Improved Corruption Robust Algorithms For Episodic Reinforcement Learning
INTERNATIONAL CONFERENCE ON MACHINE LEARNING, VOL 139(2021)
摘要
We study episodic reinforcement learning under unknown adversarial corruptions in both the rewards and the transition probabilities of the underlying system. We propose new algorithms which, compared to the existing results in (Lykouris et al., 2020), achieve strictly better regret bounds in terms of total corruptions for the tabular setting. To be specific, firstly, our regret bounds depend on more precise numerical values of total rewards corruptions and transition corruptions, instead of only on the total number of corrupted episodes. Secondly, our regret bounds are the first of their kind in the reinforcement learning setting to have the number of corruptions show up additively with respect to min{root T , PolicyGapComplexity} rather than multiplicatively. Our results follow from a general algorithmic framework that combines corruption-robust policy elimination meta-algorithms, and plug-in reward-free exploration sub-algorithms. Replacing the meta-algorithm or sub-algorithm may extend the framework to address other corrupted settings with potentially more structure.
更多查看译文
关键词
episodic reinforcement learning,improved corruption robust algorithms,reinforcement learning
AI 理解论文
溯源树
样例
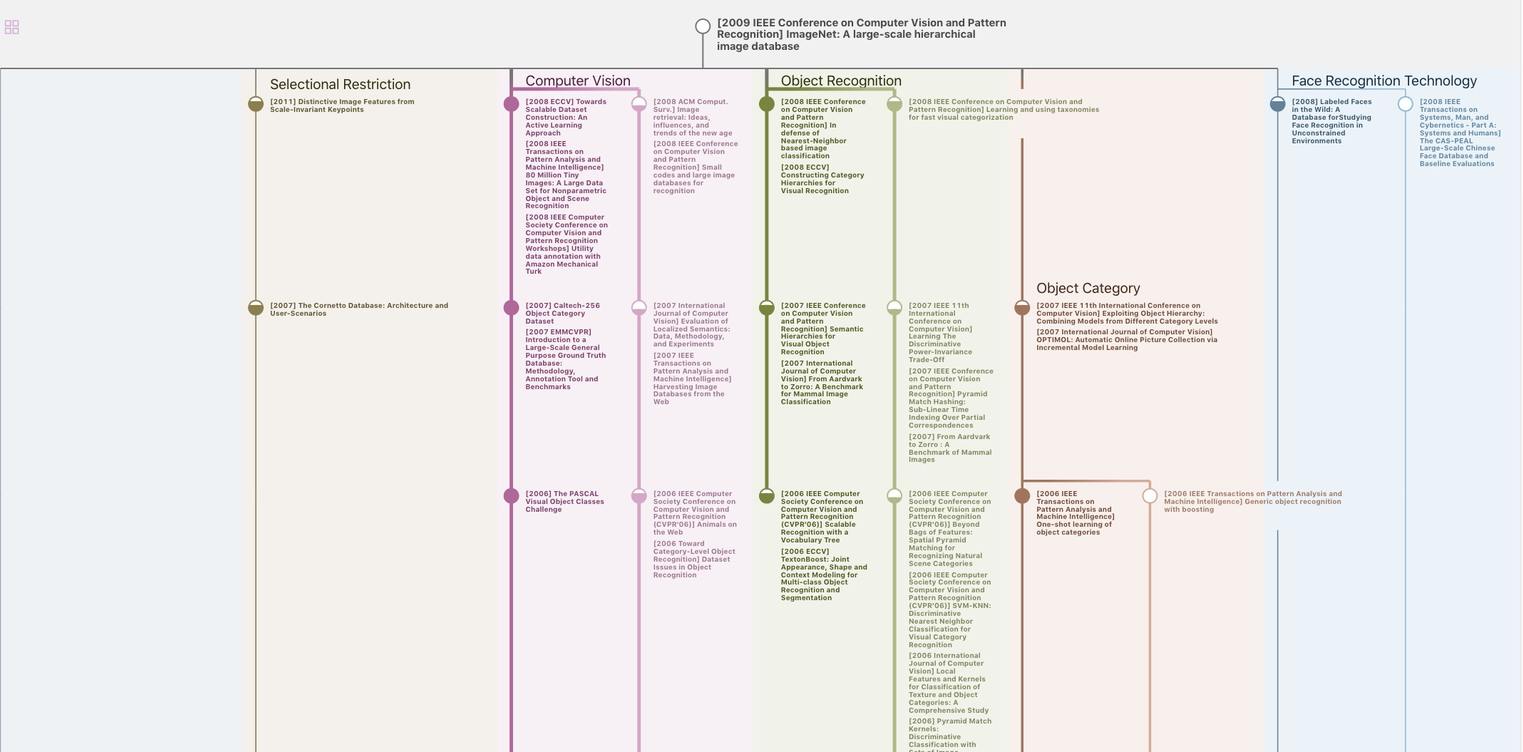
生成溯源树,研究论文发展脉络
Chat Paper
正在生成论文摘要