scalable approximate sampling strategy for probabilistic models with discrete variables. Our approach uses gradients of the likelihood function with respect to its discrete inputs to propose updates in a Metropolis-Hastings sampler. We show empirically that this approach outperforms generic samplers in a number of difficult settings including Ising models, Potts models, restricted Boltzmann machines, and factorial hidden Markov models. We also demon-strate the use of our improved sampler for training deep energy-based models (EBM) on high dimensional discrete data. This approach outperforms variational auto-encoders and existing energy-based models. Finally, we give bounds showing that our approach is near-optimal in the class of samplers which propose local updates.
更多查看译文
关键词
scalable sampling,gradient
AI 理解论文
溯源树
样例
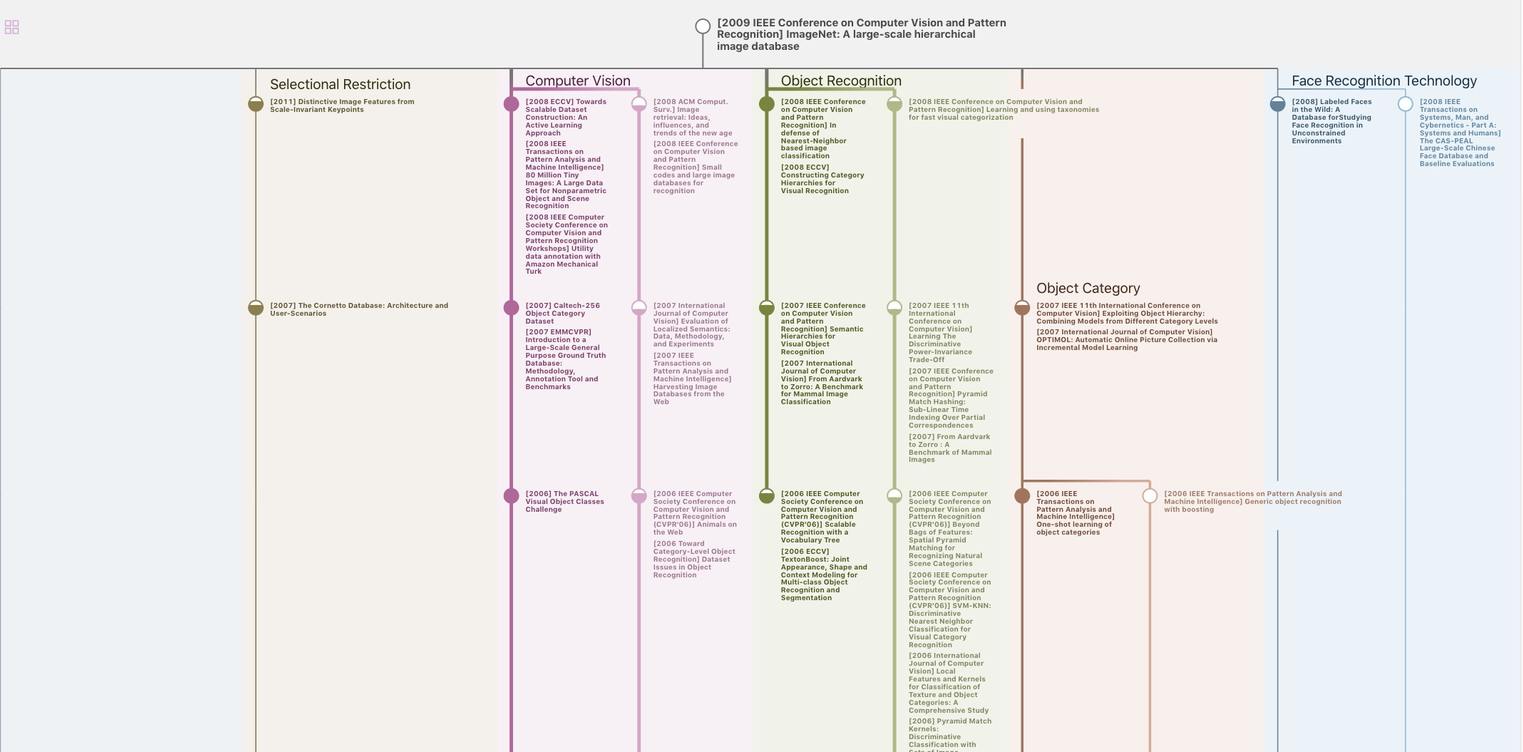
生成溯源树,研究论文发展脉络
Chat Paper
正在生成论文摘要