Non-Autoregressive Text Generation with Pre-trained Language Models
EACL(2021)
摘要
Non-autoregressive generation (NAG) has recently attracted great attention due to its fast inference speed. However, the generation quality of existing NAG models still lags behind their autoregressive counterparts. In this work, we show that BERT can be employed as the backbone of a NAG model to greatly improve performance. Additionally, we devise mechanisms to alleviate the two common problems of vanilla NAG models: the inflexibility of prefixed output length and the conditional independence of individual token predictions. Lastly, to further increase the speed advantage of the proposed model, we propose a new decoding strategy, ratio-first, for applications where the output lengths can be approximately estimated beforehand. For a comprehensive evaluation, we test the proposed model on three text generation tasks, including text summarization, sentence compression and machine translation. Experimental results show that our model significantly outperforms existing non-autoregressive baselines and achieves competitive performance with many strong autoregressive models. In addition, we also conduct extensive analysis experiments to reveal the effect of each proposed component.
更多查看译文
关键词
text,generation,language,non-autoregressive,pre-trained
AI 理解论文
溯源树
样例
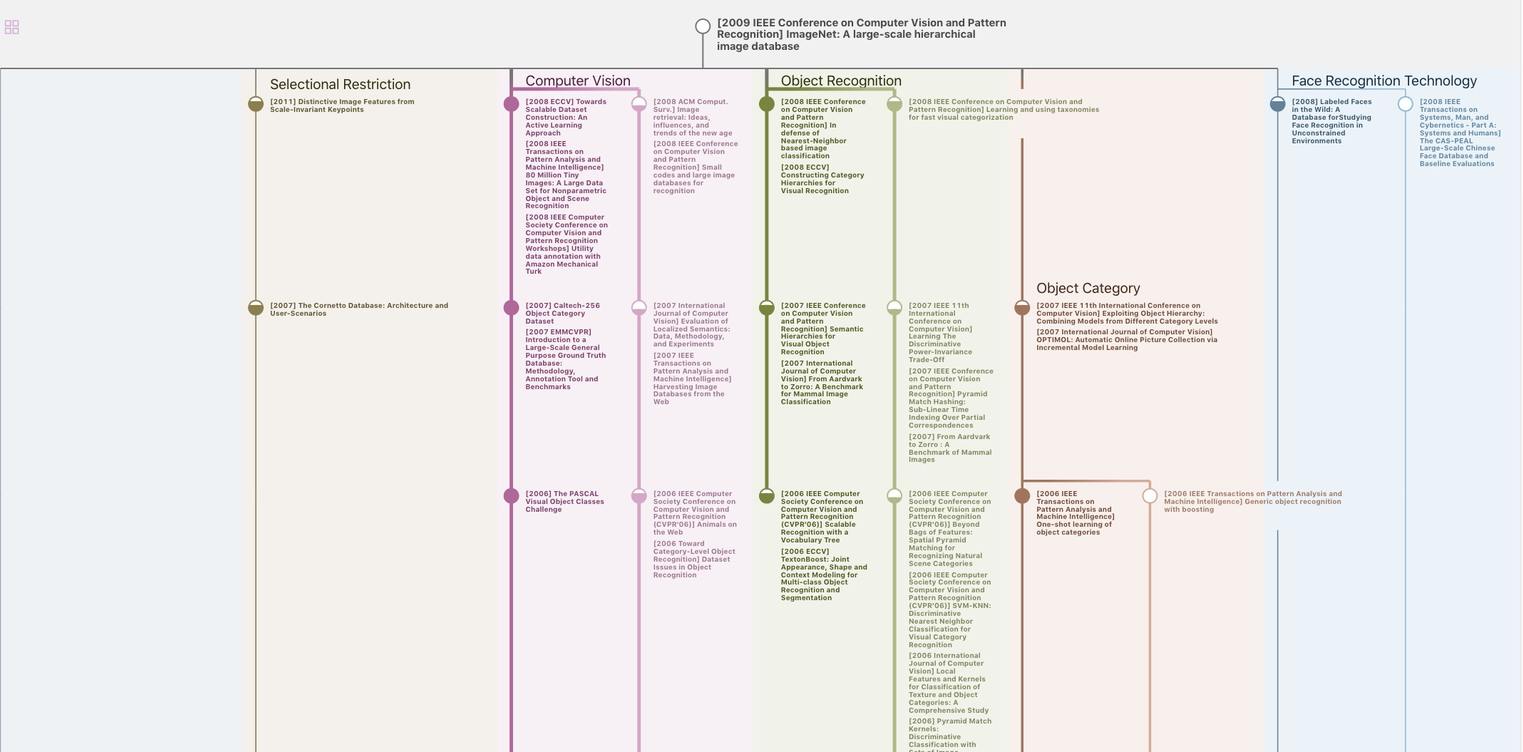
生成溯源树,研究论文发展脉络
Chat Paper
正在生成论文摘要