A Deep Learning Approach for Characterizing Major Galaxy Mergers
arxiv(2021)
摘要
Fine-grained estimation of galaxy merger stages from observations is a key problem useful for validation of our current theoretical understanding of galaxy formation. To this end, we demonstrate a CNN-based regression model that is able to predict, for the first time, using a single image, the merger stage relative to the first perigee passage with a median error of 38.3 million years (Myrs) over a period of 400 Myrs. This model uses no specific dynamical modeling and learns only from simulated merger events. We show that our model provides reasonable estimates on real observations, approximately matching prior estimates provided by detailed dynamical modeling. We provide a preliminary interpretability analysis of our models, and demonstrate first steps toward calibrated uncertainty estimation.
更多查看译文
关键词
major galaxy mergers,deep learning,deep learning approach
AI 理解论文
溯源树
样例
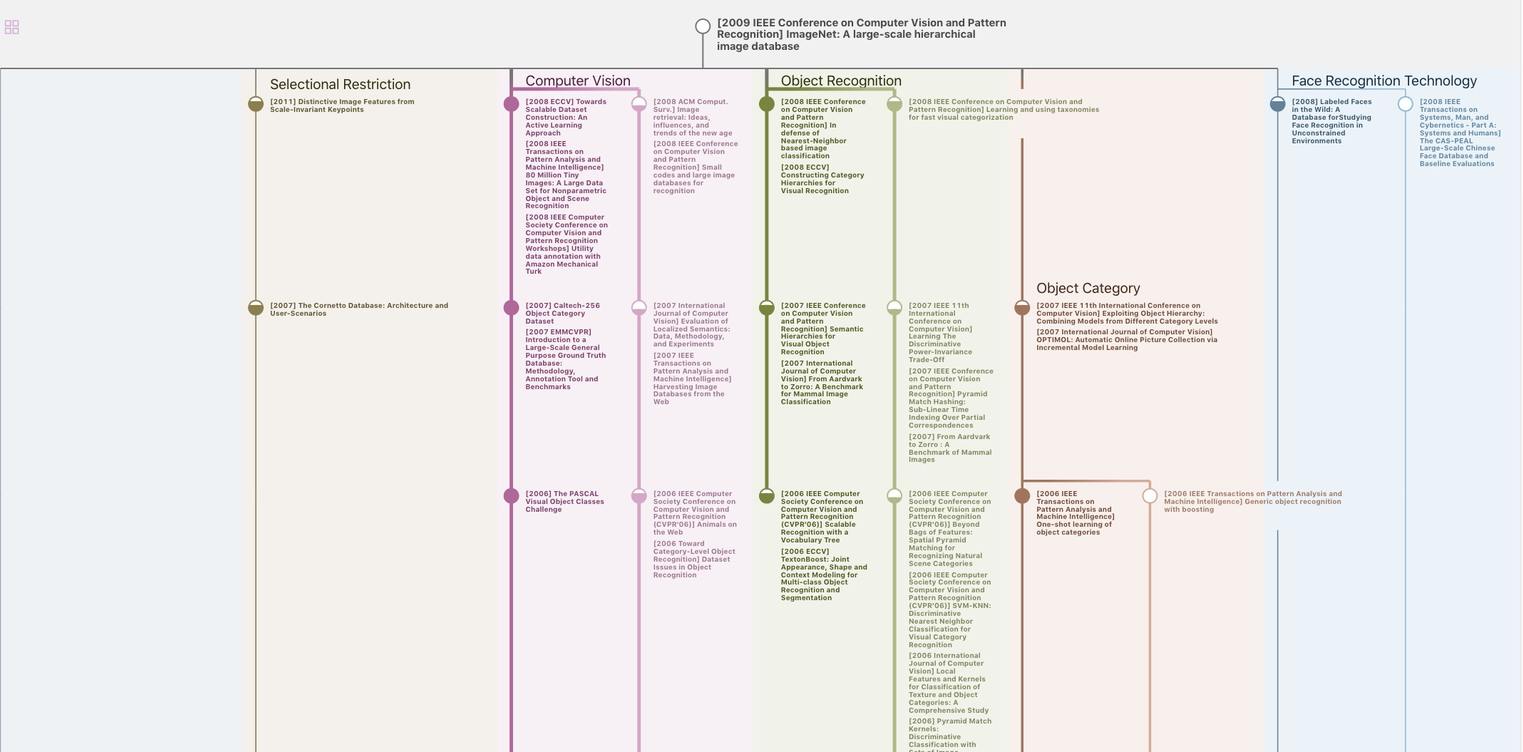
生成溯源树,研究论文发展脉络
Chat Paper
正在生成论文摘要