SPARSE GRAPH BASED SKETCHING FOR FAST NUMERICAL LINEAR ALGEBRA
2021 IEEE INTERNATIONAL CONFERENCE ON ACOUSTICS, SPEECH AND SIGNAL PROCESSING (ICASSP 2021)(2021)
摘要
In recent years, a variety of randomized constructions of sketching matrices have been devised, that have been used in fast algorithms for numerical linear algebra problems, such as least squares regression, low-rank approximation, and the approximation of leverage scores. A key property of sketching matrices is that of subspace embedding. In this paper, we study sketching matrices that are obtained from bipartite graphs that are sparse, i.e., have left degree s that is small. In particular, we explore two popular classes of sparse graphs, namely, expander graphs and magical graphs. For a given subspace U subset of R-n of dimension k, we show that the magical graph with left degree s = 2 yields a (1 +/- epsilon) l(2)-subspace embedding for U, if the number of right vertices (the sketch size) m = O(k(2)/epsilon(2)). The expander graph with s = O (log k/epsilon) yields a subspace embedding for m = O (k log k/epsilon(2)). We also discuss the construction of sparse sketching matrices with reduced randomness using expanders based on error-correcting codes. Empirical results on various synthetic and real datasets show that these sparse graph sketching matrices work very well in practice.
更多查看译文
关键词
Randomized sketching, expander graphs, low-rank approximation, least squares regression
AI 理解论文
溯源树
样例
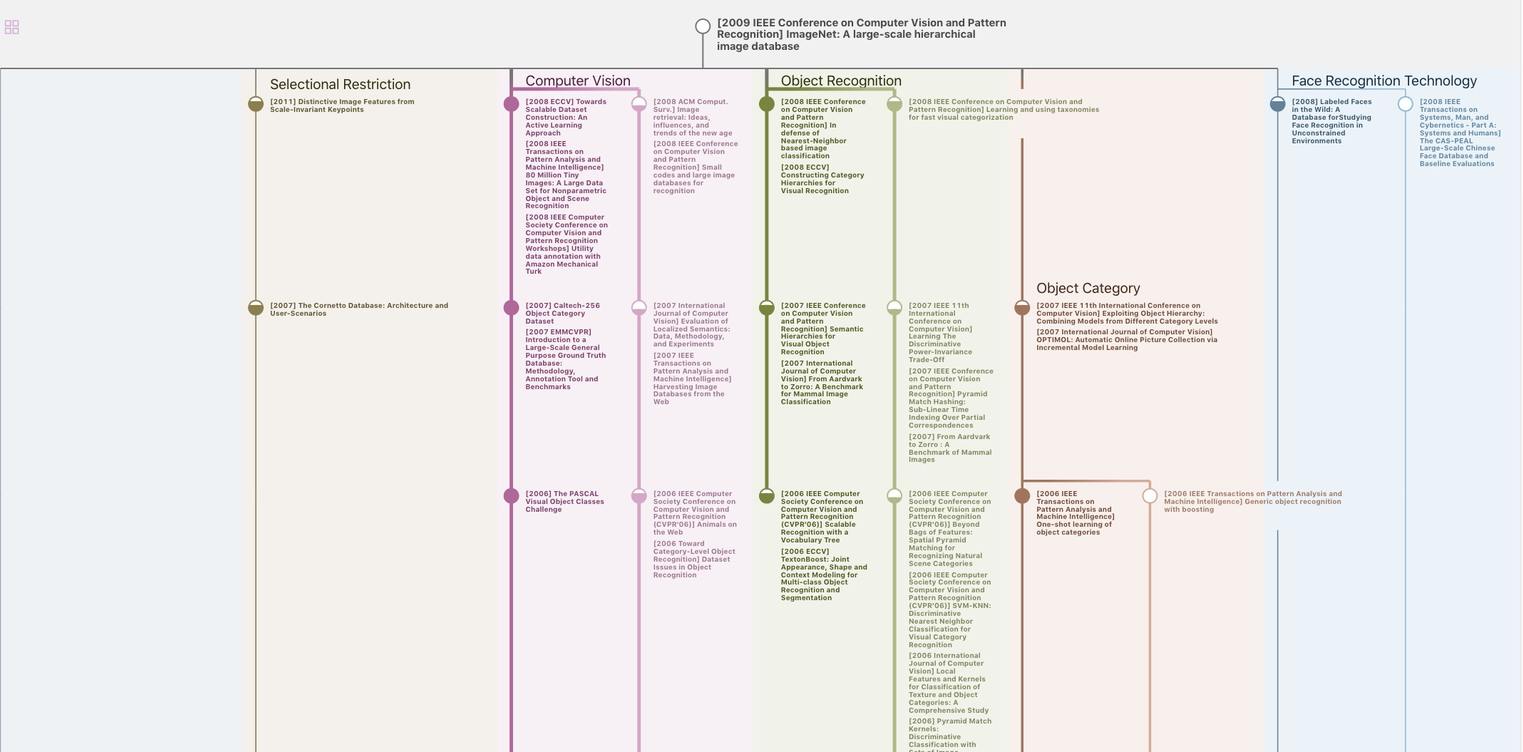
生成溯源树,研究论文发展脉络
Chat Paper
正在生成论文摘要