Sequential Sentence Classification in Research Papers using Cross-Domain Multi-Task Learning
arxiv(2021)
摘要
The task of sequential sentence classification enables the semantic structuring of research papers. This can enhance academic search engines to support researchers in finding and exploring research literature more effectively. However, previous work has not investigated the potential of transfer learning with datasets from different scientific domains for this task yet. We propose a uniform deep learning architecture and multi-task learning to improve sequential sentence classification in scientific texts across domains by exploiting training data from multiple domains. Our contributions can be summarised as follows: (1) We tailor two common transfer learning methods, sequential transfer learning and multi-task learning, and evaluate their performance for sequential sentence classification; (2) The presented multi-task model is able to recognise semantically related classes from different datasets and thus supports manual comparison and assessment of different annotation schemes; (3) The unified approach is capable of handling datasets that contain either only abstracts or full papers without further feature engineering. We demonstrate that models, which are trained on datasets from different scientific domains, benefit from one another when using the proposed multi-task learning architecture. Our approach outperforms the state of the art on three benchmark datasets.
更多查看译文
关键词
sequential sentence classification,zone identification,transfer learning,multi-task learning,scholarly communication
AI 理解论文
溯源树
样例
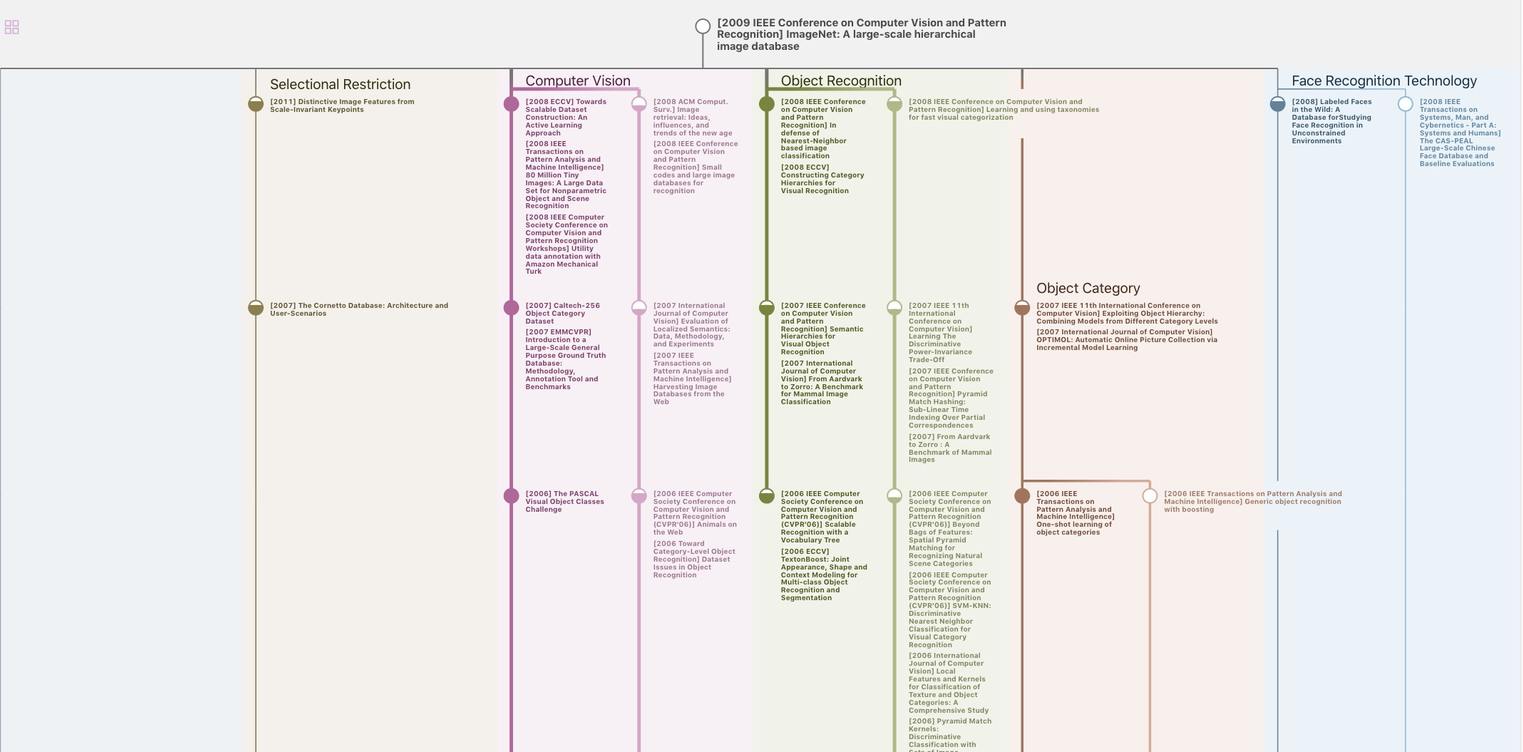
生成溯源树,研究论文发展脉络
Chat Paper
正在生成论文摘要