Towards Understanding and Inferring the Crowd - Guided Second Order Attention Networks and Re-identification for Multi-object Tracking.
IROS(2020)
摘要
Multi-human tracking in the crowded environment is a challenging problem due to occlusions, pose change, viewpoint variation and cluttered background. In this work, we propose a robust feature learning for tracking-by-detection methods based on second-order attention network that can capture higher-order relationships between salient features at the early stages of Convolutional Neural Network (CNN). Guided Second-Order Attention Network (GSAN) that, unlike the existing attention learning methods which are weakly-supervised, uses a supervisory signal based on the quality of the self-learned attention maps. More specifically, GSAN looks into the attended maps of a person having the highest confidence and supervise itself to look into the correct regions in the images of the person. Attention maps learned this way are spatially aligned and thus robust to camera-view changes and body pose variations. We verify the effectiveness of our approach by comparing with the state-of-the-art methods on challenging person re-identification and multi object tracking (MOT) datasets.
更多查看译文
关键词
multihuman tracking,viewpoint variation,tracking-by-detection methods,salient features,convolutional neural network,GSAN,self-learned attention maps,camera-view changes,multiobject tracking datasets,guided second order attention networks,attention learning methods
AI 理解论文
溯源树
样例
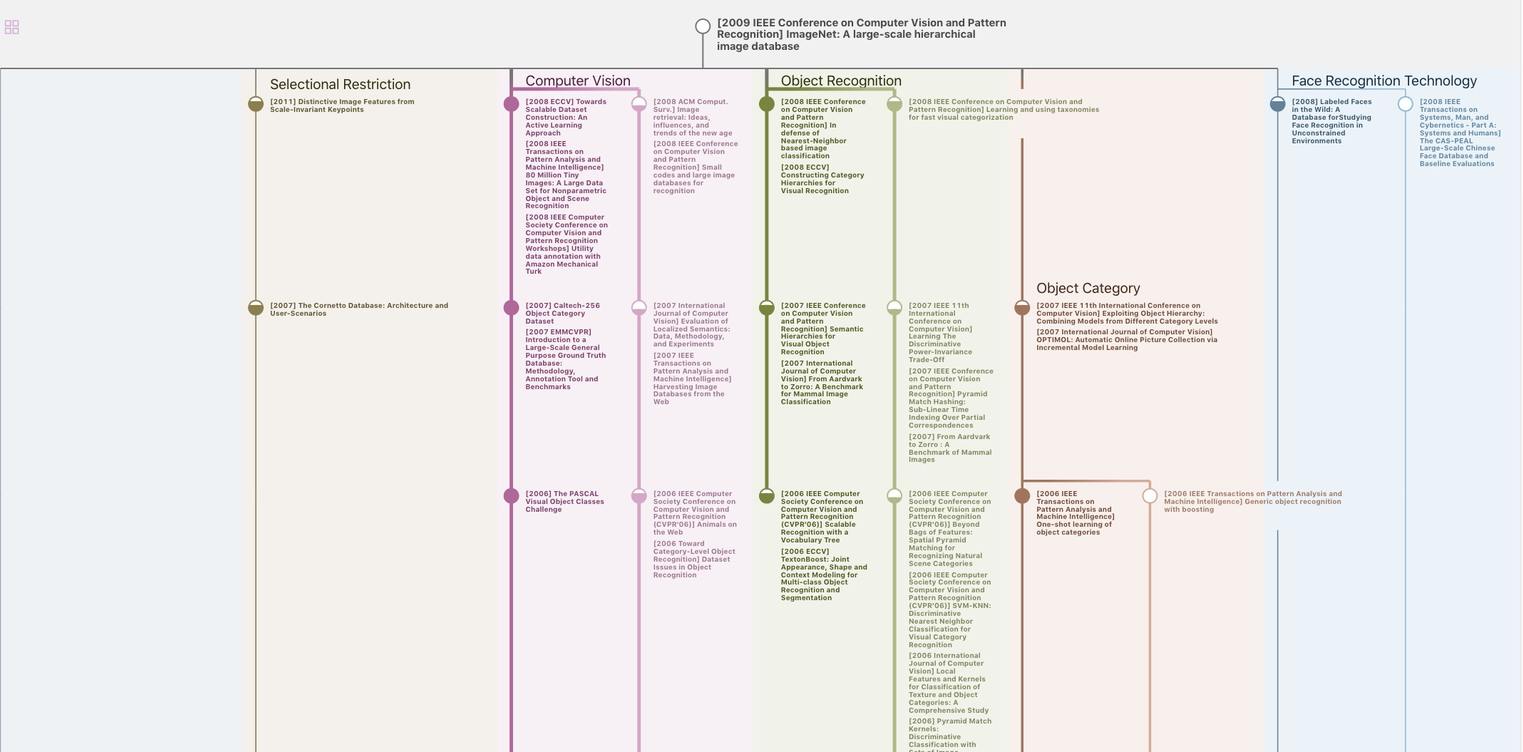
生成溯源树,研究论文发展脉络
Chat Paper
正在生成论文摘要