Comparisons Of Polyexposure, Polygenic, And Clinical Risk Scores In Risk Prediction Of Type 2 Diabetes
DIABETES CARE(2021)
摘要
OBJECTIVE To establish a polyexposure score (PXS) for type 2 diabetes (T2D) incorporating 12 nongenetic exposures and examine whether a PXS and/or a polygenic risk score (PGS) improves diabetes prediction beyond traditional clinical risk factors. RESEARCH DESIGN AND METHODS We identified 356,621 unrelated individuals from the UK Biobank of White British ancestry with no prior diagnosis of T2D and normal HbA(1c) levels. Using self-reported and hospital admission information, we deployed a machine learning procedure to select the most predictive and robust factors out of 111 nongenetically ascertained exposure and lifestyle variables for the PXS in prospective T2D. We computed the clinical risk score (CRS) and PGS by taking a weighted sum of eight established clinical risk factors and >6 million single nucleotide polymorphisms, respectively. RESULTS In the study population, 7,513 had incident T2D. The C-statistics for the PGS, PXS, and CRS models were 0.709, 0.762, and 0.839, respectively. Individuals in the top 10% of PGS, PXS, and CRS had 2.00-, 5.90-, and 9.97-fold greater risk, respectively, compared to the remaining population. Addition of PGS and PXS to CRS improved T2D classification accuracy, with a continuous net reclassification index of 15.2% and 30.1% for cases, respectively, and 7.3% and 16.9% for controls, respectively. CONCLUSIONS For T2D, the PXS provides modest incremental predictive value over established clinical risk factors. However, the concept of PXS merits further consideration in T2D risk stratification and is likely to be useful in other chronic disease risk prediction models.
更多查看译文
关键词
clinical risk scores,polygenic,polyexposure,diabetes,risk prediction
AI 理解论文
溯源树
样例
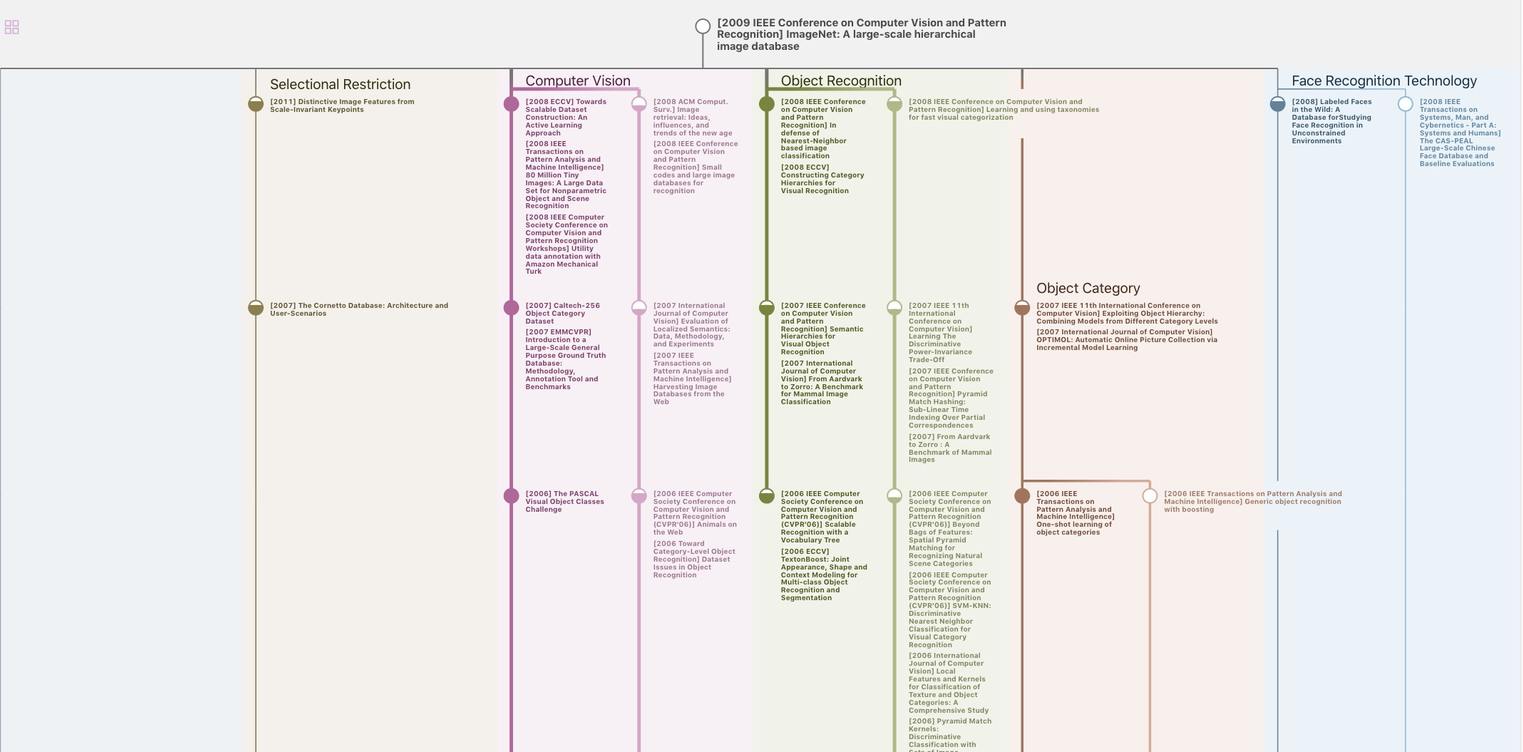
生成溯源树,研究论文发展脉络
Chat Paper
正在生成论文摘要