A General Framework for Revealing Human Mind with auto-encoding GANs
arxiv(2021)
摘要
Addressing the question of visualising human mind could help us to find regions that are associated with observed cognition and responsible for expressing the elusive mental image, leading to a better understanding of cognitive function. The traditional approach treats brain decoding as a classification problem, reading the mind through statistical analysis of brain activity. However, human thought is rich and varied, that it is often influenced by more of a combination of object features than a specific type of category. For this reason, we propose an end-to-end brain decoding framework which translates brain activity into an image by latent space alignment. To find the correspondence from brain signal features to image features, we embedded them into two latent spaces with modality-specific encoders and then aligned the two spaces by minimising the distance between paired latent representations. The proposed framework was trained by simultaneous electroencephalogram and functional MRI data, which were recorded when the subjects were viewing or imagining a set of image stimuli. In this paper, we focused on implementing the fMRI experiment. Our experimental results demonstrated the feasibility of translating brain activity to an image. The reconstructed image matches image stimuli approximate in both shape and colour. Our framework provides a promising direction for building a direct visualisation to reveal human mind.
更多查看译文
AI 理解论文
溯源树
样例
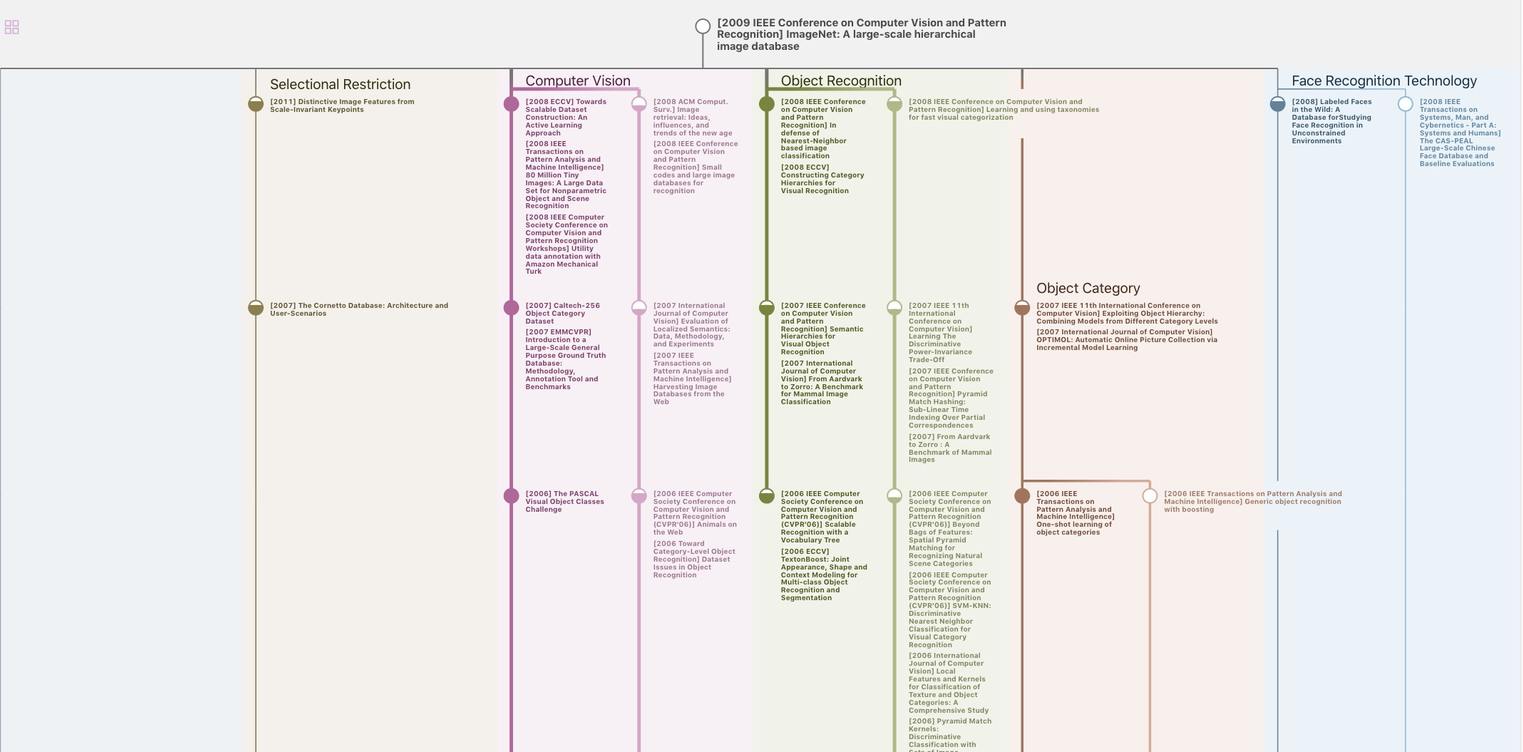
生成溯源树,研究论文发展脉络
Chat Paper
正在生成论文摘要