Inverse Reinforcement Learning in a Continuous State Space with Formal Guarantees.
Annual Conference on Neural Information Processing Systems(2021)
摘要
Inverse Reinforcement Learning (IRL) is the problem of finding a reward function which describes observed/known expert behavior. The IRL setting is remarkably useful for automated control, in situations where the reward function is difficult to specify manually or as a means to extract agent preference. In this work, we provide a new IRL algorithm for the continuous state space setting with unknown transition dynamics by modeling the system using a basis of orthonormal functions. Moreover, we provide a proof of correctness and formal guarantees on the sample and time complexity of our algorithm. Finally, we present synthetic experiments to corroborate our theoretical guarantees.
更多查看译文
关键词
reinforcement learning,continuous state space,formal guarantees,inverse
AI 理解论文
溯源树
样例
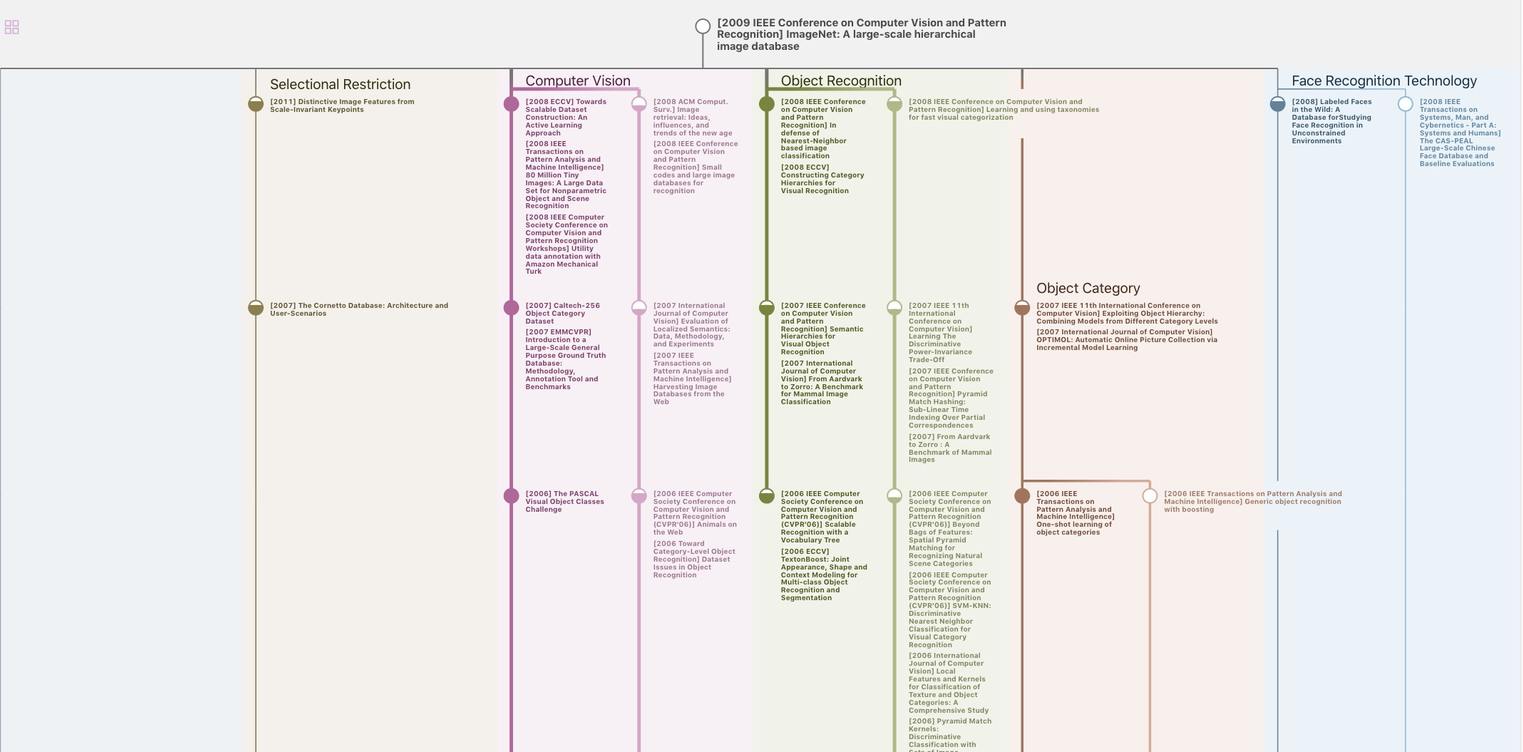
生成溯源树,研究论文发展脉络
Chat Paper
正在生成论文摘要