Improving Scalable K-Means++
ALGORITHMS(2021)
摘要
Two new initialization methods for K-means clustering are proposed. Both proposals are based on applying a divide-and-conquer approach for the K-means|| type of an initialization strategy. The second proposal also uses multiple lower-dimensional subspaces produced by the random projection method for the initialization. The proposed methods are scalable and can be run in parallel, which make them suitable for initializing large-scale problems. In the experiments, comparison of the proposed methods to the K-means++ and K-means|| methods is conducted using an extensive set of reference and synthetic large-scale datasets. Concerning the latter, a novel high-dimensional clustering data generation algorithm is given. The experiments show that the proposed methods compare favorably to the state-of-the-art by improving clustering accuracy and the speed of convergence. We also observe that the currently most popular K-means++ initialization behaves like the random one in the very high-dimensional cases.
更多查看译文
关键词
clustering initialization, K-means‖, K-means++, random projection
AI 理解论文
溯源树
样例
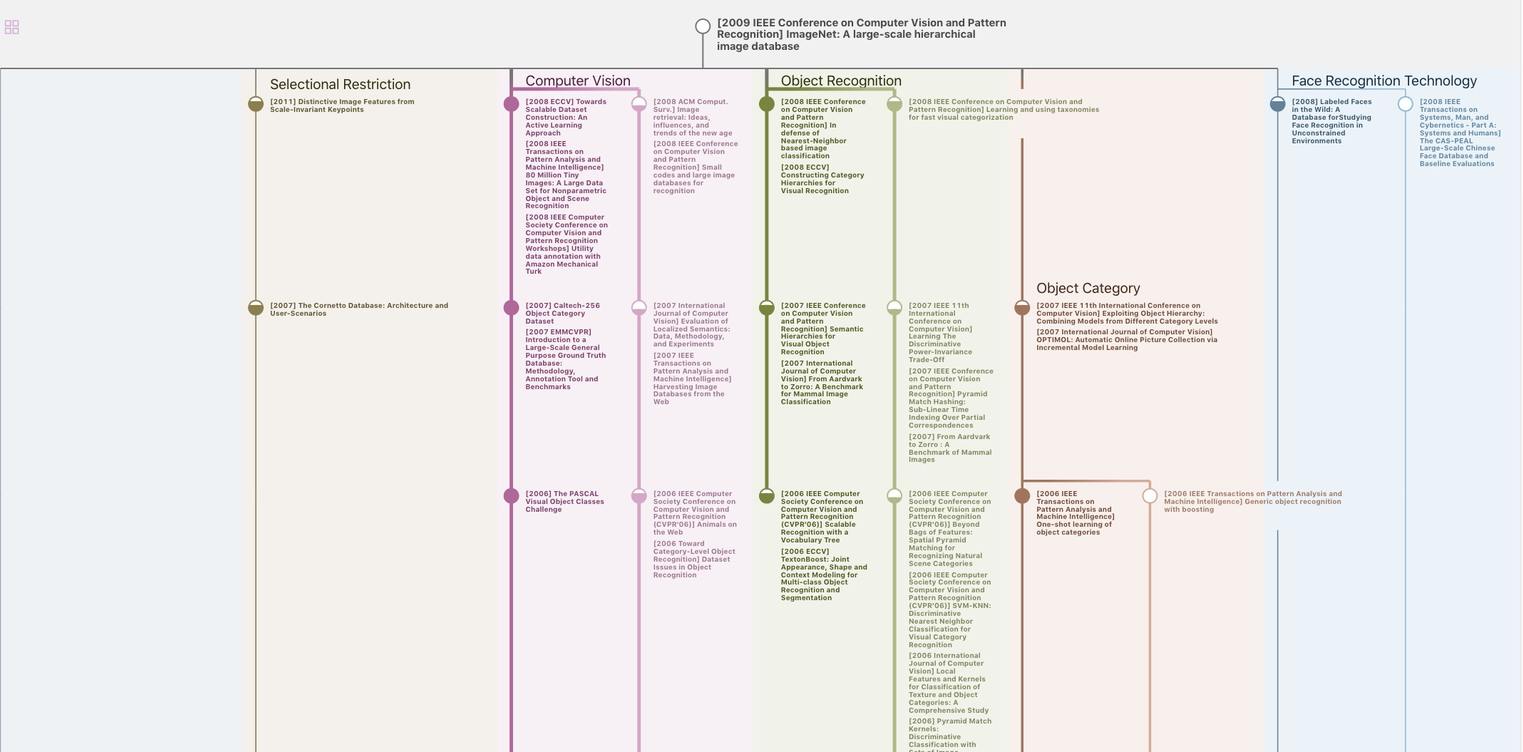
生成溯源树,研究论文发展脉络
Chat Paper
正在生成论文摘要