Exam: A Framework Of Learning Extreme And Moderate Embeddings For Person Re-Id
JOURNAL OF IMAGING(2021)
摘要
Person re-identification (Re-ID) is challenging due to host of factors: the variety of human positions, difficulties in aligning bounding boxes, and complex backgrounds, among other factors. This paper proposes a new framework called EXAM (EXtreme And Moderate feature embeddings) for Re-ID tasks. This is done using discriminative feature learning, requiring attention-based guidance during training. Here "Extreme" refers to salient human features and "Moderate" refers to common human features. In this framework, these types of embeddings are calculated by global max-pooling and average-pooling operations respectively; and then, jointly supervised by multiple triplet and cross-entropy loss functions. The processes of deducing attention from learned embeddings and discriminative feature learning are incorporated, and benefit from each other in this end-to-end framework. From the comparative experiments and ablation studies, it is shown that the proposed EXAM is effective, and its learned feature representation reaches state-of-the-art performance.
更多查看译文
关键词
person Re-ID, deep learning, loss function
AI 理解论文
溯源树
样例
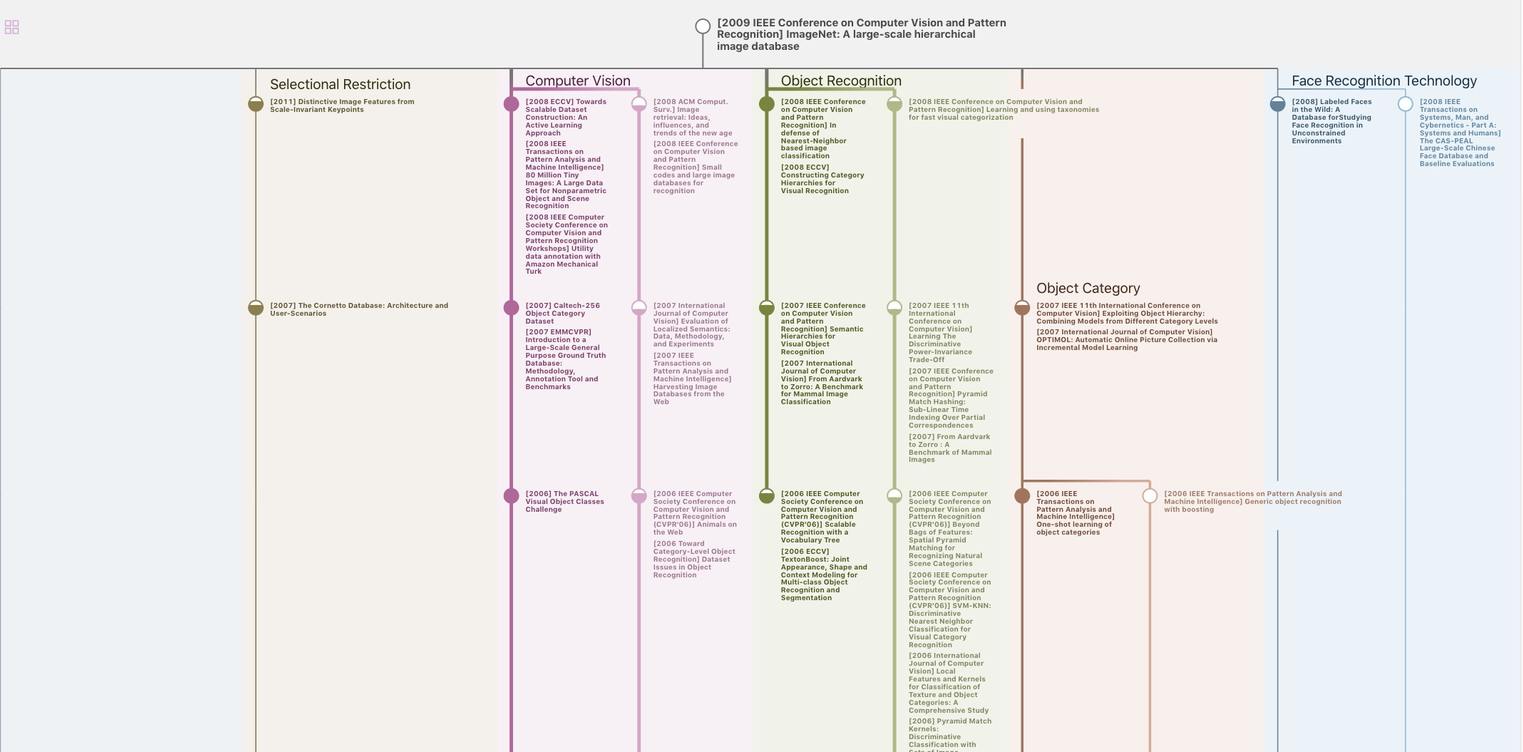
生成溯源树,研究论文发展脉络
Chat Paper
正在生成论文摘要