A discrete-time switching system analysis of q-learning
arxiv(2023)
摘要
This paper develops a novel control-theoretic framework to analyze the nonasymptotic convergence of Q-learning. We show that the dynamics of asynchronous Q-learning with a constant step size can be naturally formulated as a discrete-time stochastic affine switching system. In particular, for a given Q-function parameter, Q, the greedy policy, \pi Q(s) := arg maxaQ(s, a), in the Q-learning update plays the role of the switching policy, and is the key connection between the switching system and Q-learning. Then, the evolution of the Q-learning estimation error is over- and under-estimated by trajectories of two simpler dynamical systems. Based on these two systems, we derive a new finite-time error bound of asynchronous Q-learning when a constant step size is used. In addition, the new analysis sheds light on the overestimation phenomenon of Q-learning.
更多查看译文
关键词
Q-learning,switched linear system,stochastic approximation
AI 理解论文
溯源树
样例
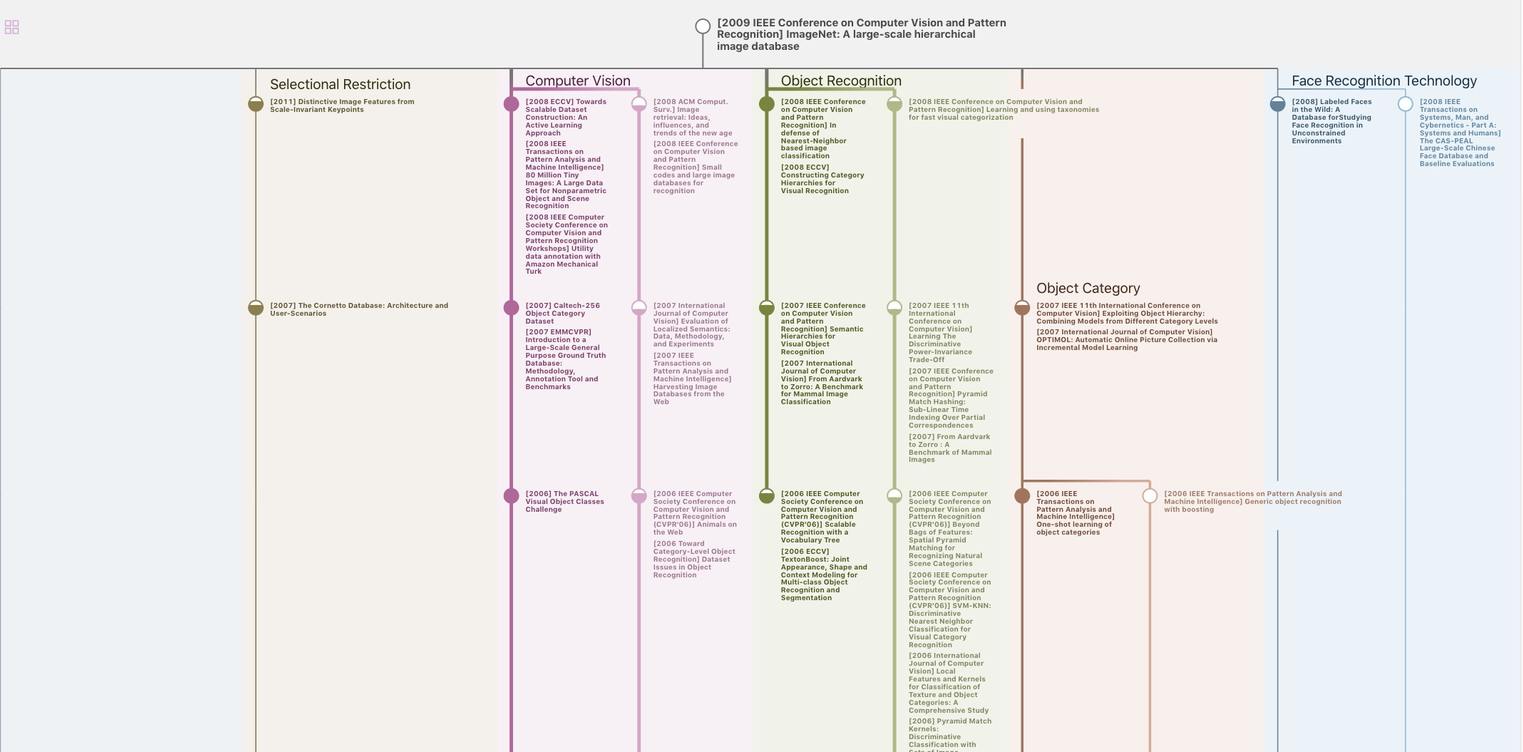
生成溯源树,研究论文发展脉络
Chat Paper
正在生成论文摘要