Temperature Prediction Based On Bidirectional Long Short-Term Memory And Convolutional Neural Network Combining Observed And Numerical Forecast Data
SENSORS(2021)
摘要
Weather is affected by a complex interplay of factors, including topography, location, and time. For the prediction of temperature in Korea, it is necessary to use data from multiple regions. To this end, we investigate the use of deep neural-network-based temperature prediction model time-series weather data obtained from an automatic weather station and image data from a regional data assimilation and prediction system (RDAPS). To accommodate such different types of data into a single model, a bidirectional long short-term memory (BLSTM) model and a convolutional neural network (CNN) model are chosen to represent the features from the time-series observed data and the RDAPS image data. The two types of features are combined to produce temperature predictions for up to 14 days in the future. The performance of the proposed temperature prediction model is evaluated by objective measures, including the root mean squared error and mean bias error. The experiments demonstrated that the proposed model combining both the observed and RDAPS image data is better in all performance measures for all prediction periods compared with the BLSTM-based model using observed data and the CNN-BLSTM-based model using RDAPS image data alone.
更多查看译文
关键词
temperature prediction, bidirectional long short-term memory (BLSTM), convolutional neural network (CNN), regional data assimilation and prediction system, automatic weather station, weather data
AI 理解论文
溯源树
样例
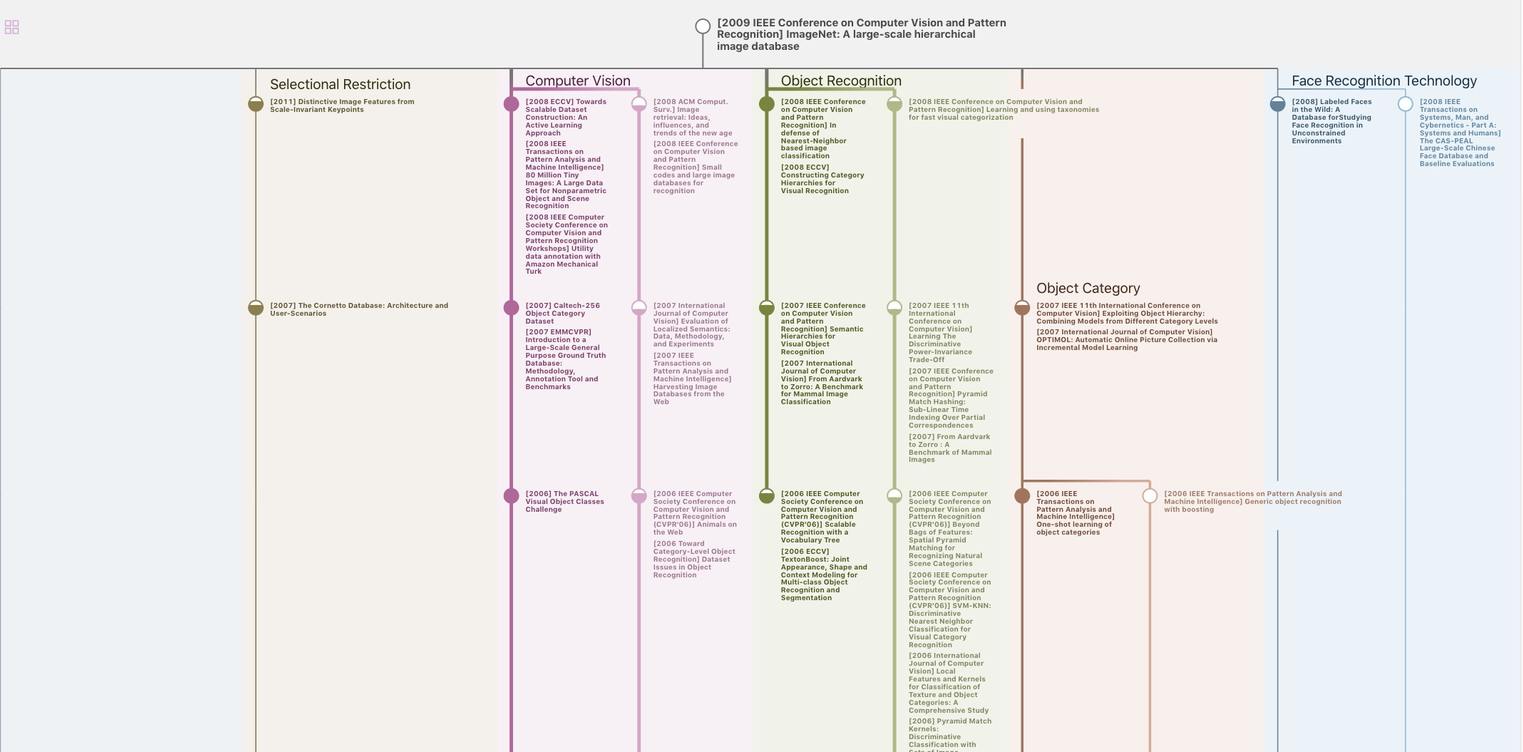
生成溯源树,研究论文发展脉络
Chat Paper
正在生成论文摘要