ReRankMatch: Semi-Supervised Learning with Semantics-Oriented Similarity Representation
2021 INTERNATIONAL JOINT CONFERENCE ON NEURAL NETWORKS (IJCNN)(2021)
摘要
This paper proposes integrating semantics-oriented similarity representation into RankingMatch, a recently proposed semi-supervised learning method. Our method, dubbed ReRankMatch, aims to deal with the case in which labeled and unlabeled data share non-overlapping categories. ReRankMatch encourages the model to produce the similar image representations for the samples likely belonging to the same class. We evaluate our method on various datasets such as CIFAR-10, CIFAR-100, SVHN, STL-10, and Tiny ImageNet. We obtain promising results (4.21% error rate on CIFAR-10 with 4000 labels, 22.32% error rate on CIFAR-100 with 10000 labels, and 2.19% error rate on SVHN with 1000 labels) when the amount of labeled data is sufficient to learn semantics-oriented similarity representation. The code is made publicly available at https://github.com/tqtrunghnvn/ReRankMatch.
更多查看译文
关键词
similarity
AI 理解论文
溯源树
样例
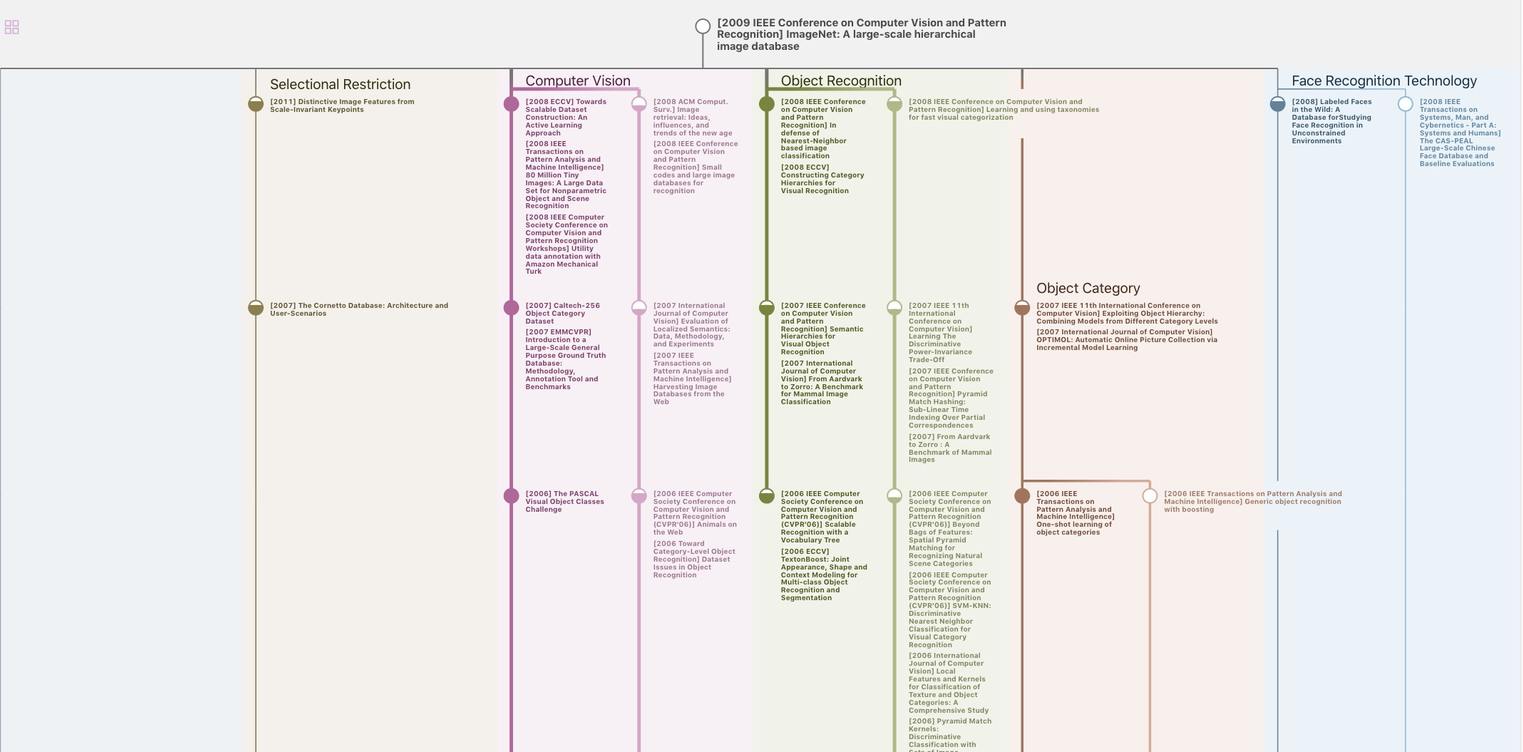
生成溯源树,研究论文发展脉络
Chat Paper
正在生成论文摘要