A Deep-Learning Approach for Direct Whole-Heart Mesh Reconstruction.
Medical image analysis(2021)
摘要
Automated construction of surface geometries of cardiac structures from volumetric medical images is important for a number of clinical applications. While deep-learning-based approaches have demonstrated promising reconstruction precision, these approaches have mostly focused on voxel-wise segmentation followed by surface reconstruction and post-processing techniques. However, such approaches suffer from a number of limitations including disconnected regions or incorrect surface topology due to erroneous segmentation and stair-case artifacts due to limited segmentation resolution. We propose a novel deep-learning-based approach that directly predicts whole heart surface meshes from volumetric CT and MR image data. Our approach leverages a graph convolutional neural network to predict deformation on mesh vertices from a pre-defined mesh template to reconstruct multiple anatomical structures in a 3D image volume. Our method demonstrated promising performance of generating whole heart reconstructions with as good or better accuracy than prior deep-learning-based methods on both CT and MR data. Furthermore, by deforming a template mesh, our method can generate whole heart geometries with better anatomical consistency and produce high-resolution geometries from lower resolution input image data. Our method was also able to produce temporally consistent surface mesh predictions for heart motion from CT or MR cine sequences, and therefore can potentially be applied for efficiently constructing 4D whole heart dynamics. Our code and pre-trained networks are available at https://github.com/fkong7/MeshDeformNet
更多查看译文
关键词
Whole heart segmentation,Surface mesh reconstruction,Graph convolutional networks,Deep learning
AI 理解论文
溯源树
样例
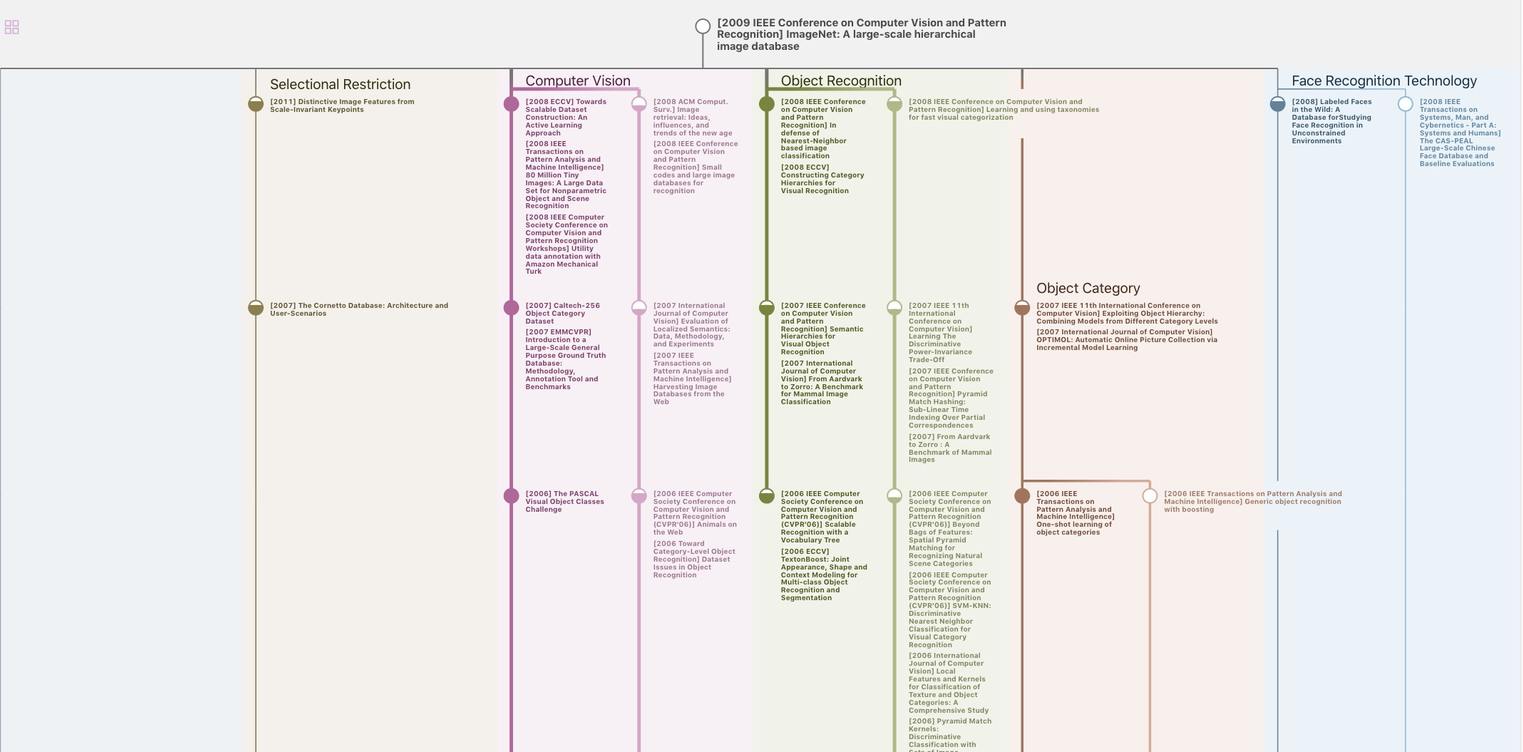
生成溯源树,研究论文发展脉络
Chat Paper
正在生成论文摘要